
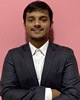
- Open Access
- Authors : Kaushal P Patil , Gregory Kapfhamme
- Paper ID : IJERTV11IS080140
- Volume & Issue : Volume 11, Issue 08 (August 2022)
- Published (First Online): 01-09-2022
- ISSN (Online) : 2278-0181
- Publisher Name : IJERT
- License:
This work is licensed under a Creative Commons Attribution 4.0 International License
Machine Learning Meets Sports
Data Analytics Demonstrating The Relationship Between Playing Surface & The Injury/Performance Of Athletes.
Kaushal P Patil
Delhi Public School-EAST Bangalore, Karnataka
Prof. Gregory Kapfhamme
(Research Guide)
Associate Professor of Computer Science College Allegheny College, University of Pittsburgh Meadville, Pennsylvania, United States
AbstractData about sports have long been the subject of research and analysis by sports scientists. The article takes deep dive into the positive impact of ML integration on sports analytics. This paper focuses on deploying the ML into the field of predicting sports injury. ML not only increases the knowledge of sports injury but also assist in proactively taking steps to avoid sports injury by predicting ahead of time. To this end, technological advancements have enabled the collection of multiple points of data for use in analysis and injury prediction. The full breadth of available data has, however, only recently begun to be explored using suitable statistical methods & processing of these large data through ML algorithms.
Paper focuses on sports injuries on Synthetic playing surfaces (artificial grass) compared that of Natural surfaces. It takes deep look at the prior work in this domain and opens some of the unanswered questions and shares importance of ML in analyzing the large sports data collected over a case study too.
Paper utilizes the advances in automatic and interactive data analysis with the help of machine learning algorithms & establishes the intricacies of the playing surface & injury relationship. Real time data shared by NFL for injured 250 players & 150 non-injured players, over two regular season is taken for case study. Data includes complex real time player data with player movement, injury type, and type of playing surface (synthetic Vs Natural), weather, player position, speed,
/acceleration of player, and so on. Data sports analytic competition is analyzed for the relationship between playing surface, NFL player's movements, and their damage, leading to potentially improved performance and minimizing the risk of injury. With this huge amount of data, the use of complex models for data analysis is mandatory and, for this reason, machine learning models are increasingly used in sports science. Article also briefly underlines the importance of critical sports parameters accurate data collection & direct impact on ML accuracy in prediction too.
KeywordsMachine learning, Sports Injury, Prediction, Lower Limb injury, NFL Sports, Foot bal injury, Artifical Playing Surface, Synthetic playing surface, Natural playing surface, Python Algoirthm for Machine learning, Data Analysis for sports, Sports Sicnce, Soccer injury.
-
INTRODUCTION
Artificial turf continues to alternate options that many players at the high school, intercollegiate, and professional levels practice and compete. There have been several studies and literature reviews to investigate the properties of artificial turf and their impact on injuries along with injury patterns too. The overall rate of football injuries has been reported to be
signicantly higher on articial surfaces compared with natural grass [56]. It is increasingly clear that the playing surface is an important factor in injury incidences and mechanisms.
John Powell from the University of Iowa [1] was among the first (1992) to quantify the higher incidence of these injuries, among the professional football teams with more major knee injuries on artificial turf than compared on natural grass.
Since that time, artificial turf companies have made significant strides to simulate more natural surfaces. Despite these modern advancements, the effect of artificial turf on injury rates is still controversial. Natural grass fields are not free from problems either. There are studies that demonstrate that playing on a grass surface that is not well maintained may also increase injury rates.
Study by JasonL Dragoo, [2] published in the National Library of Medicine concluded that college football players suffer about 1.39 times as many ACL tears when not playing on a natural surface.
Yet, according to other research, synthetic and natural surfaces lead to an equal number of orthopedic injuries. Gould H & team published a recent study in May 2022, which provided a comprehensive systematic review of sports injuries on artificial turf versus natural grass. A total of 53 articles published between 1972 and 2020 were identified for study inclusion. The study suggests that the rates of knee injuries and hip injuries are similar between playing surfaces, although elite-level football athletes may be more predisposed to knee injuries on artificial turf compared with natural grass. Only a few articles in the literature reported a higher overall injury rate on natural grass compared with artificial turf, and all of these studies received financial support from the artificial turf industry.
Rossi A, Pappalardo L, and Cintia describe very well the importance of the application of ML in sports. P [4]. With the technological advent of the last few decades, it is possible to record a huge quantity of data from athletes. Wearable devices, video analysis systems, tracking systems, and questionnaires are only a few examples of the devices used
currently to record data in sports. These data can be used for scouting, performance analysis, and tactical analysis, but an increased interest is in assessing the risk of injuries.
The National Football League is America's most popular sports league, comprised of 32 franchises that compete each year to win the Super Bowl, the world's biggest annual sporting event. Founded in 1920, the NFL developed the model for the successful modern sports league, including national and international distribution, extensive revenue sharing, competitive excellence, and strong franchises across the country.
The NFL is committed to advancing progress in the diagnosis, prevention and treatment of sports-related injuries on artificial surfaces. According to NFL, there are 12 stadiums with synthetic turf. Recent investigations by NFL showed the significantly higher lower limb injuries rate among football athletes on synthetic turf compared with natural turf (Mack et al., 2018; Loughran et al., 2019). Their epidemiologic investigations, biomechanical studies of football cleat-surface interactions have shown that synthetic turf surfaces do not release cleats as readily as natural turf and may contribute to the incidence of non-contact lower limb injuries (Kent et al., 2015). Given these differences in cleat-turf interactions, NFL has interest in determining, if player movement patterns and other measures of player performance differ across playing surfaces and how these may contribute to the incidence of lower limb injury.
This paper takes the real time data shared by NFL of each player over two seasons & implement ML algorithm to analyze the data & list the finding. Data shared by NFL, includes 250 players over two regular season schedules. One hundred of the athletes in the study data set sustained one or more injuries during the study period that were identified as a non-contact injury of a type that may have turf interaction as a contributing factor to injury. The remaining 150 athletes serve as a representative sample of the larger NFL population that did not sustain a non-contact lower-limb injury during the study period. Details of the surface type and environmental
parameters that may influence perfrmance and outcome are also provided. Real time data also covers parameters like, speed of players, and acceleration for every player, every play on every inch of the field and type of injuries and so on.
We have analyzed the data with Machine Learning algorithm to characterize any differences in player movement between the playing surfaces and identify specific scenarios (e.g., field surface, weather, position, play type, etc.) that interact with player movement to present an elevated risk of injury.
-
RESEARCH ANALYTICS:
Here Data shared by NFL is analyzed by applying the Machine Learning codes & also some the relevant plots/graphs is re-generated, along with news ones to demonstrate the benefits of the application of ML into injury prediction
Question1: Can ML (Machine Learning) establish the correlation between playing surface Vs Player injury.
FIG: 1
INJURIES OCCUR OVER 1.8x MORE ON SYNTHETIC FIELD
SYNTHETIC
SYNTHETIC
NATURAL
NATURAL
INJURY RATE (%)
After analyzing the data, it is clear that synthetic surfaces have a greater probability of injury. As seen in the FIG-1, the synthetic surfaces have approximately 1.8 times greater injury rate compared to natural surfaces. This establishes the correlation between Synthetic Surface & injury probability.
Question2: Can ML predict Injury on Synthetic being more severe than injury on the Natural surface. ?
FIG: 2
FIG:3
Percentage of higher numbers players injured in Artifical Turf each category comapre to Natural Turf
45%
40%
40% 39%
35%
30%
25%
20%
16%
15%
9%
10%
5%
0%
1 Day -Away from play 7 Days-Away from play 28 Days-Away from play 42 Days-Away from play
% of higher injury
Type of Injuries on Natural Turf
We analyze the data based on numbers of days that player has been away from play after the injury. Injured players are categorized in to four buckets based on number of days those players are away from play. These buckets are (a) Player being away from play for 1 Day (b) Players being away from play for 7 Days (c) Players being away from play for 28 days
(d) Players being ways from play for 42 days.
It clearly visible from Fig-2, that sheer numbers of injured players in each category is more in synthetic surface in comparison to natural turf. It is clearly evident from above Fig-3 that, as number of days away from play is more, higher is the percentage of players in the artificial surface over Natural surface. For example, we have seen 40% higher number of injured players in synthetic surface with more than 42 days away from play.
This clearly shows that more players are getting injured on Synthetic surface and also more importantly, the injuries appear to be more severe on synthetic surface. Which is leading to players to be away from play for longer numbers of days.
Question3: Can you differentiate the kind of Injury seen in Synthetic Vs Natural surface.
Type of Injuries on Artificial Turf
FIG-4 : Type of Injuries on Artificial Turf
FIG-5: Type of Injuries on Artificial Turf
We can see from the Fig-4, on the artificial surface, the foot or lower limb injuries are more often seen. This is evident in the box plot shown above. On artificial turf, the foot or lower limb is highest category of injures, then followed by Knee injuries. This outcome from ML application can be used by sports science to understand the injuries of particular kind in artificial surfaces.
On the contrary, it is evident from the Fig-5, on Natural surface, the foot or lower limb injuries are NOT as high as artificial surface. But there are Knee injuries on the Natural surface. Of course, these are not as high as foot injuries seen on the synthetic surfaces.
So we can conclude from above ML application that we often find lower limb or foot injury being seen more often on artificial surface compare to Natural surfaces.
Question4: Can ML establish the location of Injuries on Artificial Turf Vs Natural Turf.
Type of Injuries on Artificial Turf
FIG-6: Location of Injuries on Artificial Turf
Type of Injuries on Natural Turf
FIG-7: Location of Injuries on Natural Turf
It is evident from the Fig-6, the injuries on Synthetic turf, are more widely spread & across the location of Turf too. Comparison to Natural surface, the injuries are more concentrated on the center of Turf. This could also due to the speed that payers reach at the middle of turf. Usually, it is expected players reach higher speed at the middle of pitch.
It is important to mentioned one fact. Due to limitation of computer to analyze the data, limited numbers of sample were considered here. If the entire sample shared by NFL is considered, then the outcome can be more accurate & also may slightly vary too.
Question4: Any variation speed & distance between artificial Turf Vs Natural Turf.
FIG-7: Maximum Speed
FIG-8: Maximum distance
From the Fig-7 & Fig-8, we can observe the maximum speed is reached at the beginning of the turf length. This appears to be common observation between the Artificial & Natural turf. In other words, we do not find any difference in players reaching the maximum speed and appears to be same between both kind turfs. Same applies to maximum distances too.
-
RECOMMENDATIONS FOR FUTURE STUDY
It is clearly evident from the ML analysis, that Synthetic surface leads to (I) Higher injury rate (II) Higher Severity of injury based on number of days absent (III) injuries across the artificial turf (IV) more injuries to lower limbs and foot.
There is no difference in maximum speed & distance that players achieve between Artificial & Natural turf.
These finding perfectly match to study published by the American Journal of Sports Medicine, 2019,[8] Higher Rates of Lower Extremity Injury on Synthetic Turf Compared with Natural Turf Among National Football League Athletes Published in the American Journal of Sports Medicine, 2019
Since ML can handle large amount of Data, one of the possible recommendations to NFL to further improve & increase parameters sensitivity. NFL can also add variables like footwear, padding and even putting accelerometer/wearable device to measure physical parameters of athletes. Application of ML opens up the door of infinite opportunities to sports science.
A second phase study, we can now collect more data around lower-limb injuries, as seen more on artificial turf. This can further improve of assessment and accordingly one can work on the prevention of injuries by means various proactive measurements. This opens further study of injury preventions and sports training modules in-conjunction with ML technique
& applications.
Few Limitations: As shown above, we have used python algorithm of ML to analyze the data. Here accuracy of data is critical and also the more is the volume of data, better is prediction. We have shown in above case, results can slightly vary based on sample size & accuracy too. This is an important factor to consider in deploying the ML in sports or any other domain too.
-
CITATION REFERENCES:
ahead of print. PMID: 35593739
[4] Rossi A, Pappalardo L, Cintia P. A Narrative Review for a Machine Learning Application in Sports: An Example Based on InjuryForecasting in Soccer. Sports (Basel). 2021 Dec 24;10(1):5. doi: 10.3390/sports10010005. PMID: 35050970; PMCID: PMC8822889.
[5] [5] Gorse K, Mickey CA, Bierhals A. Conditioning injuries associated with articial turf in two preseason football training programs. J Athl Train Oct 1997;32(4):304-8 [6] Ramirez M, Schaffer KB, Shen H, Kashani S, Kraus JF. Injuries to high school foot-ball athletes in California. Am J Sports Med Jul 2006;34(7):1147-58. [7] Williams S, Hume PA, Kara S. A review of football injuries on third and fourth generation artificial turfs compared with natural turf. Sports Med. 2011 Nov 1;41(11):903-23. doi: 10.2165/11593190-000000000-00000. PMID: 21985213.
[8] Mack CD, Hershman EB, Anderson RB, Coughlin MJ, McNitt AS, Sendor RR, Kent RW. Higher Rates of Lower Extremity Injury on Synthetic Turf Compared With Natural Turf Among National Football League Athletes: Epidemiologic Confirmation of a Biomechanical Hypothesis. Am J Sports Med. 2019 Jan;47(1):189-196. doi: 10.1177/0363546518808499. Epub 2018 Nov 19. PMID: 30452873. [9] T1 – Comparison of the Incidence, Nature and Cause of Injuries Sustained on Grass and New Generation Artificial Turf by Male and Female Football Players. Part 1: Match Injuries. VL – 41 Suppl 1; DO– 10.1136/bjsm.2007.037267;JO – British journal of sports medicine [10] Hershman EB, Anderson R, Bergfeld JA, Bradley JP, Coughlin MJ,
Johnson RJ, Spindler KP, Wojtys E, Powell JW; National Football League Injury and Safety Panel. An analysis of specific lower extremity injury rates on grass and FieldTurf playing surfaces in National Football League Games: 2000-2009 seasons. Am J Sports Med. 2012 Oct;40(10):2200-5. doi: 10.1177/0363546512458888. Epub 2012 Sep 12. PMID: 22972855.
[11] Majumdar A, Bakirov R, Hodges D, Scott S, Rees T. Machine Learning for Understanding and Predicting Injuries in Football. Sports Med Open. 2022 Jun 7;8(1):73. doi: 10.1186/s40798-022-00465-4. PMID: 35670925; PMCID: PMC9174408. [12] Rein, R., Memmert, D. Big data and tactical analysis in elite soccer: future challenges and opportunities for sports science. SpringerPlus 5, 1410 (2016). https://doi.org/10.1186/s40064-016-3108-2
[13] Claudino, J.G., Capanema, D.d., de Souza, T.V. et al. Current Approaches to the Use of Artificial Intelligence for Injury Risk Assessment and Performance Prediction in Team Sports: a Systematic Review. Sports Med – Open 5, 28 (2019). https://doi.org/10.1186/s40798-019-0202-3 [14] Rommers N, Rössler R, Verhagen E, Vandecasteele F, Verstockt S, Vaeyens R, Lenoir M, D'Hondt E, Witvrouw E. A Machine Learning Approach to Assess Injury Risk in Elite Youth Football Players. Med Sci Sports Exerc. 2020 Aug;52(8):1745-1751. doi: 10.1249/MSS.0000000000002305. PMID: 32079917. [15] T1 – The Effect of Playing Surface on Injury Rate A Review of the Current Literature; VL – 40; DO – 10.2165/11535910-000000000-00000; JO – Sports medicine (Auckland, N.Z.)
[16] Fujitaka K, Taniguchi A, Kumai T, Otuki S, Okubo M, Tanaka Y. Effect of Changes in Artificial Turf on Sports Injuries in Male University Soccer Players. Orthop J Sports Med. 2017 Aug 2;5(8):2325967117719648. doi: 10.1177/2325967117719648. PMID:28812040; PMCID: PMC5544152.
[17] Butler RJ, Lehr ME, Fink ML, Kiesel KB, Plisky PJ. Dynamic Balance Performance and Noncontact Lower Extremity Injury in College Football Players: An Initial Study. Sports Health. 2013;5(5):417-422. doi:10.1177/1941738113498703 [18] [18] Naji S. Madi, Tejas J. Parekh, Selene G. Parekh,Outcome of Turf Toe Injuries in NFL Players, The Journal of Foot and Ankle Surgery, 2022,ISSN 1067-2516,https://doi.org/10.1053/j.jfas.2022.05.008.(https://www.sciencedirect.c om/science/article/pii/S1067251622001557)
[19] S. Landset, M. F. Bergeron and T. M. Khoshgoftaar, "Using Weather and Playing Surface to Predict the Occurrence of Injury in Major League Soccer Games: A Case Study," 2017 IEEE International Conference on Information Reuse and Integration (IRI), 2017, pp. 366- 371, doi: 10.1109/IRI.2017.86. [20] Dragoo, J.L., Braun, H.J. The Effect of Playing Surface on Injury Rate. Sports Med 40, 981990 (2010). https://doi.org/10.2165/11535910-000000000-00000 [21] Jason L. Dragoo, Hillary J. Braun, Alex H.S. Harris, Title The effect of playing surface on the incidence of ACL injuries in National Collegiate Athletic Association American Football, The Knee, Volume 20, Issue 3,2013, Pages 191-195, ISSN 0968- 0160,https://doi.org/10.1016/j.knee.2012.07.006. (https://www.sciencedirect.com/science/article/pii/S096801601200130 5) [22] Kalkhoven, J.T., Watsford, M.L., Coutts, A.J. et al. Training Load and Injury: Causal Pathways and Future Directions. Sports Med 51, 1137 1150 (2021). https://doi.org/10.1007/s40279-020-01413-6 [23] Orchard, J. Is There a Relationship Between Ground and Climatic Conditions and Injuries in Football?. Sports Med 32, 419432 (2002). https://doi.org/10.2165/00007256-200232070-00002