
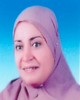
- Open Access
- [post-views]
- Authors : M. A. R. R. R. Husain , S. S. Mohamed
- Paper ID : IJERTV11IS010043
- Volume & Issue : Volume 11, Issue 01 (January 2022)
- Published (First Online): 19-01-2022
- ISSN (Online) : 2278-0181
- Publisher Name : IJERT
- License:
This work is licensed under a Creative Commons Attribution 4.0 International License
Modeling and Prediction of Impact Strength for AISI 304l and AISI 316l Stainless Steel Similar and Dissimilar Welded Joints using Regression Analysis and Artificial Neural Networks Approaches
-
A. R. R. R. Husain, S. S. Mohamed
Mechanical Engineering Department,
Faculty of Engineering at Shoubra, Benha University, Cairo, Egypt.
Abstract:- In the present investigation, the AISI 304L and AISI 316L austenitic stainless-steel (ASS) plates were welded together using gas tungsten arc welding (GTAW). Several similar butt joints, typically, 304L-304L and 316L-316L as well as dissimilar butt joints, typically, 304L-316L were formed. The dependance of the impact strength of the welded joints were modeled using regression modelling (RM) and artificial neural network (ANN) approaches. The impact energies of the welded joints were evaluated at ambient and lower temperatures up to -75 oC. The results revealed that, at low temperatures, reducing the temperature reduces the absorbed impact energy of the AISI 304L and 316L similar and dissimilar welded joints. The AISI 304L-316L dissimilar welded joints exhibited higher average impact energies when compared with the similar AISI 304L and AISI 316L welded joints. The generated ANN models based on Multi-Layer Perceptron (MLP) and Radial Basis Function (RBF) approaches can accurately predict the impact energies of the welded joints with a very high accuracy. The RBF and MLP ANN models having layers structure of 2-8-1 and 2-3-1, respectively, showed the best performance among all the investigated networks for predicting the impact energies of welded joints at different temperatures. The mean absolute error (MAE) resulted from the RBF and MLP ANN models are 4.84 and 4.60, respectively.
Keywords: Gas Tungsten Arc Welding, Stainless Steels, Impact Strength, Low Temperatures, Artificial Neural Networks.
-
INTRODUCTION
Austenitic stainless steels (ASSs) are probably the most used alloys of all the stainless steels (SS). ASSs are more resistant to corrosion due to the high content of chromium (18-20 wt.-%) and nickel (812 wt.-%) [1-3]. Generally, ASSs are non-magnetic and non-hardenable by heat treatment. However, they can be hardened by cold working. ASSs are widely used as a structural material in several industries, for example, the petrochemical, vehicle, and aviation industries [4].
Gas tungsten arc welding (GTAW) process has a wide range of applications in various industrial areas due to its capability of fabricating high-quality weld [5]. GTAW is one of the famous welding methods which using an arc between non- consumable electrode and workpiece metal to weld the sections. The broad applications of SSs for construction of equipment working in conditions of elevated as well as low temperatures, require methods for optimization, modelling, and predicting the mechanical properties of welded joints, which often affect the service life of the structure. Therefore, several approaches like the artificial neural network (ANN), machine learning (ML), regression analysis and other statistical and numerical approaches were used for such proposes [6,7].
An ANN is a form of application of an artificial intelligence (AI) system, which consisting of several interconnected processors or nodes, or process elements that are called artificial neurons [8,9]. It has been reported that the prediction results produced by ANN and ML models delivered significantly high relevance with the experimental data over the regression analysis [10]. It has been noticed that few investigations were reported on the modelling and prediction of the mechanical characteristics of ASSs welded joints, especially, as a function of temperature. Therefore, the present investigation aims to develop several models based on ANN and regression modelling (RM) methods to predict the mechanical characteristics of AISI 304L and 316L similar and dissimilar welded joints as a function of the temperature. To perform this task, a series of experiments were performed to collect the data about the impact energies of the welded joints at low temperatures up to -75 oC. The ASSs joints were welded using GTAW using constant process parameters.
-
EXPERIMENTAL WORK
In the present investigations, the AISI 304L and AISI 316L SS were used as base metals (BM). The chemical compositions of the AISI 304L and AISI 316L SS are listed in Table 1 and Table 2, respectively. Filler rods of type ER308L, with chemical compositions listed in Table 3, were used as filler material. The ER308L rods have 2.4 mm diameter. The plates form the AISI 304L and AISI 316L SS, with the dimensions of 60 mm (width) 600 mm (length) 10 mm (thickness), were machined to get single V-groove with an angle of 60o. Figure 1 shows a typical configuration for the welded joints.
Table 1. The chemical composition of AISI 304L ASS (wt.-%).
Base Alloy
Elements (wt.-%)
Cr
Ni
C
Mn
Si
P
S
Fe
AISI 304L
18.91
10.65
0.028
2.01
1.1
0.04
0.03
Bal.
Table 2. The chemical composition of AISI 316L ASS (wt.-%).
Base Alloy
Elements (wt.-%)
Cr
Ni
C
Mn
Si
Mo
P
S
Fe
AISI 316L
17.54
11.89
0.026
2.05
1.02
2.25
0.045
0.018
Bal.
Table 3. Chemical composition of the ER308L filler rods (wt.-%).
Filler rod
Elements (wt.-%)
C
Si
Mn
Ni
Cr
Mo
Cu
N
Fe
E308L
0.02
0.4
1.9
9.8
19.8
0.20
0.15
0.05
Bal.
Figure 1. The joint configuration of stainless-steel plates (dimensions in mm).
The similar welded joints (i.e., AISI 304L-304L and AISI 316L-306L) as well as the dissimilar welded joints (i.e., AISI 304L-316L) were joined using GTAW technique to form butt joints. The GTAW process was performed using Miller Syncrowave 250 CC-AC/DC welding machine. The welding voltage and current were kept constant at 38 V and 210 A, respectively. The GTAW process was carried out manually in four passes with an average welding speed of 11 mm/sec.
The impact energy of the AISI 304L-304L, AISI 304L-316L and AISI 316L-316L welded joints were measured. The Charpy impact specimens have 55 mm long and of square section with 10 mm sides, in the center of length, a V-notch of 45, 2 mm depth with a 0.25 mm radius of curve at the base of notch located at the weld centerline. Charpy impact tests were performed for welded joints at room as well as lower temperatures of -25, -50, and -75 °C. An ethanol alcohol-based cooling chamber was used to cool the welded SS specimens to the required temperatures. When the required temperature is reached, the impact welded specimen is directly subjecting o impact using computer-controlled pendulum impact tester. The absorbed energy (in Joules) was recorded. At each temperature, the impact tests are repeated, for each welding condition, for a minimum of three times and the mean value and the standard deviation of the impact energy is obtained.
The RM mathematical technique was used to correlate the impact energy of the welded specimens with both the temperature and the type of the base material. Moreover, the ANN technique was also used for modeling the influence of temperature and the base material type on the impact energy welded specimens. The ANN model was based on Multi-Layer Perceptron (MLP) as well as Radial Basis Function (RBF) neural networks. The mean absolute error (MAE) was calculated to define the accuracy of the generated ANN models. It is the difference between the measured value and true value. The MAE measures the average magnitude of the errors in a set of predictions, without considering their direction. The MAE can be calculated by using the following equation [11]:
(1)
The MAE is an average of the absolute errors , where yi is the prediction and xi the true (experimental) value.
-
RESULTS AND DISCUSSION
-
Impact Energy of the Stainless-Steel Welded Joints at Different Temperatures
Figure 2 shows the variation of the impact energy of the AISI 304L and AISI 316L SS welded joints with the temperature up to -75 oC. The results revealed that reducing the temperature reduces the impact energy of the welded joints. For instance, reducing the test temperature from ambient to -75 oC reduced the average impact energy of the AISI 304L similar welded joints from 185 J to 108 J, respectively. Similarly, for the AISI 304L-316L dissimilar welded joints, reducing the temperature from the ambient temperature to -75 oC, reduced the average impact energy from 188 J to 112 J, respectively.
Figure 2. The variation of impact energies of the stainless-steel welded joints with temperature.
The AISI 304L-316L dissimilar welded joints exhibited higher average impact energies when compared with the similar AISI 304L and AISI 316L welded joints. Such observation was noticed at all test temperatures. For instance, the average impact energies at ambient temperature were 185 J, 178 J and 188 J, for the AISI 304L, AISI 316L and AISI 304L-316L welded joints, respectively. While the average impact energies at -75 oC were found to be 108 J, 98 J and 112 J, for the AISI 304L, AISI 316L and AISI 304L-316L welded joints, respectively.
-
Regression Modelling of the Impact Energy of The Welded Joints
Figure 3 shows the regression modelling results for the impact energies (IE) of the similar AISI 304L and AISI 316L as well as and AISI 304L-316L dissimilar welded joints. The x-axis represents the temperature in Celsius and the y-axis represents IE in Joules. In Figure 3, the temperature dependence of IE can be approximated by equations (2), (3) and (4).
190
180
170
160
150
140
130
120
110
100
90
190
180
170
160
150
140
130
120
110
100
90
190
180
170
160
150
140
130
120
110
100
90
190
180
170
160
150
140
130
120
110
100
90
-80 -40 0
-80 -40 0
(a) (b)
200
190
180
170
160
150
140
130
120
110
200
190
180
170
160
150
140
130
120
110
-80 -40 0
(c)
Figure 3. Regression modelling results for the impact energy (IE) of the (a) 304L-304L, (b) 316L-316L and (c) 316L-304L ASSs welded joints; the x-axis represents the temperature in Celsius and the y-axis represents impact energy in Joules.
For AISI 304L similar joints:
(2)
For AISI 316L similar joints:
For AISI 304L-316L dissimilar joints:
(3)
(4)
Where: IE is the impact energy in Joules and t is the test temperature in Celsius. Equations 2, 3 and 4 have R-Square values of 0.95, 0.97 and 0.98, respectively.
The generalized equation that corelate the IE of the joint with both the temperature as well as the material to be welded (base materials) is shown below:
(5)
Where: t is the temperature in Celsius, x is the weld type and its equal to -1 for AISI 304L, +1 for AISI 316L, and 0 for AISI 316L-304L. Equation (5) has R-Square value of 0.977. Figure 4 shows the variation of the impact energy with temperature and the base material(s). In the Figure, the y-axis represents the temperature in Celsius and the x-axis represents joint type.
Figure 4. Regression modelling results for the impact energy (IE) of the AISI 304L-304L, AISI 316L-316L and AISI 316L-304L ASSs welded joints; the y-axis represents the temperature in Celsius and the x-axis represents joint type (-1 for AISI 304L, +1 for AISI AISI 316L, and 0 for AISI 316L-304L).
-
ANN Modelling of the Impact Energy of The Welded Joints
-
The RBF and MLP ANN models with a structure of 2-8-1 and 2-3-1, respectively, showed the best performance among all the investigated networks. The RBF ANN model showed training, test, and validation performances of 97.68%, 99.60 and 97.01%, respectively. Moreover, the MLP ANN model showed training, test, and validation performances of 97.68%, 99.82 and 96.20%, respectively.
Table 4 lists a comparison between the actual (Target) IE values of the welded joints and the output (predicted) IE values result from the generalized MLP and RBF models. Figure 5 shows a comparison between the target and the output IE values. The MAE resulted from the RBF and MLP ANN models are 4.84 and 4.60, respectively. The results demonstrate that the generated MLP model has a slightly better prediction than the RBF ANN model for predicting the impact energy of the AISI 304L and AISI 316L similar and dissimilar welded joints at different low temperatures. However, the accuracy of the RBF and MLP networks is still very high.
Table 4. The target and output IE values resulted from the generalized ANN modelling for the SS welded joints.
#
IE
Target
IE Output RBF 2-9-3
IE Output MLP 2-5-3
#
IE
Target
IE Output RBF 2-9-3
IE Output MLP 2-5-3
1
190.0000
186.6428
187.6725
14
149.0000
152.1444
145.2166
2
171.0000
166.3474
170.3196
15
138.0000
125.8291
132.4597
3
151.0000
139.8187
140.8097
16
103.0000
98.8422
101.5971
4
119.0000
110.7182
116.7105
17
178.0000
176.6684
178.5046
5
185.0000
186.6428
187.6725
18
145.0000
152.1444
145.2166
6
165.0000
166.3474
170.3196
19
132.0000
125.8291
132.4597
7
143.0000
139.8187
140.8097
20
98.0000
98.8422
101.5971
8
108.0000
110.7182
116.7105
21
172.0000
176.6684
178.5046
9
180.0000
186.6428
187.6725
22
141.0000
152.1444
145.2166
10
159.0000
166.3474
170.3196
23
126.0000
125.8291
132.4597
11
135.0000
139.8187
140.8097
24
93.0000
98.422
101.5971
12
97.0000
110.7182
116.7105
25
195.0000
185.2959
185.3065
13
184.0000
176.6684
178.5046
26
156.0000
154.5348
152.2285
Figure 5. Comparison between the target and the output of impact energy values resulted from the RBF and MLP ANN models.
-
-
CONCLUSIONS
-
Based on the results obtained from the present investigation, the following conclusions can be derived: –
-
Using the regression modelling approach, a mathematical equation was developed to correlate the relationship between the base materials and temperature with the impact energy of AISI 316L and 304L stainless steel similar and dissimilar welded joints. The equation has R-Square value of 0.977 which is very high.
-
The artificial neural network (ANN) models based on Multi-Layer Perceptron (MLP) and Radial Basis Function (RBF) techniques and with layer structures of 2-8-1 and 2-3-1, respectively, showed the best performance among all the investigated networks for predicting the impact energies of welded joints at different temperatures.
-
The RBF ANN model exhibited training, test, and validation performances of 97.68%, 99.60 and 97.01%, respectively. While the MLP ANN model showed training, test, and validation performances of 97.68%, 99.82 and 96.20%, respectively. The MAE resulted from the RBF and MLP ANN models are 4.84 and 4.60, respectively.
-
The developed ANN models based on the RBF and MLP approaches can accurately predict the impact energies of similar and dissimilar AISI 304L and AISI 316L stainless steel welded joints with a very high accuracy. These models can be used to find the dependance of the mechanical properties of the welded joints on temperature.
ACKNOWLEDGMENTS
The authors are thankful to the Benha University Faculty of Engineering at Shoubra for providing the facilities and equipment used in the present investigation.
REFERENCES
-
Kondapalli Siva Prasad, Chalamalasetti Srinivasa Rao, and Damera Nageswara Rao, A Review on Welding of AISI 304L Austenitic Stainless Steel, J. Manuf. Sci. Prod., 2014, 14(1), pp. 111.
-
C. Tembhurkar, R. Kataria, S. Ambade, J. Verma, A. Sharma and S. Sarkar, Effect of fillers and autogenous welding on dissimilar welded 316L Austenitic and 430 ferritic stainless steels, J. Mater. Eng. Perform., 2021, 30, pp. 110.
-
M. Poorkabirian, H. Mostaan and M. Rafiei, The effect of filler metal on properties of AISI 4130 to AISI 316L dissimilar joint, Journal of Advanced Materials in Engineering (Esteghlal), 2017, 36, pp. 3346.
-
Rafal M. Molak, Krystian Paradowski, Tomasz Brynk, Lukasz Ciupinski, Zbigniew Pakiela and Krzysztof J. Kurzydlowski, Measurement of mechanical properties in a 316L stainless steel welded joint, International Journal of Pressure Vessels and Piping, 2009, 86, pp. 4347.
-
M. P. Reddy, A. A. S. William, M. M. Prashanth, S. S. Kumar, K. D. Ramkumar, N. Arivazhagan and S. Narayanan, Assessment of mechanical properties of AISI 4140 and AISI 316 dissimilar weldments, Procedia Engineering, 2014, 75, pp. 2933.
-
I. Owunna and A. E. Ikpe, Modelling and Prediction of The Mechanical Properties of TIG Welded Joint for AISI 4130 Low Carbon Steel Plates Using Artificial Neural Network (ANN) Approach, Nigerian Journal of Technology (NIJOTECH), 2019, 38(1), pp. 117-126.
-
Ankita A. Kashid, Pallavi M. Patil, Monika R. Olekar, Priydarshani V. Deshmane, Sonali S. Jadkar, Analysis of Weld Joint for SS 316 Material Using Taguchi Technique, International Journal of New Technology and Research (IJNTR), 2019, 5(4), pp. 30-33.
-
Tapas Bera and Santanu Das, Application of Artificial Neural Networks in Predicting Output Parameters of Gas Metal Arc Welding of Dissimilar Steels, Indian Science Cruiser, 2021, 35(3), pp. 26-30.
-
Jeyaganesh Devaraj, Aiman Ziout and Jaber E. Abu Qudeiri, Grey-Based Taguchi Multiobjective Optimization and Artificial Intelligence-Based Prediction of Dissimilar Gas Metal Arc Welding Process Performance, Metals 2021, 11, 1858, pp. 1-20.
-
J. Xiong, G. Zhang, J. Hu, and L. Wu, Bead geometry prediction for robotic GMAW-based rapid manufacturing through a neural network and a second-order regression analysis, J. Intell. Manuf., 2014, 25, pp. 157163.
-
Yen-Hsiung Kiang, Model development and validation methodology, in Fuel Property Estimation and Combustion Process Characterization, ScienceDirect, 2018.
-