
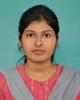
- Open Access
- Authors : Ekta Yadav, Sayantan Bhattacharya, Arunima Baur, Abhijit Majumder, Hiranmoy Samanta, Shubhadeep Mondal
- Paper ID : IJERTV13IS060134
- Volume & Issue : Volume 13, Issue 06 (June 2024)
- Published (First Online): 29-06-2024
- ISSN (Online) : 2278-0181
- Publisher Name : IJERT
- License:
This work is licensed under a Creative Commons Attribution 4.0 International License
Internet of Medical Things (IOMT) for Chronic Disease Management
Ekta Yadav Department of Pharmacy, Flemming College of Pharmacy, |
Sayantan Bhattacharya Department of Pharmacy, Flemming College of Pharmacy, |
Arunima Baur Department of Pharmacy, Flemming College of Pharmacy, |
Abhijit Majumder Department of Mechanical Engineering, Gargi Memorial Institute of Technology, |
Hiranmoy Samanta Department of Mechanical Engineering, Gargi Memorial Institute of Technology, |
Shubhadeep Mondal Department of Pharmaceutical Technology Jadavpur University |
Abstract:
Countries with aging populations are increasingly struggling with chronic diseases. Internet of Things (IoT) technology presents promising solutions to alleviate these chronic disease burdens. However, despite numerous studies on IoT for chronic disease management, comprehensive overviews are rare. This review aims to provide a detailed overview of IoT applications in chronic disease management and prioritize different chronic diseases for IoT use in developing countries. The study employs structural coding to list the technologies currently in use and applies the latent Dirichlet allocation algorithm to identify key topics in the literature. Additionally, to rank chronic diseases by their IoT applicability, a fuzzy analytic hierarchy process was used on common categories of chronic diseases. The findings include lists of IoT technologies for chronic disease management and the most- discussed chronic diseases. Text mining revealed 18 significant topics from the literature. The results show that cardiovascular disease, followed closely by diabetes mellitus, are the top priorities for IoT application in developing countries.
Keywords :
Chronic Disease,IoT, Latent Dirichlet Allocation(LDA),Fuzzy analytic hierarchy process (FAHP)
Chronic disease management, Internet of Things (IoT) for health care, Smart health care, IoT technologies for chronic diseases, Chronic disease in elderly populations, Latent Dirichlet allocation in health care, Fuzzy analytic hierarchy process, Chronic disease prioritization in developing countries, Cardiovascular disease management with IoT, Diabetes management with IoT.
Research Methodology:
A selection of articles related to literature analysis and IoT applications in chronic disease management were reviewed. These reviews helped identify dimensions essential for understanding the existing literature
10,1217. Based on these identified dimensions, the current research questions (RQs) were formulated.
Research Questions The following research questions were explored in this study:
-
What chronic diseases are most discussed?
-
Which technologies are utilized in IoT for managing chronic diseases?
-
How are documents distributed across these topics?
-
Which topics are commonly addressed in the literature?
-
Which chronic diseases are prioritized for IoT applications?
INTRODUCTION :
The Internet of Things (IoT) provides extensive solutions to enhance healthcare quality while reducing costs, making it crucial for sustainable health-care delivery. IoT, comprising interconnected smart devices like sensors and smartphones, enables continuous interaction for effective service delivery. It revolutionizes traditional healthcare by offering continuous care, accurate prognoses, regional health strategy dissemination, treatment compliance monitoring, personalized treatment plans, and valuable insights from collected data, especially benefiting chronic condition management. Chronic diseases, particularly in developing countries, are rising, with WHO projecting increased prevalence until 2030. Abegunde et al. predicted significant economic losses due to chronic diseases without intervention. Hence, IoT's potential for cost-effective healthcare solutions is critical. This study aims to compile an extensive overview of IoT applications for chronic disease management. It details methodologies, presents findings, and discusses results, emphasizing IoT's role in addressing technological, security, and infrastructure challenges to enhance global health systems.
Remote Patient Monitoring:
The Internet of Medical Things (IoMT) facilitates the continuous and remote monitoring of patients vital signs, medication adherence, and disease progression. This real-time data acquisition enables healthcare providers to take proactive measures, detect early warning signs, and deliver timely interventions. Such capabilities significantly improve patient outcomes and reduce the frequency of hospital admissions. By constantly tracking a patient's health metrics, IoMT ensures that any potential issues are identified and addressed promptly, thereby preventing complications and promoting efficient healthcare delivery.
Telemedicine and Telehealth:
IoMT greatly enhances telemedicine and telehealth services by enabling virtual consultations, remote diagnostics, and continuous patient monitoring. Through secure and reliable connectivity, healthcare professionals can perform remote examinations, offer consultations, and track patients' health progress. This technology not only broadens access to healthcare in underserved and rural areas but also alleviates the pressure on overburdened healthcare facilities. By facilitating remote interactions, IoMT ensures that patients receive timely medical advice and care without the need for physical visits, thus optimizing resource allocation and improving patient satisfaction.
Chronic Disease Management:
IoMT devices, such as wearable sensors and smart implants, provide continuous monitoring for chronic conditions like diabetes, cardiovascular diseases, and respiratory disorders. These devices collect and transmit real-time data to healthcare providers, alerting them to any abnormalities. This continuous flow of information allows for prompt, proactive interventions and the development of personalized treatment plans tailored to each patient's unique needs. By maintaining constant surveillance of chronic conditions, IoMT helps in managing diseases more effectively, reducing the risk of severe complications and improving the overall quality of life for patients.
Medication Management:
IoMT technologies improve medication adherence through innovative solutions such as smart pill dispensers, wearable devices, and mobile applications. These tools remind patients to take their medications, track their compliance, and provide detailed data to healthcare providers. This continuous monitoring helps in minimizing medication errors and ensuring that patients follow their prescribed treatment regimens. By enhancing adherence and providing healthcare providers with accurate compliance data, IoMT contributes to more effective treatment outcomes and improved patient health.
Data Analytics and Predictive Analytics:
The extensive data generated by IoMT devices can be leveraged for advanced analytics and predictive modeling. This enables healthcare providers to identify trends, predict disease outbreaks, and optimize resource allocation. By analyzing patient data, healthcare professionals can develop personalized treatment plans that cater to the specific needs of each individual.
Predictive analytics also allows for early intervention and prevention strategies, ultimately leadig to better healthcare outcomes and more efficient management of healthcare resources.
Operational Efficiency:
IoMT applications enhance operational efficiency in healthcare by automating administrative tasks, optimizing resource utilization, and improving workflow processes. This leads to significant cost savings, increased productivity, and better overall efficiency in healthcare delivery. By streamlining operations and reducing manual workload, IoMT ensures that healthcare providers can focus more on patient care and less on administrative tasks. This results in a more effective healthcare system, with improved patient satisfaction and outcomes. https://appinventiv.com/blog/internet-of-medical-things-in-
health-sector
STRUCTURAL CODING
To recognize advancements engaged with and utilizing IoT for chronic disease management, a thorough search and analysis of related research were conducted. This analysis was conducted using an index of a vast array of academic journals, books, and conference proceedings. The term "wireless sensor + chronic disease" was included because wireless sensor networks are integral to IoT and have played a crucial role in its development. The analysis was performed on February 18, 2019, without restricting the publishing year to ensure comprehensive coverage of indexed contributions up to that date. Overlaps in search results, particularly among the first and second terms, were noted, leading to the inclusion of both terms to increase reliability. Additionally, literature surveys identified in the results were considered for their comprehensive lists of IoT applications.
This search strategy resulted in 161 relevant contributions. As a supplementary measure, the WHO's list of chronic diseases was used as a checklist to ensure comprehensive coverage. Each of the 161 journal articles were examined to identify the chronic diseases. For diseases with few or no relevant contributions, further searches were conducted using Google Scholar with the term "Internet of things" and, if necessary, "wireless sensor network."
The process involves coding questionnaire before consolidating and obtaining relevant data. Structural coding facilitates the recognition of commonalities, variations, and relationships within the data, acting as an indexing framework to focus on pertinent information for specific analyses.
Utilizing structural coding, relevant sections pertaining to advancements were determined for further study. While reading selected articles addressed the research question, structural coding was adopted to mitigate uncertainty from articles that did not clearly indicate the technologies used.
Fig 1 : Structural Coding Method
QUANTEDA TECHNIQUE
Various computer-assisted tools have been developed for qualitative data analysis. Initially, content of 186 contributions was converted to plain text format and fed into the R software. Using Quanteda tutorials and modified code, searches were conducted for each chronic disease listed by the WHO within these texts. For each occurrence, a context of 60 words before and 60 words after the mention was extracted to ensure the references were part of the scientific discussion rather than metadata like affiliations, conference titles, journal names, or citations. This manual inspection was crucial to prevent misclassification, such as mistakenly identifying a paper as discussing cancer due to an authors affiliation with a cancer center.
The technique for identifying the chronic diseases had several notable considerations. First, contributions that mentioned a chronic disease in short could be misclassified as thoroughly discussing it. Second, some contributions might offer solutions applicable to multiple diseases but only explicitly mention a few. Third, the WHO list includes an "other diseases" category, which means not all chronic diseases are individually listed. However, these considerations were deemed to have minimal impact on the results for several reasons: significant chronic diseases would likely be mentioned repeatedly by authors; brief mentions were rare and generally insufficient to rank a disease as most- discussed (with manual inspections performed to avoid this issue); and while the WHO list may not be exhaustive, it likely
includes the most significant diseases, with other significant diseases potentially appearing in latent Dirichlet allocation (LDA) results.
Using Quanteda for this analysis provided a structured approach to identifying key chronic diseases discussed in the literature, contributing to a more comprehensive understanding of the IoT's role in chronic disease management. This method enabled efficient processing and analysis of large text datasets, ensuring relevant diseases were accurately identified and discussed in the context of IoT applications.
LATENT DIRICHLET ALLOCATION
An objective of the present study was to evaluate major topics in the selected pieces of literature. For this purpose, Latent Dirichlet Allocation (LDA) was applied. Before implementing this algorithm, the acquired data needed to be converted into plain text, facilitating cleaning up and other preprocessing steps. In research, this prepared data for the LDA algorithm is typically referred to as clean data. For further details, interested readers can refer to Sherman's book on text mining and data preparation. The generated word clouds visually represented the most significant words in the corpus, aiding in the intuitive understanding of prevalent themes. Identifying the optimal number of topics involved using metrics like perplexity and coherence scores, which helped balance model complexity with interpretability.
IJERTV13IS060134
(This work is licensed under a Creative Commons Attribution 4.0 International License.)
Through the application of LDA, the study was able to reveal the underlying structure of topics within the study on IoT for chronic disease management. This approach not only highlighted the main areas of focus but also provided insights into how these topics are interrelated. For instance, topics might include themes such as "remote patient monitoring," "data privacy and security," "wearable health devices," and "chronic disease-specific IoT applications." Each topic was characterized by a set of keywords that were most representative of that theme.
Furthermore, analyzing the distribution of documents among different topics helped understand the prominence and relevance of each theme across the body of literature. This distribution
analysis provided a quantitative basis for prioritizing areas of research and identifying gaps where further studies could be beneficial.
In summary, the use of LDA in this study enabled a systematic and comprehensive analysis of the literature on IoT applications for chronic disease management. By identifying and categorizing major topics, this method provided valuable insights into current research trends and highlighted potential areas for future exploration. This robust analytical approach enhances our understanding of how IoT technology is being utilized to address chronic health conditions, offering a foundation for continued innovation and development in this critical field.
Fig. 2 Latent Dirichlet Allocation Method[Sources: Scaler
Fuzzy Analytic Hierarchy Process (FAHP) for Prioritizing Chronic Diseases for Internet of Things
FAHP is particularly useful for ranking chronic diseases due to its ability to handle fuzzy logic. Chang developed an extent analysis method for FAHP, which has become widely popular. The FAHP model involved several key steps. First, criteria and alternatives were established based on literature and expert input. Each alternative was then evaluated against the criteria using pair-wise comparisons, which involved expert judgments to determine relative importance. This process was facilitated by FAHP's capacity to incorporate fuzzy loic, which addresses the inherent uncertainties in human judgment.
The method proposed by Gogus and Boucher was used in this research, which recognizes a matrix as consistent if the values of two consistency rates (CR), CRm and CRg, are equal to or lower than 0.1 for each comparison matrix. This step was crucial for validating the reliability of the pair-wise comparison results. The FAHP analysis provided a prioritized ranking of chronic disease categories. The results pointed out that cardiovascular diseases as the utmost priority. This prioritization reflects the significant burden these diseases place on health systems and the potential for IoT technologies to mitigate this burden effectively. The outcomes of the FAHP model have important implications for policy makers and health care providers in developing countries. By highlighting which chronic diseases should be
prioritized for IoT solutions, resources can be allocated more efficiently to areas where they will have the most significant impact. This approach not only enhances the effectiveness of
health care interventions but also supports the development of targeted strategies for chronic disease management using IoT technologies.
Fig 3 A Fuzzy Analytical Hierarchical Process Model for Classification of Different Categories of Chronic Diseases Based on Their Priority Using IoT in Small.
RESULTS
This section details the outcomes from applying each method. Although we differentiate between findings from different methods for clarity, they are interrelated. Together, these findings offer a comprehensive overview of the literature.
Chronic Disease Management with IoT: Technologies and Top Conditions
This section explores the connection between chronic diseases and technology used for managing them, particularly the Internet of Things (IoT).
Fig 4 Chronic Disease Management Using the Internet of Things: Technologies.
Technologies for IoT-based Chronic Disease Management:
Sensors and Devices: These gather data on a patient's health vitals or surrounding environment.
Data Processing:
-
Big Data: Stores and analyzes large amounts of health data.
-
Cloud Computing: Enables data storage and processing
accessible from anywhere.
-
Fog Computing: Analyzes data locally (at the "edge") for quicker response times.
Security and Privacy:
-
Blockchain: Protects data privacy through secure record- keeping.
-
Semantic Technology: Creates models to understand
relationships between health data points.
Most Discussed Chronic Diseases:
-
Diabetes
Cardiovascular diseases
The study also identified other chronic diseases where IoT has potential applications, like cancer, asthma, and
depression.
Future Research Directions:
-
Developing new IoT applications for less-explored chronic diseases.
-
Designing customized IoT strategies for different regions.
-
Creating new sensors to monitor emerging health parameters.
-
Addressing security and privacy concerns in IoT-based healthcare.
Overall, IoT offers promising ways to manage chronic illnesses. Further research can improve existing solutions and expand their reach to benefit more patients.
Major Themes in the Literature on IoT for Chronic Disease Management
An extensive literature analysis pertaining to the integration of Internet of Things (IoT) technologies in chronic disease management reveals several recurring themes and trends.1. Focus on Patient Monitoring: One of the predominant themes identified is the emphasis on patient monitoring through the utilization of various sensors and data collection methodologies. Keywords such as "patient," "data," "system," "sensor," "use," "health," "technology," and "monitor" underscore the paramount importance of monitoring patients' health parameters and collecting relevant data.2. Latent Dirichlet Allocation (LDA) Analysis: Researchers employed the LDA analysis technique to discern recurring topics within the literature corpus. This method necessitated the determination of the optimal number of topics, typically falling within the range of 18 to 22, to effectively capture the breadth of discussions.3. Topical Identification through LDA: Leveraging the Gibbs sampling method within the LDA framework, researchers identified the top ten keywords associated with each topic. These keywords served as anchors for the subsequent labeling of topics, ensuring coherence and relevance in topic identification.4. Predominance of Data Collection in IoT: A notable finding from the analysis is the prominence of topics related to data collection within IoT frameworks. This observation aligns with the fundamental role, particularly in context gathering vital health data through sensor- based technologies.5. Implications for Future Research: The distribution of topics across the literature provides valuable insights into prevailing research trends and areas warranting further exploration. Broader topics, such as data collection in IoT, emerge as focal points for future research endeavors, underscoring the need for continued innovation and advancement in chronic disease management practices.6. Informing Strategic Interventions: The synthesis of literature findings serves to inform strategic interventions aimed at enhancing the efficacy and scope. By elucidating key themes and research trajectories, this analysis lays the groundwork for targeted initiatives aimed at improving patient outcomes and healthcare delivery.
Prioritization of Chronic Diseases for IoT Implementation
The survey questionnaire comprised three key questions pertaining to criteria for ranking and alternatives for each criteria. To ensure validity of responses, participants were provided with comprehensive information regarding the study objectives, IoT technology.
Utilizing the consistency ratio (CR), participants were prompted to revise their responses if the rate exceeded 0.1, ensuring the reliability of the collected data.
The work prioritized preventing of fatality held greater significance over cost reduction. Consequently, decision-makers are advised to base their strategies on prioritizing fatality prevention over cost considerations. However, regardless of the selected criterionwhether cost reduction, fatality prevention, or a combination of bothcardiovascular diseases and diabetes mellitus emerged as the diseases warranting higher priority compared to other chronic conditions such as malignant neoplasms or respiratory diseases.
This insight underscores the critical importance of considering both the health outcomes and economic implications when determining the optimal allocation of resources and the deployment of IoT technologies in chronic disease management. By prioritizing diseases based on their potential for fatality prevention and cost-effectiveness, healthcare managers can effectively strategize and allocate resources to maximize the impact of IoT interventions in combating chronic diseases.
The synthesis of literature pertaining to the utilization of Internet of Things (IoT) in chronic disease management provides nuanced insights into the evolving landscape of healthcare technology and research priorities.
In-depth Analysis from Literature Review:
-
A thorough examination of the literature elucidated the primary technologies at play in IoT-driven chronic disease management. Central to this discussion is the pivotal role played by sensor- driven data collection methodologies, which form the backbone of IoT applications in healthcare.
-
Through the appication of Latent Dirichlet Allocation (LDA) analysis, prominent themes within the literature were identified. Notably, topics such as data collection in IoT emerged as dominant, underscoring the fundamental importance of this aspect in chronic disease management strategies.
-
It revealed cardiovascular diseases and diabetes mellitus as focal points due to their significant prevalence and potential for impactful IoT interventions.
Implications and Future Research Directions:
-
While certain chronic diseases have received substantial attention, there exists a critical need for further exploration into the application of IoT technologies in managing conditions such as Parkinson's disease, Alzheimer's disease, epilepsy, and respiratory disorders.
-
Additionally, the discussion emphasized the imperative of addressing security and privacy concerns inherent in IoT-enabled chronic disease management, reflecting broader apprehensions within the healthcare sector regarding data protection and confidentiality.
Recommendations for Advancing the Field:
-
Future research endeavors should adopt a more targeted approach by focusing on specific categories of chronic diseases, integrating relevant sensor technologies and platforms, and delineating comprehensive guidelines for future investigations.
-
It is crucial to acknowledge the limitations inherent in this study, including the potential for inaccuracies in topic labeling and the reliance on a limited pool of expert opinions.
Concluding Remarks:
-
In conclusion, this research enriches the existing body of literature by offering multifaceted insights into the utilization of
IoT in chronic disease management. By employing a robust methodology encompassing structural coding, LDA, and the Fuzzy Analytic Hierarchy Process (FAHP), this study provides a holistic understanding of technological trends, research priorities, and challenges in this dynamic field.
Final Note:
-
Interested readers are encouraged to explore review articles that delve deeper into the intricate nuances of IoT applications in healthcare, offering nuanced perspectives and further enriching the discourse on this burgeoning domain.
### R Packages Utilized in the Current Study
-
**RColorBrewer**: Color palettes inspired by Cynthia Brewer's color schemes86
-
**readtext**: Reading text data into R93
-
**SnowballC**: Snowball stemmers based on the C libstemmer UTF-8 library84
-
**ggplot2**: Data visualization using the grammar of graphics91
-
**ldatuning**: Tuning of the Latent Dirichlet Allocation models88
-
**summarytools**: Tools to quickly and neatly summarize data94
-
**tm**: Text mining framework for R82, 83
-
**wordcloud**: Word clouds85
-
**tidytext**: Text mining using tidy data principles90
-
**dplyr**: Data manipulation tools92
-
**topicmodels**: Topic modeling89
-
**quanteda**: Quantitative analysis of textual data30
-
**readxl**: Read Excel files (xls and xlsx)87
-
REFERENCE :
-
Zubiaga A, Procter R, Maple C. A longitudinal analysis of the public perception of the opportunities and challenges of the Internet of Things. PLoS One 2018;13:e0209472.
-
Couturier J, Sola D, Borioli GS, Raiciu C. How can the internet of things help
to overcome current healthcare challenges. Digiworld Econ J 2012;87:6781.
-
Vermesan O, Friess P, Guillemin P, Sundmaeker H, Eisenhauer M, Moessner K, et al. Internet of Things Strategic Research and Innovation Agenda. IERC- European Research Cluster on the Internet of Thing; 2014.
-
Li S, Xu LD, Zhao S. The internet of things: A survey. Inf Syst Frontiers
2015;17:24359.
-
Wang SH, Ding Y, Zhao W, Huang YH, Perkins R, Zou W, et al. Text mining for identifying topics in the literatures about adolescent substance use and depression. BMC Public Health 2016;16:279.
-
Guillemin P, Friess P. Internet of Things Strategic Research Roadmap. Cluster of European Research Projects on the Internet of Things. IERC-European Research Cluster on the Internet of Things; 2009. p. 150.
-
Abegunde DO, Mathers CD, Adam T, Ortegon M, Strong K. The burden and costs of chronic diseases in lowincome and middleincome countries. Lancet 2007;370:192938.
-
Giusto D, Iera A, Morabito G, Atzori L. The Internet of Things: 20th Tyrrhenian Workshop on Digital Communications. New York: Springer; 2010. Available from: https://books.google.nl/books?id=vUpiSRc0b7AC. [Last accessed on 2020 May 03].
-
Jagadeeswari V, Subramaniyaswamy V, Logesh R, Vijayakumar V. A study on medical Internet of Things and Big Data in personalized healthcare system. Health Inf Sci Syst 2018;6:14.
-
Chou D. What Can IoT do for Healthcare?; 2016. Available form:
https://www.cio.com/article/3117385/internetofthings/whatcaniotdofor
healthcare.html. [Last accessed on 2019 Jan 02].
-
Nugent R. Chronic diseases in developing countries: Health and economic
burdens. Ann N Y Acad Sci 2008;1136:709.
-
Patra J, Popova S, Rehm J, Bondy S, Flint R, Giesbrecht N. Economic Costs of Chronic Disease in Canada 19952003. Ontario chronic disease prevention alliance and the ontario public health association Canada; 2007. p. 137.
-
Saldaña J. The Coding Manual for Qualitative Researchers. London: Sage; 2015.
-
Haynes E, Garside R, Green J, Kelly MP, Thomas J, Guell C. Semiautomated text analytics for qualitative data synthesis. Res Synthesis Methods 2019;10:452-64. [doi: 10.1002/jrsm.1361].
-
de Morais Barroca Filho I, de Aquino Junior GS. IoTbased healthcare applications: A review. In: Gervasi O, Murgante B, Misra S, Gervasi O, Murgante B, Misra S, Borruso G, Torre CM, Rocha AMAC, et al., editors. Computational Science and Its Applications ICCSA 2017. Switzerland: Springer International Publishing; 2017. p. 4762.
-
Liberatore MJ, Nydick RL. The analytic hierarchy process in medical and health care decision making: A literature review. Eur J Oper Res 2008;189:194207.
-
Turcu CE, Turcu CO. Internet of things as key enabler for sustainable healthcare delivery. Procedia Soc Behav Sci 2013;73:2516.
-
Yang K, Meho LI. Citation analysis: A comparison of Google Scholar,
Scopus, and Web of Science. Procee Am Soc Inf Sci Technol 2006;43:115.
-
Benoit K, Watanabe K, Wang H, Nulty P, Obeng A, Müller S, et al. Quanteda: An R package for the quantitative analysis of textual data. J Open Source Softw 2018;3:774.
-
Adeyi O, Smith O, Robles S. Public Policy and the Challenge of Chronic Noncommunicable Diseases. Washington, DC: The International Bank for Reconstruction and Development/The World Bank; 2007.
-
Islam SM, Kwak D, Kabir MH, Hossain M, Kwak K. The internet of things
for health care: A comprehensive survey. IEEE Access 2015;3:678708.
-
Quick Stat Guide Quanteda. Available from: https://quanteda.io/articles/quickstart.html. [Last accessed on 2019 Jun 03].
-
Sugimoto CR, Li D, Russell TG, Finlay SC, Ding Y. The shifting sands of disciplinary development: Analyzing North American Library and
Information Science dissertations using latent Dirichlet allocation. J Am Soc
Inf Sci Technol 2011;62:185204.
-
Guest G, MacQueen KM, Namey EE. Applied Thematic Analysis. USA: Sage Publications; 2011.
-
Sherman R. Business Intelligence Guidebook: From Data Integration to Analytics. Newnes; 2014.
-
Paez DG, Aparicio F, de Buenaga M, Ascanio JR. Big Data and IoT for
Chronic Patients Monitoring. Cham, Switzerland: Springer; 2014. p. 41623.
-
MacQueen KM, Guest G. An introduction to teambased qualitative research. Handbook for TeamBased Qualitative Research. AltaMira Press, UK: 2008.
p. 319.
-
Namey E, Guest G, Thairu L, Johnson L. Data reduction techniques for large
qualitative data sets. Handbook for TeamBased Qualitative Research. Vol.
2. AltaMira Press, UK: 2008. p. 13761.
-
Saaty TL. A scaling method for priorities in hierarchical structures. J Math Psychol 1977;15:23481.
-
Saaty TL. Analytic hierarchy process. In: Gass S.I., Harris C.M. (eds) Encyclopedia of Operations Research and Management Science. New York, NY: Springer; 2001. p. 5264.
-
Saaty TL. How to make a decision: The analytic hierarchy process. Eur J Oper
Res 1990;48:926.
-
Kubler S, Robert J, Derigent W, Voisin A, Le Traon Y. A stateof theart survey & testbed of fuzzy AHP (FAHP) applications. Expert Syst Appl 2016;65:398422.
-
Nikita M. Select Number of Topics for LDA Model; 2016. Available from: https://cran.rproject.org/web/packages/ldatuning/vignettes/topics.html. [Last accessed on 2019 Mar 31].
-
Wu Y, Liu M, Zheng WJ, Zhao Z, Xu H. Ranking genedrug relationships in biomedical literature using latent Dirichlet allocation. In: Biocomputing 2012. Singapore: World Scientific; 2012. p. 42233.
-
Haley DF, Vo L, Parker KA, Frew PM, Golin CE, Amola O, et al. Qualitative methodological approach. In: OLeary A, Frew PM, editors. Poverty in the United States: Womens Voices. Ch. 2. Cham: Springer International Publishing; 2017. p. 923.
-
Sherman R. Business Intelligence Guidebook: From Data Integration to Analytics. Newnes; 2014.
-
Dadkhah M, Lagzian M, Santoro G. How can health professionals contribute to the Internet of Things body of knowledge? A phenomenography Study. VINE J Inf Knowl Manage Syst 2019;49:299-40.
-
Chang DY. Applications of the extent analysis method on fuzzy AHP. Eur J
Oper Res 1996;95:64955.
-
Özdaolu A, Özdaolu G. Comparison of AHP and fuzzy AHP for the multicriteria decision making processes with linguistic evaluations. stanbul Ticaret Üniver Fen Bilimleri Derg 2007;6:6585.
-
Zubiaga A, Procter R, Maple C. A longitudinal analysis of the public perception of the opportunities and challenges of the Internet of Things. PLoS One 2018;13:e0209472.
-
Sugimoto CR, Li D, Russell TG, Finlay SC, Ding Y. The shifting sands of disciplinary development: Analyzing North American Library and Information Science dissertations using latent Dirichlet allocation. J Am Soc Inf Sci Technol 2011;62:185204.
-
Nikita M. Select Number of Topics for LDA Model; 2016. Available from:
https://cran.rproject.org/web
IJERTV13IS060134
(This work is licensed under a Creative Commons Attribution 4.0 International License.)