
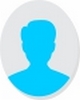
- Open Access
- Authors : Tekalign Lemma Woldesilassie , Venkata Mallikhajuna Kishan Ivatury
- Paper ID : IJERTV9IS020295
- Volume & Issue : Volume 09, Issue 02 (February 2020)
- Published (First Online): 02-03-2020
- ISSN (Online) : 2278-0181
- Publisher Name : IJERT
- License:
This work is licensed under a Creative Commons Attribution 4.0 International License
Key Determinant Factors Affecting the Performance of Small and Medium Scale Manufacturing Enterprise: A Case Study on West Shoa Zone, Oromia National Regional State, Ethiopia
Tekalign Lemma Woldesilassie
Department of Industrial Engineering, Institute of Technology, Ambo University,
Ambo, Ethiopia
Venkata Mallikhajuna Kishan Ivatury
Department of Industrial Engineering, Institute of Technology, Ambo University,
Ambo, Ethiopia
Abstract: This study was focused on the assessment of key determinant factors affecting small- and medium scale manufacturing enterprise (SSME). The study was conducted in west Shoa Zone Districts Oromia National State, Ethiopia. From a total population of N=696 wood work and metal work manufacturing enterprise among 18 districts, a sample size of (n= 153) were selected through simple random sampling techniques. Based on the literature review and conceptual frame work developed eight hypothesis were developed and tested. Eight independent variables considered are labor and personal factors, material factors, equipment and machinery factors, marketing ingredient, production techniques, product quality factors, productivity factors, other factors and dependent variables organizational performance. The results were presented in the form of mean, standard deviation, Pearson correlation, ANOVA and regression analysis. The finding of the study shows that seven independent variables accounted 78.7% for the performance of the enterprise. Five factors shows the positive effect and statistically significant determinants for the performance of the firm except production techniques, equipment and machinery factors.
Keywords Small and Medium Scale Manufacturing Enterprise; Organisational Performance; Correlation Analysis; Multiple Linear Regression
-
INTRODUCTION
Small and medium scale manufacturing enterprise (SMSI) has important role in the economy of any country. They create employment and acts as an engine of economic growth in developing countries. They play a key role in triggering economic growth and equitable development in developing countries. Medium scale manufacturing enterprise (MSEs) exerts a strong influence on the economic development of all countries, particularly in the developing countries and reported that they are major engine in the economic growth, innovation and technological progress of any country [1].
Ethiopia being the one of the fastest developing economy in Africa identified the development of micro and small manufacturing enterprises as key strategic sector to
reduce poverty and unemployment and also to transform the economy from agriculture based one to manufacturing based one. The country has been investing large amount of money in Small and Medium enterprises, especially for manufacturing sectors with a view to improve the socio- economic condition of the people. Small and micro enterprise plays a vital role in the improvement of the country gross domestic product (GDP), generating additional capitals, for ensuring flow of goods to the nations and reduce unemployment rate [2].
Small and medium scale manufacturing enterprises performance is affected by different factors (problems). Lack of knowledge and skills of basic marketing ingredients like marketing research, market segmentation and marketing planning and control are the major factors that affect the performance of small and medium scale enterprise. The main factors that affect the performance of MSEs are access to markets, as well as lack of information and lack of finance and institutional support [3] and [4]. Two primary causes of small business failures are identified as lack of appropriate management skills and inadequate or lack of capital. Lack of managerial training, technical skills and experience [5]
The level of training, experience and number of years in operation, knowledge of the market, level of differentiation (in terms of price, quality or other) and diversification of products, access to the necessary resources and/or technologies and level of planning as the challenges faced by MSEs are identified as the main factors affecting organizational performance [6]. Lack of managerial skills leads to problems in production due to lack of coordination in production process, and inability to identify failures of machinery and equipment are some other factors affecting organizational performance [7]. Many MSEs owners or managers lack managerial training and experience. The typical owner or managers of small scale manufacturing enterprise develop their own approach to management, through a process of trial and error method. Consequently owners are ill prepared to face
changes in the business environment and to plan for appropriate changes in technology. Entrepreneurs level of poverty is one of the factors contributing to the poor performance of MSEs. One of the major challenges in Small scale enterprise development in Ethiopia is supply of raw materials. Linking small scale manufacturing enterprise to raw material input suppliers, improving suppliers capacity and regular supply of quality information on input supply sources have positive effects on the performance of the enterprise [8] and [9].
The factors affecting the performance of micro and small enterprises in Arada and Lideta sub-cities, Addis Ababa, Ethiopia and identified poor selection of employees, lack of strategic business planning, inaccessible and costly training facilities, lack of persistence and courage to take responsibility for ones failure and absence of initiative to assess ones strengths and weaknesses are the main problems [10]. The findings of [11] shows that experience, planning practice, record keeping practice, access to finance and access to raw materials (inputs), socio-demographic variables (age and education level of the respondents) significantly and positively affect the performance of the micro and small scale enterprises. Factors like lack of adequate finance, lack of working premise, lack of managerial and technical skill, lack of adequate market and lack of infrastructure affect the performance of micro and small enterprises [12]. Lack of capital and inappropriate locations are major problems affecting the performance of MSEs [13]. Financial problem, lack of qualified employees, lack of proper financial records, marketing challenges, lack of working premises and raw materials, lack of information about market opportunities and standards and regulations are identified as the factors that hinder the performance of MSEs [14]. Lack of integration between the vocational curriculum taught at academic institutions and skills required at the workplace in small businesses and enterprises is a major obstacle for the growth and development of MSEs [15].
From the above discussion, it is obvious that few studies have been done on factors affecting the
.
performance of SMES. Most of the research conducted in Ethiopia in the study area did not analyze anything about the key determinant factors affecting the performance of medium scale manufacturing enterprise. In addition they did not identify key determinant factors depending on the types of sectors (manufacturing and service). These sectors have different working culture, different types of product (output), behavior, and different competitors; different in the use and level of technology, different types of layout, etc, In addition most of research conducted in the study area carried out in sub cities of Addis Ababa and at zonal level such as Awassa, Nekemte, Diredewa and Hossana. Little or no research has been done in west Shoa zone, Oromia national regional stat at woreda (District) level in manufacturing sectors. This research is thus undertaken to fill the gap, by identifying and assessing key determinant factors affecting the success or performance of small scale manufacturing enterprise. For the purpose of this study the researchers classified the key determinant factors that affect the performance of the enterprise in to eight input factors- labor and personal factor, production techniques factor, equipment and machinery factor, material factors, market ingredients factor, other factors, product quality factor and productivity factor. The output (organizational performance) is measured by performance measuring factors- profitability, sales volume, market share and customer satisfaction.
-
CONCEPTUAL FRAME WORK AND
HYPOTHESIS
Based on the literature reviews, a new conceptual frame work was developed for identifying and evaluating key determinant factors affecting the performance of small and medium scale manufacturing enterprise. This conceptual frame work is described in figure 1. It considers the relationships between eight input factors- labor and personal, material, machinery and equipment, production techniques, marketing ingredient, other factors, product quality and productivity with organizational performance
Fig. 1 Conceptual frame work of the study developed the researchers
In accordance with the literature and Conceptual frame work the following hypotheses were developed.
Hypothesis 1: There is a positive relationship between labor and personal factor and organizational performance.
Hypothesis 2: There is a positive relationship between market ingredients and organizational factors
Hypothesis 3: There is a positive relationship between equipment and machinery factors and organizational performance.
Hypothesis 4: There is a positive relationship between production technique and organizational performance
Hypothesis5: There is a positive relationship between other factors and organizational performance.
Hypothesis6: There is a positive relationship between material factors and organizational performance.
Hypothesis7: There is a positive relationship between product quality and organizational performance.
Hypothesis8: There is a positive relationship between productivity factors and organizational performance.
-
Mathematical Model
Mathematical models were develop to conduct multiple linear regression analysis in order to identify which independent variables (input factors) were most important and study the relationship between a dependent variables and a set of independent variables.
Mode l: The effects of input factors on organizational performance
OP=f (IF) =f (LP, PT, EM, MF MI, OF, PQ, PF)
Where: – OP= Organizational Performance, IP= Input Factor, LP= Labor and Personal Factor
PT= Production Techniques Factor, EM= Equipment and Machinery Factor,
MF= Material Factors, MI= Market Ingredients Factor, OF= Other Factors,
PQ= Product Quality Factor, PF= Productivity Factor
-
-
RESEARCH METHODOLOGY
-
Research Design, Data Collection, Instrument, Procedure, and Analysis Methods
TABLE 1. CRITERIA TO SCALE MEAN SCORE [16]
Degree
Range
Strongly dissatisfied (not true at all)
1.001.80
Dissatisfied (true to minimal degree)
1.812.60
General (true to a moderate degree)
2.613.40
Satisfied (true to a high degree)
3.414.20
Very satisfied (absolutely true)
4.215.00
It is expected to investigate the key determinants of organizational performance in small scale manufacturing enterprise in Oromia National regional state westshoa zone, Ethiopia. This study used a descriptive and inferential statics in data analysis.
The data used to conduct this study were the primary data obtained through the five-point Likert scale questionnaires where the lowest scale is strongly disagree=1, disagree=2, neutral=3, agree=4, and strongly agree=5. The questionnaire contained close-ended questions. The questionnaires are distributed and collected by the
researcher by direct contact. Mean, standard deviation, Pearson correlation, ANOVA and regression analysis are carried out. Table 1 is used to express the degree of mean scores based on the criteria to scale rating of class interval.
-
Description of the Study Area (Target Population), Sample Size, and Sampling Procedure
The study was conducted at west shoa zone, Oromia National Regional State in the Westdirection of Addis Ababa. This zone has 18woredas (district):Ambo,
.
Tokekutaye, Ilu Gelan, Nono, Jibat, Dandi, Gindeberet, AbunaGindeberet, Ejere, Ada-Berga, Bako-Tibe, Cheliya, Dano, Jeldu, Midakegn, Meta Robi, and Tikur-Enchini. Each district has its own ub-capital cities. The main capital city of this district is Ambo town which is 115km from the capital city of Ethiopia-Addis Ababa. According to (CSA, 2007) the total population of west shoa zone is 2,058,676 of which 1,028,501 are men and 1,030,175 are women.
Fig. 2 Map of study area (West Shoa Zone)
-
Target population
The target populations of the research are owners of metal and wood work small scale manufacturing enterprise. The zone of the study area has 18 districts but this research was conducted in the 14 sub cities of the district (Ambo, Toke kutaye, Jibat, Nono, Midakegni, chelia, Bakotibe, Dano, Tikurenchine, Dendi, Ejerie, Abuna Gindaberet, Ginda beret and Jeldu.). The selected sub cities have relatively large number of metal and wood working manufacturing enterprise. So this 14 sub cities have a total of696 metal and wood working manufacturing enterprises.
-
Sampling technique and sampling size
West shoa zone was selected because of insufficient studies on key factors affecting performance of manufacturing enterprise. For this study both simple random sampling and stratifies probability sampling techniques were used to select the respondent. Based on this, among 18 districts, 14 districts were selected as these districts have large number of manufacturing enterprises. Finally the sample size (number of sample respondent) was determined by (Kothari, 2004) sampling design formula.
n=z2pqN/e2 (N-1) +z2pq Where: – n = sample size
N=Total number of population
Z= 95%confidence interval under normal curve (1.96) e=acceptance error term (0.07)
P and q=estimate of the proportion of population to be sampled (p=0.5 and p+q=1)
Based on this the sample size for the study was determined as
n=z2pqN/e2 (N-1) +z2pq
n=1.962×0.5×0.5×696/ (0.07)2(696-1)
+1.962X0.5X0.5
Based on this the total sample size was 153 manufacturing enterprise. Finally in order to proportionally distribute the sample among each district the following formula was used (Yaman, 1967).
nh=(Nh/Ns)n
Where: nh=sample size from each district, Nh= total population from each district, Ns=total population of sum of all district, n=total sample size from the population.
Table2: Sample size determination for each sub city
No
Woreda
sub-capital cities
Number of Enterprise Nh
nh=(Nh/Ns)n
1
Ambo
Ambo
112
25
2
Toke kutaye
Guder
43
9
3
Jibat
Shenen
27
6
4
Nono
Silkamba
16
4
5
Midakegni
Midakegn
21
5>
6
chelia
Gedo
76
17
7
Bakotibe
Bako
98
21
8
Dano
Ijaji
23
5
9
Tikurenchine
Dire-Inchini
19
4
10
Dendi
Ginchi
47
10
11
Ejerie
Addisalem
78
17
12
Abuna gindaberet
Kachise
48
11
13
Gindageret
Qalate
43
9
14
Jeldu
Jaldu
45
10
n=696
153
-
-
RELIABILITY ANALYSIS
The reliability of the data is checked using SPSS 20.Cronbachs alpha is calculated to determine the reliability of the data obtained questionnaires. Table 3 shows the reliability result of the construct alpha value before and after the construct is deleted which have a bad loading. Cronbachs alpha () < 0.6 indicates unsatisfactory internal consistency reliability (Malhotra& Birks, 2007) and > 0.7 indicates satisfactory internal
No. |
Description(factors) |
#item* |
Cronbach's Alpha |
|
Before item is deleted |
After item is deleted |
|||
1 |
Labor and personal |
7(12) |
0.878 |
0.903 |
2 |
Material |
3(7)* |
0.417 |
0.677 |
3 |
Production techniques |
4(4) |
0.893 |
0.893 |
4 |
Equipment and machinery |
3(3) |
0.644 |
0.742 |
5 |
Market ingredient |
4(5) |
0.644 |
0.701 |
6 |
Other factors |
4(7) |
0.783 |
0.904 |
7 |
Product quality |
4(9) |
0.550 |
0.692 |
8 |
Productivity |
4(7) |
0.857 |
0.944 |
9 |
Organizational performance |
4(4) |
0.757 |
0.757 |
No. |
Description(factors) |
#item* |
Cronbach's Alpha |
|
Before item is deleted |
After item is deleted |
|||
1 |
Labor and personal |
7(12) |
0.878 |
0.903 |
2 |
Material |
3(7)* |
0.417 |
0.677 |
3 |
Production techniques |
4(4) |
0.893 |
0.893 |
4 |
Equipment and machinery |
3(3) |
0.644 |
0.742 |
5 |
Market ingredient |
4(5) |
0.644 |
0.701 |
6 |
Other factors |
4(7) |
0.783 |
0.904 |
7 |
Product quality |
4(9) |
0.550 |
0.692 |
8 |
Productivity |
4(7) |
0.857 |
0.944 |
9 |
Organizational performance |
4(4) |
0.757 |
0.757 |
Table 3: Reliability results of the constructs
*item after deleting (item before deleting), scale 1-5
-
Descriptive Analysis of input factors
Findings of the study inTable4 revealed that the highest of all the mean values shows the presence of high degree of poor productivity practice( problem) due to ineffective and inefficient use of employees, inefficient use of machinery and equipment, and ineffective utilization of production time(working hrs.) (Mean=3.80, standard deviation= 1.145) The second highest is labor and personal factors practice (implementation) that shows the enterprise have high degree of poor implementation this factors (it) due to use of inexperienced workers, poor facility for workers, absence of training, lack of motivation system, insufficient labor income, and unavailability of skilled workers in the
market (mean = 3.67 , standard deviation =0.901).
The third highest input factor is under other factor practice that shows the enterprise have high degree of poor implementation in this factors (mean = 3.6454, standard deviation = 1.10532) due to high wastage of materials and availability of excessive rework and lack of government support both financial and non-financial)
The fourth highest mean values shows the presence of moderate degree of poor product quality (mean = 3.3578, standard deviation =.82506) due to
The fifth highest is under marketing ingredient practice that shows the enterprise have moderate degree of poor implementation of this factors (mean =3.3513, standard deviation = .83135) due lack of competing in the market, poor market planning and information flow.
consistency reliability (commonly accepted level) (Nunnally&Berstein, 1994). The finding showed that all have a Cronbachs Alpha value 0.6. Moreover, all of the items under each construct have a factor loading
0.55. The reliability of questionnaire indicates that the proposed constructs have a relatively higher reliability, ranging from 0.677 0.944, which is considered as more than satisfactory.
Sixth highest is under production technique practice that shows the enterprise have moderate degree of poor implementation of this factors (mean =3.2138, standard deviation =1.22792) due to lack of production planning, lack of designing the product before production, lack of using flexible manufacturing system and lack of knowledge to identify the specification.
Seventh highest is under material factor practice that shows the enterprise have moderate degree of poor implementation of this factors(mean= 3.0632, standard deviation = 0.89977)is due to quality problem of raw materials, unavailability of the required raw materials and lack of capital.
The last highest is under equipment and machinery factor practice that shows the enterprise have moderate degree of poor implementation of this factors (mean
=2.7124, standard deviation = .97787) due to use of inappropriate technology, lack of energy, lack of identifying the required equipment and machinery and lack of maintenance .
Table 4.Descriptive Statistics
Input factors
Mean
Standard Deviation
Labor and Personal Factors
3.6788
.90102
Material Factors
3.0632
.89977
Production Technique Factors
3.2138
1.22792
Equipment and Machinery Factors
2.7124
.97787
Market Ingredients Factors
3.3513
.83135
Other Facors
3.6454
1.10532
Product Quality Factors
3.3578
.82506
Productivity Factors
3.8072
1.14510
-
Organizational performance factors correlation coefficient analysis
Table 5 shows a correlation analysis which is used to examine the strength of the relationship between the independent variables like (PRFM, EMFM, MFFM, MIFM, OF, PTFM, LPF, PQFM) and dependent variables organizational performance (OP).
The result in Table 5 shows that organizational performance has strong positive and significant correlation with labor and personal factors (r=0.825, p<0.01), material
factors(r= 0.618, p<0.01) and productivity factors(r=0.603, p<0.01).
On the other hand organizational performance has a moderate positive correlated with marketing ingredients(r=0.380, p<0.01) and product quality(r=0.371, p<0.01). In addition the result indicates that organizational performance has weak correlation with production techniques(r=0.287, p<0.01).On the other hand the finding indicated that 0.And other factor has very weak negative correlation with organizational performance (r= -0.052, p<0.01)
Table 5: Correlation matrix of input factors and mean organizational performance factors (N=153)
1
2
3
4
5
6
7
8
9
1
1
.644**
.000
.274**
.000
.316**
.000
.386**
.000
.032
.348
.376**
.000
.394**
.000
.825**
.000
2
1
.195**
.008
.272**
.000
.326**
.000
.027
.372
.320**
.000
.230**
.002
.618**
.000
3
1
.591**
.000
.302**
.000
.298**
.000
.302**
.000
.163*
.022
.287**
.000
4
1
.387**
.000
-.126
.060
.400**
.000
.084
.150
.271**
.000
5
1
-.029
.363
.997**
.000
.215**
.004
.380**
.000
6
1
-.023
.390
-.031
.350
-.052
.263
7
1
.208**
.005
.371**
.000
8
1
.603**
.000
r=0.644**, p=0.000, ** Significant at the 0.01 level,* Significant at the 0.05 level where:-1= labor and personal factors, 2= material factors, 3= production technique, 4= equipment and machinery factors, 5= market ingredients, 6=other factors, 7=product quality, 8= productivity, 9= organizational performance
-
Multiple linear regression analysis of input factors and organizational performance
Regression analysis was conducted to examine the causal relationships between dependent variable (OP) and independent variables (LP, MF, PT, EM, MI, OF, PQ AND PR). The regression result explores the necessary indicators of organizational performance using the variables identified in the model.
As indicated in the model summary and coefficients and collinearity diagnostics (Tables 6 and 7, the appropriate indicators of the variable that are used to identify organizational performance were explored. The value of R square is used to identify how much of the variance in the dependent variable (OP) identifies the model. The larger the value of R square, the better the model is. The regression analysis method was used to regress the dependent variable on the independent variable (Tables 6 and 7). The independent variable (OF) were not entered as
it had no correlation with dependent variable as shown in the correlation coefficient matrix (Table 5).
Table 6 shows the R values for assessing the overall fit of the model. The adjusted R square value in this case is 0.787. This shows that the seven independent variables of the model account for 78.7%, the variance of its errors is 78.7% less than the variance of the dependent variable organizational performance. Clearly this is a very good model as there are factors other than the seven input factors which should also be used to predict organizational performance. The regression coefficient R = 0.887 shows the strength of the causal relationship between the dependent and independent variable. Model 1 was able to explain 88.7% of the observations (Table 5). The adjusted R square was 0.787 the model estimated shows that there was 78.7% positive variation in organizational performance as a result of changes in input factors explained by our model. 21.3% of the variation in input factors was
explained by other factor that is not included in the study (than the identified input factors by this study that affects the performance of the organizational).
Overall statistical significance of the regression model was examining by testing the null hypothesis that R = 0 and the regression coefficient is not significant. From the model significance = 0.000, the null hypothesis was rejected and it was concluded that there was a statistically significant positive causal relationship between input factors and organizational performance.
If the significance value of the F statistic is small (smaller than say 0.05) then the independent variables do a good job explaining the variation in the dependent variable. If the significance value of F is larger than say 0.05 then the independent variables do not explain the variation in the dependent variable, and the null hypothesis that all the population values for the regression coefficients are 0 is accepted. The null hypothesis is that there is no relationship, i.e., the beta coefficient is not different from
zero
Table 6 Model summery
Model
R
R2
Adjusted R2
Std. error of the estimate
Change statistics
1
.887a
.787
.776
.38412
R2 change
F change
df1
df2
Sig.F change
.787
75.919
7
144
.000
-
Predictors: (Constant), PRFM, EMFM, MFFM, MIFM, PTFM, LPF, PQFM
Table 6 gives the result of the analysis for the hypothesis. The first row between-groups variability, the second row the residual shows within groups gives variability due to random error and the third row gives the total variability. In the Table 6, F-value is 75.919 and the corresponding p value is given as < 0.000. Therefore, reject
the null hypothesis (H0) and conclude that the eight input factors have influence on organizational performance. Table 7 results shows that there is a significant relationship between the input factors and organizational performance, F (7, 144) = 75.919, p<0.001.
Table 7 Analysis of Variance (ANOVA)
Sum of squares
df
Mean square
F
Si.
Regression
78.411
7
9.843
75.919
0.000a
Residual
21.247
144
0.146
Total
99.657
151
-
a. Dependent Variable: OPFM
-
Predictors: (Constant), PRFM, EMFM, MFFM, MIFM, PTFM, LPF, PQFM
-
-
-
Hypothesis Testing
The regression analysis was used to test the developed hypothesis and investigate the contributions of the independent variables to the dependent variable in the model.
Hypothesis 1: There is a positive relationship between labor and personal factor and organizational performance. Figure 3 shows a significant contribution of labor and personal factors on organizational performance with (= 0.582, p=0.00).Therefore validating (confirming H1) Hypothesis 2: There is a positive relationship between market ingredients and organizational factors.
As show in figure 3 the coefficient of marketing ingredient has no statistical significant contribution on organizational performance ( = -0.083, p=0.875). This indicates that there was no statistical significant relationship between these variable
Hypothesis 3: There is a positive relationship between equipment and machinery factors and organizational performance.
As show in figure 3 the coefficient of equipment and machinery factors has no statistical significant contribution on organizational performance (= -0.022, p=0.680). This indicates that there was no statistical significant relationship between these variable
Hypothesis 4: There is a positive relationship between production technique and organizational performance.
As shown in figure 3 the coefficient of production technique (PT) has statistical significant contribution on
organizational performance (OP) with (=0.048, p=0.049).this indicates that there was statistical significant relationship between these variable. Therefore validating H4
Hypothesis5: There is a positive relationship between other factors and organizational performance.
Hypothesis6: There is a positive relationship between material factors and organizational performance.
As shown in figure 3 the coefficient of material factors (MF) has statistical significant contribution on organizational performance (OP) with (=0.154=0.00).This indicates that there was statistical significant relationship between these variable. Therefore validating H6 Hypothesis7: There is a positive relationship between product quality and organizational performance.
As shown in figure 3 The-e coefficient of product quality (PQ) has statistical significant contribution on organizational performance (OP) with ( = 0.111, p= 0
.026).This indicates that there was statistical significant relationship between these variable. Therefore validating H7
Hypothesis8: There is a positive relationship between productivity factors and organizational performance.
As shown in figure 3 the coefficient of productivity factor (PRF) has statistical significant contribution on organizational performance (OP) with (=0.327, p=0.000).This indicates that there was statistical significant relationship between these variable. Therefore validating H8
Fig.3 Shows models estimates consisting of path coefficients with their probability values
CONCLUSION
By taking the literature review and conceptual frame work developed, this study has identified factors that contribute to the performance of small scale manufacturing enterprise and then analyzed the key determinant factors that determine(affect)the performance the enterprise by testing the hypothesis, and the following conclusion were drawn.
The regression analysis showed that the performance of the manufacturing enterprise improved (increased) when there were more favorable conditions in the labor and personal factors (LPF), production techniques (PT), material factor (MF), productivity factor (PRF) and product quality (PQ). Correlation analysis shows the five independent variables( LPF, PT, MF, PRT and PQ) which have the direct effect on organizational performance of the enterprise, and the regression result also shows all these independent variables have significant and positive sign of coefficients the organizational performance (OP).
In this study, the key determinants of small and medium scale manufacturing enterprise can be explained by five of the eight factors that contribute for the performance of the enterprise. The factors on production techniques (PT) and equipment and material (EM) cannot contribute to the performance of the organization since they have weak correlation with the dependent variables. On the other hand the factors on other factors (OF) cannot be used to explain the performance of the firm in the study area as the responses from the respondent do not correlate with organizational performance of the firm.
ACKNOWLEDGEMENT
The authors would like to thank Ambo University, Ambo- Ethiopia for providing financial assistance in conducting the research.
REFERENCES
-
Mulhern D. Entrepreneurship and management.Journal of Small Business Management, 1995, vol. 25(3):92.
-
Federal Micro and Small Enterprise Development Agency (FMSEDA) Support Package For Metal and Wood Work Micro and Small Enterprises under the Micro and Small Enterprise Development Program. The Urban Development Package 2006
.Addis Ababa, Ethiopia
-
D.C Mead and Liedholm The Dynamics of Micro and Small Enterprises in Developing Countries. World Development, 1999, vol. 26(1) pp. 61.
-
F. W. Swierczek, and T. Ha Entrepreneurial orientation, uncertainty avoidance and firm performance: an analysis of Thai and Vietnamese SMEs. International Journal of Entrepreneurship and Innovation, 2003 vol. 4(1) pp.46-58.
-
G. Hall .Reasons for Insolvency amongst Small Firms- A Review and Fresh Evidence, Small Business Economics 1992 vol. 4(3) pp.237-250.
-
M. Roy and D. Wheeler, A Survey of Micro-Enterprise in Urban West Africa: Drivers Shaping the Sector. Development in Practice, 2006 vol. 16 pp. 452-464.
-
CLEP Entrepreneurship: Unpublished manuscript, 2006 Addis Ababa, Ethiopia
-
MUDC. Survey on Micro and Small Scale Enterprises in Selected Major cities of Ethiopia. 2013, Addis Ababa, Ethiopia.
-
S. Siva A Study on Problems Faced by Entrepreneurs of Small Scale Industries Asian Journal of Research in Social Science and Humanities, 2012, pp.181-188.
-
Admasu Abera Factors Affecting the Performance of Micro and Small Enterprises in Aradaand Lideta Sub-Cities, Addis Ababa
M.Sc. Thesis Addis Ababa University 2012
-
Endalkachew Desta Assessment on the Determinant Factors on the Performance of Micro and Small Enterprises: The Case of Hossana Town in Hadiya Zone M.Sc. Thesis 2016
-
Mokonnen and Tilaye Manufacturing in Micro-Enterprises in South Africa Report Submitted to the Industrial Strategy Project, 2013 University of Cape Town.
-
Workneh Fiseha. The Constraints of Micro and Small Scale Enterprises in Addressing Employment Opportunity: The case of KolfeKeraneo Sub-city, Addis Ababa M.A. Thesis in Regional and Local Development Studies. RLDS, 2007 Addis Ababa University.
-
Mulu Gebreeyesus Innovation and Micro-enterprises Growth in Ethiopia Word Institute for Development, Economic research, United Nations University, 2009, No. 51.
-
Zeleke Worku Efficiency in Management as a Determinant of Long-term Survival in Micro, Small and Medium enterprises in Ethiopia Problems and Perspectives in Management 2009 vol.7(3)
-
M. Saunders, P. Lewis and A. Thornhill Research methods for business students, 5th edn. 2009 Pearson, Harlow