
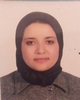
- Open Access
- Authors : Hanane Fikri , Taoufiq Fechtalil , Mohamed Mamoumi
- Paper ID : IJERTV10IS020065
- Volume & Issue : Volume 10, Issue 02 (February 2021)
- Published (First Online): 15-02-2021
- ISSN (Online) : 2278-0181
- Publisher Name : IJERT
- License:
This work is licensed under a Creative Commons Attribution 4.0 International License
Modeling of Activity of Cyclic Urea HIV-1 Protease Inhibitors using QSAR
Hanane Fikri, Taoufiq Fechtalil, And Mohamed Mamoumi
Laboratory of Neurosciences, Integrated Physiopathology and Natural SubstancesF.S.T. Faculty of Sciences and Technology, University Hassan II Casablanca, Mohammedia, Morocco
Abstract In this present work a Bayesian-Regularized artificial neural networks technique (BRNNs) was used of cyclic-urea derivatives, inhibiting HIV-1 protease. As a preliminary step, linear dependences were established by means a of multiple linear regression (MLR) approaches, selecting the relevant descriptors .The quality of the models was evaluated by means of cross-validation procedure and the best results correspond to nonlinear ones . The results are along the same lines as those of our previous studies on cyclic derivatives and indicate the importance of the hydrophobic parameter in and steric modelling the QSAR for cyclic urea derivativesis.
KeywordsComponent QSAR analysis; Backpropagation neural network analysis; Bayesian regularization , molecular descriptor, biological activity prediction, HIV-1 protease inhibitors.
-
INTRODUCTION
HIV-1 protease inhibitors (PIs) make a vital contribution on highly active antiretroviral therapy (HAART) of human immunodeficiency virus (HIV) (Tian et al., 2020)[1], and Several investigations suggest that there is a crucial need to develop a novel protease inhibitor with higher potency and reduced toxicity[2]. The PR is an enzyme which makes it possible the VIH[3]to be propagated. Like an apparatus of cut on a chain of production, the protease automatically divides the long chains of the VIH into smaller segments. They are these smaller sections of the virus which escapes to go to infect other cells CD4+.[4] The inhibitors of the protease disturb this process of cutting by the protease. Indeed, the IP block the way with the protease, a "sharp-edged chemical blade", so that the latter cannot divide the long chains of the VIH any more into more small parts which the VIH needs to reproduce. The long chains of VIH are unable to infect new cells CD4+ if they are not divided into shorter segments. Inhibitors of the viral protease prevent the maturation [5,6,7],of the viral particles, which removes their character infectious [8] A great number of inhibitors of protease, including the six made up ones which are the subject currently of clinical applications, act as peptidomimetic molecules, in which a bond replaces the peptide connection normally cleaved by the protease [9]. The addition of the inhibitors of protease to the range of the anti retrovirus treatments is at the origin of significant progress in the assumption of responsibility of the patients infected by the VIH. The large majority of treatments HAART (Highly Activates inert hydroxyethylene Anti Retroviral Therapy) include now these molecules [10]
Utilization of prior knowledge by application of computer- aided drug discovery approaches reduces time and financial expenses and increases the chances of positive results in anti- HIV R&D [11]. The development of new and safe anti-human
immunodeficiency virus (anti-HIV) drugs has been an urgent task for medical research recently. [12]. The quantitative structure-activity relationships (QSARs) procedures are certainly a major factor in contemporary drug design. Thus, it is quite clear why a large number of users of QSAR [13.14], it have been useful tools for the study of the PR mutants and their inhibitors. This NN method is therefore also complementary to previous studies on interaction of HIV-1 PR inhibitors with the target enzyme.
In this work, constitutional and 2D descriptors were used for encoding structural information from cyclic urea derivatives, and linear and non-linear models for their inhibition of HIV-1 protease (Ki,) were built using multivariate linear regression analysis (MLR) a new, robust structure-activity mapping technique, a Bayesian-regularized neural network, to develop a quantitative structure-activity relationships (QSAR) model, and the ability of the model was tested by using the cross validation technique. The results show that the method is robust and reliable and give good results. Comparisons of Bayesian neural net models with those derived by classical MLR model analysis showed its superiority in generalization.
-
RELATED WORK
The availability of computational techniques based on structureactivity relationships has accelerated the drug design process. Large databases of candidate inhibitors exist that have yet to be evaluated against HIV-1 protease or its resistant variants. The quantitative structure-activity relationships (QSARs) [11,12] procedures are certainly a major factor in contemporary drug design. Thus, it is quite clear why a large number of users of QSAR [13.14], it have been useful tools for the study of the PR mutants and their inhibitors. This NN method is therefore also complementary to previous studies on interaction of HIV-1 PR inhibitors with the target enzyme.
-
METHODOLOGY
-
Compounds Studied
The set of 68 cyclic urea derivatives PR inhibitors, then 40 symmetric and 28 nonsymmetric was compiled from literature [15-16-17-18-19-20-21-22-23] The criteria used for selection were: the level of inhibition constants Ki < 10 nM, and the variety of substituents to cyclic urea. Ki (nM) values represent the enzyme inhibition constants
Figure 1.General structures of cyclic-urea derivatives studied R1, R2 ,R3 and R4 : substituents cyclic-urea
log (1/ki) observed values were used as dependant variable in the biological activity Ki is the HIV protease enzyme inhibition constantHIV-1.
-
Molecular descriptors
In this study a set of descriptors related to physicochemical and geometric properties of the molecules was used. In order to study their influence on the in vitro activity of these compounds. All these descriptors were calculated for the separate substituents R1, R2 ,R3 and R4. Molecular properties used for each substituent, were:
-
LogP: logarithm of the partition coefficient between the water and the octanol of the molecule.
-
S: surface of the molecule
-
V: volume of the molecule
-
Hydrogen-bonding donors (HBD) and hydrogen-bonding acceptors (HBA).
-
Electronegativity.
-
MR: molecular reactivity
-
MW: Molecular weight
-
Ov: Ovality estimation
These descriptors were calculated for the individual substituents and molecular structure. All these descriptors were calculated with molecular modelling program (Mmp) demo version [24].
III.3. Statistical Analysis
III.3.1 Regression Analysis
MLR [25] analysis with stepwise selection and elimination of variables was employed to model the structure-activity relationships with different set of descriptors. In order to avoid all difficulties in the interpretation of the resulting models, pairs of variables with a correlation coefficient superior to
0.80 were classified as intercorrelated, and only one of these was included in the screened model. The quality of the model was considered as statistically satisfactory on the basis of squared correlation coefficient (r2), standard deviation (s), and F-statistics (F) when all parameters in the model were significant at 95% confidence level (p<0.05).
To remain close to the experimental error (5%), we take away the molecules having : Di > 2s/ the mean of observed activity where Di = |Obsi Cali | /Obsi. Consequently, a nw regressions models was established.
III.3.2. ARTIFICIAL NEURAL NETWORKS
As biological phenomena are considered non-linear by nature, ANN technique was applied in order to discover the possible existence of non-linear relationships between activity and molecular descriptors that are ignored for the linear approach.
Bayesian regularization overcomes the remaining deficiencies of neural networks. Bayesian methods produce models that are robust and able to comprise complex relations. No test or validation sets are involved so that all available training data can be devoted to the model and the potentially lengthy validation process can be avoided. At the end of training, ANN has optimal generalization qualities. The Bayesian neural net has the potential to give models which are relatively independent of neural network architecture, above a minimum architecture, and the Bayesian regularization method estimates the number of effective parameters.
-
-
RESULTS AND DISCUSSION
In this work we used centered and reduced values [26], of relevant descriptors selected, in order to have homogeneity in our data.
The QSAR multiple linear regression analyses were executed with the STATIGRAPHICS Program[27].
Our Bayesian regularized ANN are classical back-propagation neural nets that incorporate the Bayesian regularization algorithm for finding the optimum weights. The Bayesian regularization implemented in Matlab environment[28].
Table 1: comparison of the quality of the MLR and BRNN of cyclic- urea
Symetric
Non Symetric
Symetric+ Non Symetric
MLR
BRNN
MLR
BRNN
MLR
BRNN
Data
40
28
68
Selected
LogP(R1)
logP(R2)
log(R2)
descriptors
LogP(R2)
MW(R3)
MW(R3)
MW(R2)
MW(R4)
logP(R3)
r
0,86
0.962
0.89
0.985
0.86
0.983
s
0,447
0.23
0,2066
0.074
0,34728
°0.1151
F
32.12
461.4
28.67
823.324
173.04
1179.57
(r) corrélation Coefficient , (s) standard deviation and (F) F-statistics
The comparison of the quality of the MLR and BRNN models shows that the BRNN have substantially better predictive capabilities.
The standard errors of calculation are lower and the correlation coefficients are higher with ANN than with regression analysis. This preliminary study enables us to conclude that all the NN architectures were able to establish a satisfactory relationship between the molecular descriptors and the anti-HIV-1 activity.( The plot in Figure.1 ).
Figure 2: Observed (closed circles) and predicted (open circles) activity values with the BRNN and MLR models.
-
CONCLUSION
Multiple regression analysis is employed in such studies in the hope that it capacity point to structural factors that influence a particular property
This study confirms that Ki values can be predicted on the basis of simplest molecular structure information. The BRNN models can be applied to prediction of inhibitory activities of compounds that are not present in data set used in this study as long as they are structurally similar.
The development of non-linear QSAR combined with Bayesian Regularized search can lead to more predictive models; therefore, a more accurate structureactivity relation can be obtained
The results obtained by BRNN method for QSAR indicate that the BRNN was able to establish a satisfactory relationship between the molecular descriptors and the anti-HIV activity.
REFERENCES
-
Tian, Y., Zhang, S., Yin, H., & Yan, A. (2020). Quantitative structure- activity relationship (QSAR) models and their applicability domain analysis on HIV-1 protease inhibitors by machine learning methods. Chemometrics and Intelligent Laboratory Systems, 196, 103888.
-
Bhargava, S., Adhikari, N., Amin, S. A., Das, K., Gayen, S., & Jha, T. (2017). Hydroxyethylamine derivatives as HIV-1 protease inhibitors: A predictive QSAR modelling study based on Monte Carlo optimization. SAR and QSAR in Environmental Research, 28(12), 973990.
-
arré-Sinoussi, F.; J.C. Chermann; F. Rey; M.T. Nugeyre; S. Chamaret;
J. Gruest; C. Dauguet; C. Axler-Blin; F. Brun-Vezinet; C. Rozioux; W. Rozenbaum; L. Montagnier, Isolation of a T-lymphotropic retrovirus from a patient at risk for acquired immunodeficiency syndrome (AIDS). Science, 1983. 220: p. 869-871.
-
Alimonti, J.B.; T.B. Ball; K.R. Fowke, Mechanisms of CD4+ T lymphocyte cell death in human immunodeficiency virus infection and AIDS. Journal of general Virology, 2003. 84: p. 1649-1661.
-
Louis, J.M.; N.T. Nashed; K.D. Parris; A.R. Kimmel; D.M. Jerina, Kinectics and mechanism of autoprocessing of human immunodeficiency virus type 1 protease from an analog of the Gag-Pol polyprotein. Proc Natl Acad Sci U S A., 1994. 91: p. 7970-7974.
-
Lapatto, R., Blundell, T., Hemmings, A., Overington, J., Wilderspin, A., Wood, S., Merson, J.R., Whittle, P.J., Danley, D.E., and Geoghegan, K.F. X-ray analysis of HIV-1 proteinase at 2.7 resolution confirms structural homology among retroviral enzymes. Nature 1989, 342(6247), 299-302.
-
Wlodawer, A., and Vondrasek, J. Inhibitors of HIV-1 protease: a major success of structure-assisted drug design. Annu. Rev. Biophys. Biomol. Struct. 1998, 27, 249-284.
-
Abu-Ata, O.; J. Slim; G. Perez; S.M. Smith, HIV Therapeutics : Past, present, and future.,in HIV-1 : Molecular biology and pathogenesis. 2000, Academic Press. p. 1-40.
-
Lebon, F.; M. Ledecq, Approaches to the design of effective HIV-1 protease inhibitors. Current Medicinal Chemistry, 2000. 7(4): p. 455- 477.
-
Holodniy, M.; V. Miller, Human immunodeficiency virus, in Practical guidelines in antiviral therapy, C.A.B.B.G.J. Galasso, Editor. 2002, Elsevier Science. p. 95-125.
-
Stolbov, L., Druzhilovskiy, D., Rudik, A., Filimonov, D., Poroikov, V., & Nicklaus, M. (2020). AntiHIV-Pred: Web-resource for in silico prediction of anti-HIV/AIDS activity. Bioinformatics, 36(3), 978979.
-
Wang, Y., Yan, F., Jia, Q., Dai, Y., & Wang, Q. (2017). Quantitative structure-activity relationship of anti-HIV integrase and reverse transcriptase inhibitors using norm indexes. SAR and QSAR in Environmental Research, 28(12), 10251044.
-
Topliss, J.G., and Edwards, R.P. Chance Factors in Studies of Quantitative Structure Activity Relationships. J. Med. Chem. 1979, 22, 1238-1244.
-
11 Bazoui, H., Zahouily, M., Boulaajaj, S., Sebti, S., Zakarya, D, QSAR for anti-HIV activity of HEPT derivatives. SAR QSAR Environ. Res. 2002, 13, 567-577.
-
Garg, R.; Gupta, S. P.; Gao, H.; Mekapati, S. B.; Debnath, A. K.;
Hansch, C. Chem. Rev. 1999, 99, 3525
-
Gupta, S. P.; Babu, M. S.; Garg, R.; Sowmya, S. J. Enzym. Inhib. 1998, 13, 399.
-
Gupta, S. P.; Babu, M. S.; Kaw, N. J. Enzym. Inhib. 1999, 14, 109.
-
Gupta, S. P.; Babu, M. S.; Sowmya, S. Bioorg. Med. Chem. 1998, 6, 2185.
-
Gayathri, P.; Pande, V.; Sivakumar, R.; Gupta, S. P. Bioorg. Med. Chem. 2001, 9, 3059.
-
Debnath, A.K. J. Chem. Inf. Comput. Sci. 1998, 38, 761.
-
Kurup, A.; Mekapati, S. B.; Garg, R.; Hansch, C. Curr. Med. Chem. 2003, 10, 1819.
-
R. Garg, B. Bhhatarai / Bioorg. Med. Chem. 12 (2004) 58195831
-
MMP, molecular modelling pro-Demo (TM) Revision 301 demo. ChemSW Software (TM)
-
STATGRAPHICS Plus for Windows
-
Bazoui H, Zahouily M, Boulaajaj S, Sebti S, Zakarya D (2002) 401SAR and QSAR in Environmental Research 13:567577
-
Neural network toolbox for use with MATLAB, Howard Demuth, Mark Beale, The MathWorks, Inc, September 2001