
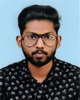
- Open Access
- Authors : Thoufil Ali M S , Divya Chandran
- Paper ID : IJERTV11IS070181
- Volume & Issue : Volume 11, Issue 07 (July 2022)
- Published (First Online): 30-07-2022
- ISSN (Online) : 2278-0181
- Publisher Name : IJERT
- License:
This work is licensed under a Creative Commons Attribution 4.0 International License
Impact of Land use Land Cover Change on Runoff in Kuttiyadi River Basin
Thoufil Ali M S 1, Divya Chandran2
1PG Scholar, Department of Civil Engineering, College of Engineering, Trivandrum,
2Assistant Professor, Department of Civil Engineering, College of Engineering, Trivandrum
AbstractLand use land cover significantly influence the hydrologic response of watershed. Changes in land use-land cover alter the hydrologic behaviour of watersheds. Quantifying the magnitude of these changes are essential for the long term planning and implementation of various water resource initiatives. This report presents the impact of land use land cover change on runoff in the Kuttiyadi River Basin. Using five LULC maps for the years 1988, 2002, 2012, 2020 and 2030, this study investigates the impact of land cover change on the study area. ERDAS 9.1 software was used to create maps from 1988 to 2020 using Landsat images. The Land Change Modeller of IDRISI Terrset was used to predict the LULC map for 2030.
The hydrological modelling was done using the Soil and Water Assessment Tool (SWAT) to run five different models. The fix- changing' method was used, in which climatic data from 1985 to 2013 was held constant and the sole variable was LULC maps. Monthly flow was calibrated in SWAT-CUP software for the years 19852003 and then validated for the years 20042013. Model 1, Model 2, Model 3, Model 4 and Model 5 calibration yielded a well-established model results with R2 values of 0.756, 0.778, 0.75, 0.71 and 0.762, respectively.
Results indicate that between 1988 and 2030, the spatial extent of Urban area, Plantation rose, whereas Forest, Paddy and Waterbodies decreased. Changes in LULC resulted in a 3.03% increase in surface runoff. The Curve Number increased as well, as it is closely related to land use change. From this study, it can be inferred that, land cover change is a significant element influencing a watershed's hydrological response, particularly surface runoff
-
INTRODUCTION
Land Use Land cover (LULC) and hydrology are intertwined, but, land use change has undeniably a significant impact on global water output. The quantity of evaporation, groundwater penetration and overland runoff that happens during and after precipitation events is directly influenced by land cover and land use. These variables influence the water yields of surface streams and groundwater aquifers, as well as the amount of water available for ecosystem function and human consumption. In specific sections of the watersheds, land use and land cover have an impact on runoff behaviour as well as the balance between evaporation, groundwater recharge and stream discharge (Sahin and Hall, 1996).
Humans are the main cause for altering the natural environment, especially through agriculture, deforestation, urbanization and changing land use/land cover, but the effects of these changes on the hydrologic cycle are not well understood. For optimal natural resource management, it is necessary to comprehend the effects of land use/land cover change on hydrologic conditions. Impacts of LU/LC changes on surface components of the hydrologic cycle have not been well understood thus far. To accurately assess the kind and direction of changes occurring within the catchment, change
detection analysis of multi-temporal remotely sensed data found to be more useful.
Understanding and exploring LULC change can be done efficiently using remote sensing and geographic information systems (GIS). For the investigation and simulation of LULC evolution, satellite imaging can provide adequate means of acquiring spatial and temporal information. Remote sensing is a valuable technique for providing detailed, accurate, consistent and cost-effective information. Future land use land cover forecasting is a recent study is extremely helpful in natural resource management. The scientific community is increasingly recognising LULC change detection and prediction, as an ideal approach to understand human interactions with earth systems. (Carpenter et al., 2001).
Primary input of many hydrologic models can be treated as Land cover maps. LULC change within a watershed has been identified as a crucial component influencing runoff generation and some researchers have claimed that LULC change has a bigger impact on runoff than climate change. A proper understanding of the hydrological processes that occur in the watershed is necessary for effective watershed management. At various locations, numerous studies on the impact of land use change on hydrological components such as runoff mechanism have been conducted. Numerous quantification approaches combined with the spatial data handling capabilities of Geographic Information Systems (GIS) can be used to handle data for hydrologic models.
To assess the hydrologic response of the study area under various land use, the Soil and Water Assessment Tool (SWAT) was chosen. GIS and remote sensing are used to prepare inputs to the SWAT model and can be used to predict and quantify the effects of land use change on a catchment's hydrology.
Since the study area Kuttiyadi river basin, has been subjected to extensive development, a large portion of the area is characterised by higher levels of built-up areas, deforestation and agricultural exploitation as a result of population growth. The catchment remained unaffected by human activities for some decades. However, it is now witnessing a large degree of LU/LC shift, which has a significant impact on the hydrological behaviour but is underappreciated. As a result, the need for a study is unquestionable in order to contribute precisely to the relationship between LU/LC and the area's hydrological state.
This study is being carried out to better understand and quantify the impact of LULC on hydrology, which will ultimately aid in the better use and management of natural resources, as well as contribute significantly to the solutions to the challenges mentioned above.
-
OBJECTIVES OF THE STUDY The study is mainly aimed;
-
To study the past changes in the LULC of the study area over the period 1988, 2002, 2012 and 2020 using ERDAS imagine 9.1
-
To predict the future scenario of LULC change of the river basin using TerrSet v.18
-
To assess the impact of LULC change on runoff using SWAT
-
STUDY AREA
The Kuttiyadi river basin is located in Kozhikode district of northern Kerala in India have longitudinal extension 75 35' 18" to 75 85' 53" East and latitudinal extension in 1 10 30'
19" to 1 10 44' 37" North (Fig3,l). The total basin area is 657km2 . The Kuttiyadi river originates from the Narikota Range of the western slopes of Wayanad Hills, which is a part of Western Ghats situated at an elevation of 1220 m M.S L flows west and joins the Arabian sea near Vadakara of Kozhikode districts. The Vadakara, Koyilandi and Kozhikode are the Taluks in which the river is flowing. The river is also known as the Murat River in Vadakara Taluk
Kuttiyadi river basin also contains two reservoirs namely Kuttiyadi reservoir and Kakkayam reservoir. Kakkayam reservoir is mainly used for hydroelectric power generation whereas Kuttiyadi reservoir serve the purpose irrigation. As per 2020 land use/ land cover analysis the basin is covered mainly with plantation and forest. The salient features of Kuttiyadi river basin is given in Table 3.
-
DATA COLLECTION
This section describes the various data required for the model and their source. Data for land use map preparation, SWAT modellin are included
-
Data required for land use map preparation
Four Landsat satellite images of year 1988, 2002, 2012 and 2020 were used in the study. One Thematic Mapper (TM), one Enhanced Thematic Mapper Plus (ETM+) and two Operational Land Imager OLI) were downloaded from USGS (United State Geological Survey). The satellite image with the acquisition dates and resolution is given in Table 3.2.
-
Data required for SWAT
-
-
METHODOLOGY
-
This chapter includes the systematic methodology adopted in the study to achieve the objectives. It also include the details of software and steps in modelling
-
Past Land Use Change Modelling
Landuse map of 1988, 2002, 2012 and 2020 were prepared using ERDAS Imagine v2014. To prepare LULC map of past decades landsat satellite images from USGS were used. To perform supervised classification the TIFF file of landsat images were layer stacked in ERDAS Imagine software. Any shift with respect to other georeferenced images had checked in ArcGIS and rectified in ERDAS Imagine. Supervised classification of landsat image had carried out according to the NRSC(National Remote Sensing Centre) Level-I classification having classes as follows:
Class-1: Agriculture Class-2: Barren land Class-3: Urban Class-4: Forest Class-5: Plantation Class-6: Scrubs
Class-7: Water bodies
-
Supervised Classification
The present study conducted Land Use Land Cover classification using Supervised classification method. Ancillary data used for this study includes False Colour Composite images. Easily recognizable features and locations in False Colour Composite (FCC) were selected for developing the spectral signatures. Locations that were selected, were given the generic name 'training sites and were digitized in the false composite images. Based on data from training sites and visual interpretation of the images, reclassification of each pixel was done by the Maximum Likelihood Classification Method (MAXLIKE). It is a tougher classification process, based on the assumption that the statistics for each class and in each band are normally distributed. This method calculates the probability of a pixel by the way that if a given pixel belongs to a specific class, then each pixel will be assigned to the class that has the highest probability, which is termed as the maximum likelihood classification method.
-
Accuracy Assessment
It is necessary to assess the correctness of the prepared LULC maps in order to determine how close the classified map is to reality or a ground reference. For this purpose, accuracy assessment is done. For all of the prepared images, Google Earth Image was used to generate a random set of points. For each class, about 20 points were chosen and compared to each pixel to ensure accuracy.
The Kappa coefficient is used to assess the correctness of a categorised image. The Kappa () index of agreement measures inter-rater agreement between a LULC map and ground control data that has already been taken from Google Earth Image. It has a value ranging from 0 to 1, with 1 indicating perfect agreement. A value greater than 0.80 (80%) shows strong agreement, a value between 0.40 and 0.80 (40 to 80%) suggests moderate agreement and a value below 0.40 (40%) indicates poor agreement. The flowchart of procedures involved in creating a land use land cover map is shown in Figure 4.1
-
Future Prediction Of Land Use Land Cover LULC change processes must be identified and projected as part of the LULC change study. Identifying the mechanisms of LULC change that entail human-environment interactions can help us better understand LULC transitions. A Business- As-Usual scenario (BAU) was used to predict LULC in this study. It is based on the premise that previous land-use
change tendencies would continue in the future, which is achieved by extrapolating past land-use changes (Ghaffari et al., 2010). In LULC change analysis, a comparison of current and past LULC trends, as well as modelling futuristic LULC patterns, will be beneficial. The land use patterns and possible future development can be better examined using a modelling technique. For LULC change analysis, a number of models are available. The most extensively utilised are the modelling methods provided within IDRISI. Land Change Modeller (LCM), CA Markov, GEOMOD and STCHOICE are some of the different modelling approaches accessible in IDRISI (Yonaba et al., 2021). However, LCM is the most extensively used modelling tool. In this study, the LCM in IDRISI Terrset was utilised to forecast future land usage. This methodology essentially assesses the pattern of land use change from one category to the next. Many contributing elements that affect the land change can be used to explain this. The final output will be a land use pattern prediction, which is mostly dependent on the prior change trend. In LCM, future forecasting of LULC is done in three steps: (1) change analysis, (2) transition potential and explanatory variable determination and (3) change prediction. (Hasan et al., 2020). The goal of LULC modelling in this study was to focus on the patterns of land change rather than only the sources of change.
-
Change Detection Analysis
Detecting the changes between two land use land cover maps of two distinct time periods is the main purpose of this panel. Changes are basically shown as graphical pattern of gain and losses, which helps to understand the dominant class wise transitions.
-
Transition Potential Modelling
The main goal of this tab is to create transition potential maps with acceptable degree of accuracy. It helps to group the transitions into set of subset models. Not only that, it also identifies the power of explanatory variables. The model allows the user to add either static or dynamic components. Static variables does not dependent on time. On the other hand, dynamic variables are time-dependent drivers i.e., they can be recalculated over time.
-
Selection of Explanatory variables
Factors that cause LULC changes are defined as Drivers or Agents or Explanatory Variables. These drivers are broadly categorized into biophysical (natural) and socioeconomic (anthropogenic) drivers. The biophysical drivers include weather and climate variables, soil depth, soil properties, elevation, slope, drainage patterns while socioeconomic drivers include demographic (population size, population distribution), economic growth, technological change, industrial structure, political and institutional factors (Pieri et al., 1995). Drivers selected for the present study are Topography, Slope, Distance from Built up and Distance from Vegetation. ArcGIS was used to create all of the drivers.
-
Multi-Layer Perceptron (MLP) neural network Multi-Layer Perceptron (MLP) neural net is one of the most commonly used Artificial Neuron Networks (ANN). It is based on the Backpropagation (BP) algorithm which is a supervised training algorithm. It consists of three layers, namely (1) input, (2) hidden, and (3) output. The advantage
of MLP is that during modelling, the multilayer perceptron allows more than one transition at a time. The data in MLP initially flows through the hidden layer in one direction, from an input layer to an output layer and determines non-linear relationships. Nodes are assembled within the layers and every node receives an input signal from different nodes and produce a transformed signal to other nodes. Each original input layer will be assigned a weight and it passes through either a linear or non-linear stimulation function. The multilayer perceptron will be trained with various influencing factors for each of the sub-models and it produces time- explicit transition potential maps that represent time-explicit change potential
-
Change Prediction
Change prediction is the final phase in the future prediction process and it is performed using a Markov chain with historical rate of chane and transition potential maps
-
Markov Chain Modelling
The Markov chain model is a stochastic modelling approach that predicts future land use and land cover from time t = 1 to time t + 1 using the transition probability matrix of each land use and land cover class. The transition matrix depicts the likelihood of a change in land use or land cover from one land use group to another throughout the reported time period (Han et al., 2015).
A Markov Chain can be thought of as a random process in which the following step is largely determined by the present state. By analysing two LULC images from two separate dates, Markov generates transition matrices and a set of conditional probability images.
-
Future Scenario
In LCM, predictions are divided into two categories: 1) hard predictions and 2) soft predictions. A hard prediction generates a projected map based on a Multi-Objective Land Allocation (MOLA) module (Megahed et al., 2015), in which each pixel is assigned to one of the land cover classes based on their most likely probability. Soft prediction calculates the likelihood of a pixel changing to another land category by creating a vulnerability map and by assigning a value ranging from 0 to 1 for each pixel (Megahed et al., 2015). Figure 4.2 shows the flowchart of methodology involved in Land Change Modeller (LCM)
-
Hydrological Modelling
Models are useful for understanding hydrologic processes, developing management methods, and assessing the risks and benefits of land use across time. Many models have been created to simulate watershed runoff as well as anticipate the influence of watershed management strategies or land use changes on runoff. There are two types of hydrologic models: lumped and spatially distributed. (Spruill et al., 2000) Lumped models are the ones that simulate a spatially averaged hydrologic system without accounting for spatial variation within the modelling domain. Precipitation, temperature and other environmental variables can all fluctuate regionally in spatially distributed models. It also takes into account the distribution of watershed characteristics including soils, slope and land cover types. As a result, spatially distributed models are preferable to lumped models for analysing the effects of land use/land cover changes.(Chow et al., 1988)
SWAT model was chosen and employed in the current study for hydrological modelling based on data availability and capability of accounting LULC, soil, climate data and hydrology.
-
SWAT Model
The USDA's daily-use Soil and Water Assessment Tool (SWAT) is a long-term, physically based conceptual model designed using an Arcview GIS interface. The SWAT model is primarily used to predict the impact of land management practises on water, sediment and chemical yields over long time periods across broad basins with a wide range of soils, land use, and management. Surface runoff, evapotranspiration (ET), percolation, infiltration, aquifer flow (shallow and deep), and channel routing are among the hydrological processes considered in the model. Hydrologic processes are computed in five phases: (i) precipitation and interception, (ii) surface runoff, (iii) soil and root zone infiltration, (iv) evapotranspiration, soil and snow evaporation, and (v) groundwater movement. (Neitsch et al. 2001)
The SWAT model separates the catchment into sub-basins or sub-watersheds based on topographic factors, then further divides it into a number of HRUs (Hydrological Response Units) based on unique combination of soil, slope and land use. Simulations for components of hydrological cycles, nutrient cycles and sediment yield can be run separately and then aggregated for sub-basins. Figure 4.3 shows the methods used in SWAT modelling.
The fundamental hydrological used in SWAT is based on the water balance eq. (4.1) which is based on mass balance and it calculates the change in soil water content (SWt).
can also alter the retention parameter. The following equation (4.3) defines the retention parameter:
(4.3)
Where, CN is the Curve Number (100 CN 0). The soil hydrologic group, soil permeability, and land use all influence CN. The model employs the NRCS categorization system, which divides soils into four hydrologic groups (A, B, C, and D) based on their infiltration properties. The hydrologic soil groups and their attributes are listed in Table 4.1
Where,
SWt= Final soil water content in mm,
(4.1)
SW0= Initial soil water content minus the permanent wilting point water content (mm),
Rday= Amount of precipitation on day i (mm), Qsurf= Amount of surface runoff on day i (mm), Ea= Amount of evapo-transpiration on day i (mm),
Wseep= Amount of water entering the vadose zone from soil profile on day i (mm),
Qgw= Amount of return flow on day i (mm), t = Time in days
-
SWAT Runoff estimation
Surface runoff occurs when the rate of water application to the ground surface exceeds the rate of water infiltration (Neitsch et al., 2001). The SCS curve number process (SCS, 1972) and the Green and Ampt infiltration method are both accessible in SWAT for predicting surface runoff
.Due to the lack of sub-daily data for the Green & Ampt approach, the current study used the SCS Curve Number method to estimate surface run off, which is mainly influenced by permeability of the soil, land use and antecedent moisture. The equation (4.2) shows the runoff computation using SCS Curve Number (SCS, 1972):
(4.2)
Where,
Qsurf = Accumulated runoff or rainfall excess (mm), Rday = Rainfall depth for the day (mm),
Ia = An initial abstraction which includes surface storage, interception and infiltration prior to runoff (mm),
S = Retention parameter (mm). Because of differences in soils, slope and land use management, the retention parameter varies spatially. Changes in the amount of water in the soil
-
SWAT MODEL INPUT
-
Digital Elevation Model (DEM)
A digital elevation model is a geographic grid of an area in which the contents of each grid cell describes the elevation of any point at a particular location with a specific spatial resolution in the form of a digital file (Ghosh, 2016). It's a critical spatial input for determining the boundaries of watersheds. The DEM utilised in this study was downloaded from the BHUVAN (Carstosat). A DEM with a resolution of 30 metres was created and clipped to the study region. The DEM for the Kuttiyadi river basin was used to determine various sub-basin parameters such as slope, length of terrain, slope gradient and stream network features such as channel length and width.
-
Land Use Data
The LULC map is another key model input for SWAT modelling. The modellers can use this data to investigate the effects of LULC modification by changing its spatial and temporal distribution. ERDAS 9.1 Software was used to
create the land use map of the Kuttiyadi river basin, as stated in Section 4.1.1 of this Chapter.
-
Soil Data
Soil data for SWAT modelling should include important inputs on the physical and chemical parameters of the catchment's soil. Soil data for this study was gathered from the Food and Agriculture Organization of the United Nations' Global Soil Map, which has a geographical resolution of 10 km. Using the raster projection in ArcMap, the FAO map was projected to WGS1984 UTM Zone43N. Finally, it was clipped with the study area's shape file in ArcGIS, which provided the necessary data for modelling the watershed
-
Weather Data
Following the reclassification of land use/cover and soil data, weather data can now be submitted into the SWAT interface. For the period 1985 to 2013, weather data such as precipitation (mm), temperature (° C), wind speed (m/s), solar radiation (MJ/m2), and relative humidity (given as a fraction) were acquired from SWAT Global Weather Data. This information is critical for determining the water baance components in the watershed.
-
-
SWAT MODEL SET UP
The entire process of simulation basically involves four steps, namely, watershed delineator, HRU analysis, writing input tables and lastly SWAT simulation. All the steps are described below.
-
Watershed Delineation
Watershed delineation implies creation of a boundary that depicts a contributing area for a particular outlet or control point. In the process of delineation, watershed is divided into sub basins. For this purpose, ArcSWAT uses DEM, based on which stream definition was done followed by flow direction and accumulation due to which stream network and outlets were created. When the delineation is complete, it creates a detailed topographic report which describes the statistical summary and distribution of discrete land surface elevation within the watershed along with all the sub-basins
-
HRU Definition
A Hydrological Response Unit (HRU) is defined as a unique combination of various land use, land cover, soil and slope classes (Ghosh M, 2016). When the watershed is subdivided into areas having unique land use and soil combinations, it enables the model to reflect differences in evapotranspiration and other hydrologic conditions for different land use and soils. The prediction of runoff is done separately for each HRU and then it is routed to obtain the total runoff for the watershed. This helps to increases the accuracy and provides much better description of water balance. The distribution of hydrologic response units (HRU) within the watershed can be determined, only when the land use and soil data layers have been imported. This process is performed for every sub-basin. In the HRU definition process, a land use and soil distribution will be obtained. This will provide the detailed description of land use and soil classes after the application of HRU overlay for the basin and sub-basin (Ghosh M, 2016)
-
Weather Definition
After the HRU distribution is completed, the next step is weather data input. The weather station locations and weather data is introduced into the sub-basins. Weather data should be stored in a specific tabular and supportive file format of Arc SWAT. Considering that aspect, they were stored in DBF format which can be read by ArcSWAT interface.
-
SWAT Simulation
After inputting LULC, soil and weather data as per SWAT input format, SWAT simulation can be carried out. Simulation period need to be specified (1985-2013). SWAT simulation provides an option to choose the warm up period (NYSKIP Number of Years to Skip) to ensure that there are no effects from the initial conditions in the model. The length of warm up period differs from watershed to watershed usually ranges from 3 to 5 years. For this period, the simulated results are not printed. SWAT computes the water balance components such as runoff, lateral flow, PET, ET and sediment yield. Runoff is calculated based on equation (4.2).
-
-
SENSITIVITY ANALYSIS
Sensitivity analysis evaluates the influence of different parameters on simulation result, i.e. the response of output variable to a change in input parameter. The most difficult process is to determine which parameters to calibrate such that it reflects the field parameters as closely as possible. In such situations, sensitivity analysis helps to identify and rank the parameters which have noteworthy effect on specific model outputs of interest (Saltelli et al., 2000). The parameter which produce greater change in the output response is considered as most sensitive parameter. Initially 15 parameters were considered, after an initial iteration run of model, the most sensitive parameters were identified and only those parameters were adjusted, in order to improve the calibration efficiency.
-
MODEL CALIBRATION AND VALIDATION
The model calibration procedure is developed based on optimization techniques (Guo et al., 2008) with the assumption that an optimal set of parameters exists in the model to describe the hydrology of the river basin. The process of adjusting the model parameters is defined as Calibration. It has two sub-processes namely parameter identification and parameter estimation. Through the process of parameter identification, one can define and choose the most sensitive parameters of the model. Meanwhile, parameter estimation consists of fixing the values of the chosen parameters. Parameter identification is done through Sensitivity Analysis. Both the sub-process helps to limit the number of optimized parameters required to obtain a good fit between the simulated and observed data. Hence, this reduces the number of parameters to be adjusted considerably. In this study, sensitivity analysis of the model, calibration and validation has been done using the SWAT-CUP (SWAT Calibration and Uncertainty Program) tool.
-
SWAT CUP TOOL
SWAT- CUP was developed by Eawag Swiss Federal Institute, to find out the uncertainty of SWAT model calibration and validation results. Currently, the program is able to run algorithms such as SUFI2 (Sequential Uncertainty Fitting ver.2), GLUE (Generalized Likelihood Uncertainty Estimation), MCMC (Markov Chain Monte Carlo) and ParaSol (Parameter Solution). In this study, SUFI2 algorithm has been used as the calibration algorithm, because of its wide popularity as a calibration tool. Not only that, it is capable of producing good calibration and uncertainty results.
-
Sequential Uncertainty Fitting
The SUFI-2 approach employs a Bayesian framework. This method uses sequential and finite procedures to determine uncertainty. This model takes into account uncertainties in the model input, model structure, model parameters and observable data from all conceivable sources. The SUFI2 approach involves developing an initial or default input parameter, then creating the necessary input files for SWAT CUP. The model must be run with the input files and default settings already configured. At this point, the most sensitive parameter can be identified. Then, for each parameter, assign an initial uncertain range (usually 20% to 30%). Run the SWAT-CUP-SUFI2 model after assigning the parameter range, then execute the global sensitivity analysis and evaluate the results. The p-value and t-statistic are utilised at this point to rule out non-sensitive parameters from the calibration procedure. Then, regionalize the parameters after watching the model's performance. The model performance was evaluated using Nash Sutcliffe Model Efficiency Coefficient (NSE) which is calculated based on
-
RESULTS AND DISCUSSSIONS
-
Land Use Land Cover Change
Land use Land Cover classification of the study area was carried out for the years 1988, 2002, 2012 and 2020, supervised classification was used to classify the land cover of the study region. Plantation, Forest, Urban, Barren Land, Paddy field, Scrubs and Waterbody are the seven land use classes that have been recognised during classification. Figures 5.1 depicts the results obtained after post- classification of Landsat images.
In 1988 plantation and forest covers 45.93% and 36.72% of total area respectively. Following years shows a decreasing pattern for forest area and an increasing pattern for plantation area. From 1988 to 2020 forest area decreased by 6.33% whereas there is 5.01% increase in plantation area. Urban area covers 2.64% of total area in year 1988, which increased to 2.95% in 2002 and 4.51% in 2012 which further increased to 4.82% in 2020. That is urban area shows an increasing trend. Paddy field area decreased from 5.46% to 2.89% from year 1988 to 2020. There is no significant change for waterbodies.
equation (4.4)
Where
(4.4)
qo and qs are the observed and simulated flow respectively (m3 /s);
qo.i – observed value on day i (m3 /s);
qs – average value of the observed flow (m3 /s); i – Number of variables;
n – Total number of values within the period of analysis.
NSE ranges fro -1 to 1, with 1 being a perfect match between the expected and observed models. A value of zero, on the other hand, shows that the expected and actual values are not identical. If the NSE value is negative, the predictions are likely to be inaccurate. All fitted parameters should be updated back to the ArcSWAT model after finishing the calibration, sensitivity analysis and validation. The model should then be run again with the updated parameters.
Fig:5.1 Land use/ land cover map of a) 1988, b) 2002, c) 2012 and d) 2020
Table.5.2 illustrates producers, user and overall accuracy and kappa statistics of the various landuse classification for different period which is calculated by selecting random points in classified map and comparing with google earth image. The overall accuracy is 88.16%, 87.76%, 90.61% and
90.20% and kappa statistics is 0.86, 0.86, 0.89 and 0.89 for
1988, 2002, 2012 and 2020 respectively. The past changes in LULC is taken as base for the projection of LULC maps future.
-
FUTURE PREDICTION OF LULC
After creating required LULC maps, future prediction was carried out in LCM of IDRISI Terrset for the year 2030. Prediction map was created by using the prepared LULC maps of year 2002 and 2012. Driver variables used for projections are elevation, slope, proximity to urban, proximity to forest and proximity to water bodies.
Land Change Modeller was calibrated using LULC maps of year 2002 and 2012. After finishing 500 iterations by MLP, accuracy rate attained was 82.71 %, with an overall skill measure of 0.793 across all transitions being modelled. Root Mean Square Error (RMSE) achieved in the training and testing stage were respectively of 0.2087 and 0.2204. Finally, after conducting Markov chain modelling, the prepared model first predicted the map of 2020. For this transition matrix of 2020 (Table.5.3) were produced using 2002 and 2012 LULC and driver variables. Main transitions where transition from paddy to barren, urban and plantation, barren to urban and forest to plantation.Inorder to check the accuracy of the predicted map, it was validated with the actual LULC map of 2020 prepared from Landsat images. Area statistics of of LULC classes obtained is shown in Table
5.4 and Figure 5.3.
Fig.5.3 Actual and projected 2020 LULC
Visual examination and area statistics of the simulated map indicates that MLP model has better predicted all the LULC classes of year 2020. On the predicted map, areal extent of all LULC matched approximately with the actual map. This indicates that the model can be used for the future prediction of LULC with better accuracy rate. As the model shows accepted accuracy rate, it was used for the prediction of LULC map of 2030. Predicted map of 2030 and its transition probability matrix is shown in Fig 5.5 and Table 5.5.
From the predicted map of 2030, the area bound by Plantation will be 346.4 km2. 186.4 km2 will be covered by Forest, 33.53 km2 by urban, 11.01 km2 by Barren Land. Paddy feild and Waterbody will have an areal extent of 17.89 km2 and 16.46 km2 respectively.
Plantation is the dominating LULC type, comprising majority of the study area, based on area statistics figures from 1988 to 2030 . According to the findings, plantation had a rate of area coverage of 45.93 percent in 1988, which was significantly higher than other LULC classes. However, by the end of 2020, an increase of 5.415 percent in plantation had been detected. From 2020 to 2030, the predicted results show an increasing trend of 1.522 percent. Throughout the study period, there was a increasing trend in plantation
The urban is on the rise, that has increased from 2.64 percent to 5.08 percent. During the study period, forest cover decreased from 36.72 percent to 28.23 percent. Paddy field aerial expanse has shrunken over the course of the study. Between 1988 and 2012, there was a 2.75 percent reduction in area. From 2012 to 2020, it gets reduced to 0.25 percent. The future result reveals a 0.104 percent decline in paddyfield area. Decrease in forest and paddy field is reflected in increase of plantation and urban area. The area under Barren Land changed in a different way. Barren Land's aerial extent decreases marginally from 1988 to 2030, with a
1.1 percent decrease.
-
SWAT HYDROLOGICAL MODELLING
The first step in SWAT modelling is watershed delineation. The steps involved in watershed delineation is explained in Chapter 4, Section 4.5. Watershed delineation was carried out using BHUVAN DEM of spatial resolution 30m x 30m. Figure 5.6 shows the delineated watershed.
Next step after watershed delineation is HRU definition.
HRUs in SWAT is defined on the basis of LULC Map, Soil Map and Slope Map. In this study, all the prepared LULC maps (Fig 5.1) are used for SWAT model preparation. Next input data after LULC is the Soil map. Preparation of Soil map is described in Section 4.5. Figure 5.7 shows the soil map of the study area.
The weather data must then be defined in the SWAT model. Climate data from 1985 to 2013 was used in this modelling. Firstly, the SWAT model was calibrated and validated for the selected periods in order to mimic the influence of LULC change across the decades. The fix-changing or delta method (Ghaffari et al., 2010) was used for this. All changes in hydrological variables were presumed to be the result of various land use inputs in this approach. Only the LULC maps were changed, while other variables like climate, soil, and topography remained constant.
For performing calibration and validation, three models were simulated. Table 5.8 shows the details of Models. After conducting successful calibration and validation, five scenarios were created by updating the calibrated parameters back to the simulated models.
The climate data from 1985 to 2013 was kept constant. The simulation period was from 1985 2013 with a warm up period of three years (1985 1987). Calibration was performed in SWAT CUP software using SUFI2 algorithm. Initially, the sensitive parameters were identified using Global sensitivity analysis. Parameters with greater absolute t-stat value and lower p-value is considered as most sensitive parameter.
Out of 15 parameters considered, 6 parameters were most sensitive. For further calibration, only these parameters were adjusted. To increase the model efficiency, several iterations were done each having 150 simulations. All the models were calibrated for the same time period (1985 2013) in a monthly time step and Table 5.9 shows the parameters selected for calibration.
In Table 5.10, where r_ r epresents the relative change in parameter and 'v_represents replace with existing parameter. The initial and final ranges and the fitted values of parameters after the calibration was done are depicted in Table 5.11.
For model validation, the remaining observed flow data (2004 -2013) were used. In the validation process, the model was run with input parameters set during the calibration process without any change. Validation period shows a good agreement between monthly measured and simulated flows. Results of goodness of fit of calibrated data for all the models have been presented in Table 5.12
Comparison between Observed and Simulated flow for each model has been presented in Fig 5.6, 5.7, 5.8, 5.9, 5.10.
Figure 5.8, 5.9, 5.10, 5.11 and 5.12 shows the best fit between observed and simulated values of Flow for model 1, 2, 3, 4 and 5. Finally, the calibrated values of selected parameters were given as new input to the five models. Models were simulated again with the new calibrated parameters. Five Scenarios were developed to assess the changes in LULC alone.
-
Impact of LULC Change on surface runoff
The surface runoff has changed due to the effect of LULC change during the study period, according to simulations of the five SWAT scenarios. The surface runoff increased from the first scenario (S1) to the fifth scenario (S5) for an average precipitation of 2574.7mm. The mot sensitive parameter in estimating the fraction of precipitation converted to surface runoff is the Curve Number (CN2). Changes in land use have been linked to changes in Curve No. (CN) values. The average CN for antecedent moisture condition-II (CN2) for all the scenarios is shown in Table 5.13, which likewise indicates an increasing trend. An increase in CN levels resulted in more surface runoff.
From Table 5.13, it is evident that, initially, surface runoff was lower in Scenario 1 and it gradually increases in Scenario 2 ,3,4 and 5. Around 35.21 mm (3.03%) of surface runoff increased from Scenario1 to Scenario 5. Average annual surface runoff increased by 0.82% for the LULC change from 1988 to 2002. An increase of 1.76% was seen from LULC of 1988 to 2012. Increase in surface runoff witnessed from 1988 to 2020 is about 2.16%. Prediction of runoff from 1988 to 2030 shows an increase of 3.03%. This rise in run off can be linked to the increase in Curve Number, which is a function of land use and practise.
Curve Number have a direct relationship with runoff variation. Because SWAT estimates Surface Runoff using the SCS Curve Number method, CN is critical in hydrological modelling. Initially, the Curve Number was 83.22, but by the fifth scenario (S5), the Curve Number had risen to 87.51 due to changes in the land cover of the study area.
-
Runoff at sub watershed level
Figure 5.6 shows the sub watersheds of the study area. Total 12 sub watersheds were found. Effect of sub-basin level land- use change on the run off was studied using simulations based on the 1988 and 2030 land-use data(Scenario-1 and Scenario-5). From Figure 5.14, it is evident that surface runoff has increased in all the 12 sub-watershed (SW) with changes varying from 0.803% to 10.562%. Variability of surface runoff changes among sub-basins suggests variations of changes in each LULC class at sub-basin level. Sub basin 3 (Perambra) shows the lowest change in surface runoff of about 0.803%. Highest change in runoff was observed in Sub basin 12 (Pathiyarakara) which is 10.562%. Sub basin 2,4,6,9,10 and 11 also experienced significant changes in run off. Sub-basin level analysis revealed that more distinct runoff increase was observed mostly in the downstream end compared to upstream end where there is forest. Since there were several LULC types in each sub-basin with varying degrees of change, the change in runoff can be attributed to the resultant impact of decrease in forest and increase in urban area and plantation.
-
-
CONCLUSION
-
The study's main goal was to look at the impact of LULC changes on surface runoff in the Kuttiyadi River Basin. From 1988 through 2030, all land cover classes were shown to have undergone significant alterations. Past land use changes indicate that forest and paddy has been declined and plantation area increased. The study region has lost a significant amount of forest land, amounting to 8.49%. The area of Urban land, on the other hand, increased by 2.44% throughout the study period. Waterbody and Paddy field have both decreased by roughly 0.26% and 2.75%, respectively. Plantation showed a rapid increase of about 6.53%. As a result, it can be concluded that all land cover classes have gone through significant changes.
The results from this study showed that the SWAT model can successfully simulate the hydrological modelling in the Kuttiyadi River Basin, as evidenced by strong R2 and NSE values during the calibration and validation periods. From 1988 to 2012, average annual surface runoff increased by
1.76 % and from 1988 to 2020 and 1988 to 2030, it would grow by 2.16% and 3.03%, respectively.
Loss in forest cover resulted in an increase in runoff. Plantation expansion and urbanisation have a favourable impact on surface runoff, while forest, and paddy field destruction have a negative impact. The findings lead to the conclusion that land cover changes had a significant impact on surface runoff in the past and will continue to do so in the future.
The current study found that the impact of land use change at the sub-basin level was more pronounced, with considerable runoff variation. The analysis concludes that the increase in surface runoff is mostly due to increased urbanisation, plantation and decreased forest cover, both of which alter the river hydrological behaviour. The methods utilised in this study can be used to anticipate hydrological repercussions of land cover changes in a range of watersheds where time- sequenced digital land cover data is available
REFERENCES
[1] R. Yonaba., M. Koïta., L.A. Mounirou., F. Tazen., P. Queloz., A.C. Biaou ., D.Niang and H. Yacouba., (2021), Spatial and transient modelling of land use/land cover (LULC) dynamics in a Sahelian landscape under semi-arid climate in northern Burkina Faso, Land Use Policy 103 (2021) 105305 [2] Sarah Hasan., Wenzhong Shi., Xiaolin Zhu., Sawaid Abbas and Hafiz Usman Ahmed Khan, (2020), Future Simulation of Land Use Changes in Rapidly Urbanizing South China Based on Land Change Modeller and Remote Sensing Data, Sustainability 2020, 12, 4350 [3] Ramesh H and Venkatesh K., (2018), Impact of land use land cover change on run off generation in Tungabhadra river basin, ISPRS Annals of the Photogrammetry, Remote Sensing and Spatial Information Sciences, Volume IV-5, 2018 [4] Han,H.; Yang, C.; Song, J. Scenario Simulation and the Prediction of Land Use and Land Cover Change in Beijing, China. Sustainability 2015, 7, 42604279 [5] Richards, J.A., 2013. Remote Sensing Digital Image Analysis.Springer, Berlin, Heidelberg, Fifth Edition, 2013, pp. 247 -318.
[6] Abbaspour, K.C., (2008), Application of a SWAT model for estimating runoff and sediment in two mountainous basins in central Iran, Journal of Hydrology, 53(5) October 2008 Special issue: Advances in Ecohydrological Modelling with SWAT. [7] Ghaffari G, Keesstra S, Ghodousi J and Ahmadi H, 2010, SWAT- simulated hydrological impact of land-use change in the Zanjanrood Basin, Northwest Iran. Hydrol. Process. 24, 892 903 (2010) [8] Ghosh M., 2016, Application of SWAT model to assess the impact of land use changes on daily and monthly streamflow of Subarnarekha River Basin, National Institute of Technology Rourkela, 2016 [9] Guo, H., Hu, Q. and Jiang, T. 2008, Annual and seasonal stream flow responses to climate and land-cover changes in the Poyang Lake basin, China. Journal of Hydrology, 355(14), 106122. [10] Mulungu, D. M. and Munishi, S. E. (2007). Simiyu River catchment parameterization using SWAT model. Physics and Chemistry of the Earth, Parts A/B/C, 32(15), 1032-1039. [11] Neitsch (2001) Soil and Water Assessment Tool, Theoretical Documentation: Version (2001). Temple, TX. USDA Agricultural Research Service and Texas A&M Blackland Research Center. [12] Sinha R.K. and Eldho T. (2017). Effects of historical and projected land use/cover change on runoff and sediment yield in the Netravati river basin, Western Ghats, India International Soil and Water Conservation Research, Science Direct 2017. [13] Spruill, C. A., Workman, S. R., & Taraba, J. L. (2000). Simulation of daily stream discharge from small watersheds using the SWAT model Transactions of the ASAE. American Society of Agricultural Engineers, (43), 1431. [14] Wagner, P.D., Kumar, S. and Schneider, K., 2013, An assessment of land use change impacts on the water resources of the Mula and Mutha Rivers catchment upstream of Pune, India., Hydrology and Earth System Sciences, 17(6), pp.2233-2246. [15] Sahin, V. & M.J. Hall, (1996), The effects of afforestation and deforestation on water yields, Journal of Hydrology, Volume 178,Issues 14, 15 April 1996, Pages 293-309
[16] Carpenter, Gopal, S., Shock, B. and Woodcock, C., 2001, A neural network method for land use change classification, with application to the Nile River Delta. Beacon Street, Boston University, 1-8pp. [17] Pieri,C., Dumanski, J., Hamblin, A. and Young, A., 1995. Land Quality Indicators (World Bank Discussion Paper No. 315). The World Bank, Washington DC, USA. [18] Megahed, Y.; Cabral, P.; Silva, J.; Caetano, M., (2015). Land Cover Mapping Analysis and Urban Growth Modelling Using Remote Sensing Techniques in Greater Cairo Region Egypt. ISPRS Int. J. Geo-Information 2015, 4, 17501769. [19] Chow V.T, Maidment D.R. and Mays L.W. 1988. Applied Hydrology. McGraw-Hill Publishing Company (1988), ISBN: 0071001743 [20] USDA, Soil Conservation Service, (1972) National Engineering Handbook, Section 4: Hydrology. U.S. Government Printing Office,Washington, DC
[21] Saltelli A, K Chan and E Marrian Scoot, 2000, Sensitivity Analysis , Wiley 2000, ISBN: 0471998923