
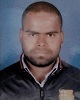
- Open Access
- Authors : Abhishek , Niranjan Sorout
- Paper ID : IJERTV9IS040213
- Volume & Issue : Volume 09, Issue 04 (April 2020)
- Published (First Online): 14-04-2020
- ISSN (Online) : 2278-0181
- Publisher Name : IJERT
- License:
This work is licensed under a Creative Commons Attribution 4.0 International License
Multi Objective Optimization of Process Parameters on MRR and TWR in EDM for EN 353 Material using Grey-Taguchi Method
Abhishek Dept. Of Mechanical Engg. Rattan Institute of Technology and Management Palwal, India |
Niranjan Sorout Dept. Of Mechanical Engg. Rattan Institute of Technology and Management Palwal, India |
AbstractThe use of the Taguchi method with grey relational analysis to establish the process parameters in electro-discharge machining of EN 353 alloy steel with selected tool electrode material . Throughout the study, multiple quality characteristics like tool wear rate (TWR) & metal removal rate (MRR) are focused. The effort has been made to minimize tool wear rate and maximize metal removal rate to produce high-quality finishing of the die. The process parameters, namely Peak Current, Pulse on time, Different tool materials are optimized with respect to tool wear rate and metal removal rate, using the Taguchi quality design concept, an L9 Orthogonal Array table is chosen for the experiments. From the grey analysis, a grey relational grade is obtained and based on this value. An optimum level of process parameters has been identified. Furthermore, using analysis of variance method, significant contributions of process parameters have been determined. The results of the confirmation experiment show that the optimal process parameters may be determined successfully so as to perk up numerous quality characteristics (TWR & MRR) through this approach.
KeywordsEDM, Electrode, Pulse on Time, MRR, TWR
-
INTRODUCTION
Electrical Discharge Machining Process, which is also called electro-erosion machining process. When the gap voltage is sufficiently large (i.e. reaches the breakdown voltage of dielectric fluid), high power spark is produced, which increase the temperature about 10,000 degrees Celsius. By this way the metal is removed from the work piece [1].A major advantage of EDM is that the tool and the work piece do not come into contact [2]. In this process material is removed by controlled erosion through a series of electric sparks between the tool (electrode) and the work piece. The thermal energy of the sparks leads to intense heat conditions on the work piece causing melting and vaporizing of work piece material. Due to the high temperature of the sparks, not only work material is melted and vaporized, but the electrode material is also melted and vaporized, which is known as electrode wear (EW). The EW process is quite similar to the material removal mechanism as the electrode and the work piece are considered as a set of electrodes in EDM. Due to this wear, electrodes lose their dimensions resulting in inaccuracy of the cavities formed. During EDM, the main output parameters are the material removal rate (MRR) and
Tool wear ratio (TWR). Electric discharge machining (EDM) is a non- traditional machining operating procedure in the manufacture of complex shaped dies, moulds and scathing parts used in automobile, aerospace, surgical and other industrial applications [3] Electrical-discharge machining (EDM) is extensively used for high strength materials. An analysis on the influence of current, pulse duration and duty cycle over MRR and TWR was performed. EDM is now unquestionably recognized as an important precision machine tool forming process for producing internal shapes on work piece. This study presents experimental analysis based on three factors and three levels Orthogonal Array L9 design. The objective of this research is to study the performance of different electrode materials on EN 353 work piece with EDM process.
EN 353 is a high feature alloy steel giving good ductility and shock resisting properties collective with resistance to wear. This steel is mostly known as bearing steel and used for bearing production in industrial sector.EN 353 steel is chiefly famous as bearing steel. This is used for roller bearing components such as 1) Brakes 2) Cylinders 3) Conical and Needle rollers.
Studies show that selection of process parameters and fixing the appropriate range of parameters to machine every product decides the quality of the product and in turn the processes requirements.Vikas et.al has done EDM process optimization with multiple performance characteristics based on orthogonal array with grey relational analysis for EN-41 with copper electrode [4]. ShaileshDewanganet.al studied the process of EDM with cylindrical shaped graphite tool electrode and AISI P20 work-piece for obtaining favorable preference measures in surface integrity (surface crack density, white layer thickness and surface roughness) using PCA based grey relational analysis has been selected[5].The original Taguchi method was designed to optimize only a single performance characteristic whereas The Grey relational analysis has been proved to effectively resolve the complicated interrelationships among multiple performance characteristics of the EDM process .
The goal of the present study was to determine the optimal machining parameters for maximum MRR and minimum TWR under given machining conditions. The Taguchi method was employed to explain the effect of the machining
parameters on the characteristics of the EDM process. Additionally, Grey relational analysis was used to find the optimal machining parameters satisfying the multiple characteristics of the EDM process.
-
EXPERIMENTAL DETAILS
-
Tool material
In EDM electrode material plays a significant role. During study, the electrode material is measured as a variable. In the Present study, three different electrodes are used as tool material for machining. The electrode materials used are brass, aluminum and copper.
-
Work material
In the present study the work material EN 353 is used whose hardness is 62-64 HRC and fig.1.Shows that the work piece before and after the machining.
Fig.1.Work Piece Before and After Machining
-
-
RESERCH METHODOLOGY
The selection of optimum machining parameters in EDM is an important step. Taguchi Optimization technique is single parameter optimization based on the signal to noise ratio [8]. Grey relational analysis is applied to optimize the parameters having multi-responses through grey relational grade. Furthermore, a statistical analysis of variance (ANOVA) is performed to see which process parameters are statistically significant. The steps involved are
-
Selection of process parameters and responce
The process parameters and levels chosen for the experiments are shown in the Table I. These parameters are so selected because of the prominent impact on the performance and at the same time may be controllable individually and effectively.
Table I
Level values of input Factors
Control Factors
1
level
2
level
3
level
Peak Current(Ip),amp
5
10
15
Pulse on time (Ton) µsec.
50
100
150
Tool Material
Brass
Al
Cu
-
Design of experiment
Experiments are performed, randomly, according to the L9 orthogonal array generated by minitab-17 software, on EN
353. For each experiment a separate electrode is used. The machining time is 10 minutes for all experiments. The machining time is noted from the timer of the machine. The eletrode wear rate is calculated by weight difference of the
electrodes using automatic weighing machine with 300g capacity with a precision of 10mg. The experiments along with the details of the values of various parameters for evaluating TWR, MRR based on L9 orthogonal array are shown in Table II
Table II Design of Experiment
S.NO.
Peak Current(IP)
Electrode Material
Pulse on Time(TON)
1
5
Brass
50
2
10
Copper
100
3
15
Aluminum
150
4
5
Aluminum
100
5
10
Brass
150
6
15
Copper
50
7
5
Copper
150
8
10
Aluminum
50
9
15
Brass
100
-
-
RESULT AND DISCUSSION
-
Observation And Calculation for MRR and TWR
After the experimental procedure, different response factors like MRR, TWR calculated from the observed data in Table
-
Statistical analyses were performed on the calculated values and the Where is the value of the response, S.NO is the experiment condition with no.1, 2, and 3 …n
Table III
Values for MRR and TWR after the experiment
S.NO.
MRR(g/min)
TWR(g/min)
1
0.087
0.0683
2
0.299
0.0343
3
0.022
0.0193
4
0.121
0.0263
5
0.101
0.1683
6
0.379
0.0243
7
0.43
0.0213
8
0.123
0.0343
9
0.184
0.1823
-
-
Calculation for Normalized value of S/N ratio
In this step of the grey relational analysis, pre-processing of the data was first performed for normalizing the raw data for analysis. This is shown in Table-IV, Yi, Xi is normalized by the following formula to avoid the effect of adopting different units and to reduce the variability. The normalized output parameter (MRR) corresponding to the larger-the-better criterion can be expressed by equation-1and 2.
xi(k)minxi(k)
Xi(k)=
maxxi(k)minxi(k)
(1)
Then for the output parameters (TWR), which follow the lower-the-better criterion can be expressed as
Y (k)= maxyi(k)yi(k)
i maxyi(k)minyi(k)
(2)
Where
xi (k),Xi(k)&yi(k),Yi(k) are the sequence after the data preprocessing and comparability sequence respectively, k=1; i=1, 2, 3, 9 for experiment numbers 1 to 9.
Table IV
Normalized Value for MRR and TWR
Sr.No
Normalized MRR(xi)
Normalized TWR(yi)
1
0.1593
0.6983
2
0.6789
0.9087
3
0.0000
1.0000
4
0.2426
0.9537
5
0.1936
0.0765
6
0.8750
0.9704
7
1.0000
0.9870
8
0.2475
0.9068
9
0.3971
0.0000
-
Calculation for deviation sequence
oi=x *(k) x *(k)
o i
(3)
oi=x *(k) x *(k)
o i
(3)
The grey relational coefficient is calculated to express the relationship between the ideal (best) and actual normalized experimental results. Before that the deviation sequence for the reference and comparability sequence were found out. These are given in Table V and grey relational coefficient is given in Table VI the deviation sequence can be expressed by equation-3.
Where
o
o
i
i
oi is the deviation sequence of the reference sequence x *(k) and the comparability sequence x *(k) and comparability value is taken as 1 for all the experiments
Table V
S.NO.
oi(MRR)
oi(TWR)
1
0.8407
0.3006
2
0.3211
0.0920
3
1.0000
0.0000
4
0.7574
0.0429
5
0.8064
0.9141
6
0.1250
0.0307
7
0.0000
0.0123
8
0.7525
0.0920
9
0.6029
1.0000
S.NO.
oi(MRR)
oi(TWR)
1
0.8407
0.3006
2
0.3211
0.0920
3
1.0000
0.0000
4
0.7574
0.0429
5
0.8064
0.9141
6
0.1250
0.0307
7
0.0000
0.0123
8
0.7525
0.0920
9
0.6029
1.0000
Deviation sequence for MRR and TWR
-
Calculation for grey relational coefficient and grade
(k) = ( minmax ) i oi(k)max
(4)
(k) = ( minmax ) i oi(k)max
(4)
After data pre-processing is carried out, a grey relational coefficient can be calculated with the pre-processed sequence. It expresses the relationship between the ideal and actual normalized experimental results. The grey relational coefficient is defined as follows by equation-4.
Where
i
i
oi(k) is the deviation sequence of the reference sequence x*(k) and the comparability sequence is x *(k), distinguishing or identification coefficient. If all the parameters are given equal preference &taken as 0.5.The grey relational coefficient for each experiment of the L9 OA can be calculated using equation and same is expressed in Table VI. After obtaining the grey relational coefficient, the grey
relational grade is computed by averaging the grey relational coefficient corresponding to each performance Characteristic. The overall evaluation of the multiple performance characteristics is based on the grey relational grade that is given by euation-5.
Table VI
Grey Relational Coefficient and Grade
EX.NO
GRCMRR
GRCTW
Grey Relational Grade
MRR
TWR
i= (iMRR + itwr)
Rank
1
0.3729
0.6245
0.4987
7
2
0.6090
0.8446
0.7268
3
3
0.3333
1.0000
0.6667
4
4
0.3977
0.9209
0.6593
5
5
0.3827
0.3536
0.3682
9
6
0.8000
0.9422
0.8711
2
7
1.0000
0.9760
0.9880
1
8
0.3992
0.8446
0.6219
6
9
0.4533
0.3333
0.3933
8
i= (iMRR + iTWR)
(5)
Where
ith the grey relational grade for the ith experiment and n is the number of performance characteristics which is taken as 2 for MRR and TWR, Table VI shows the grey relational grade for each experiment using L9 OA. The higher grey relational grade represents that the corresponding experimental results is closer to the ideally normalized value. Experiment 7 has been multiple performance characteristics among nine experiments because it has the highest grey relational grade. It can be seen that in the present study, the optimization of the complicated multiple performance characteristics of EDM of EN 353 has been converted into optimization of a grey relational grade.
-
Calculation for Main effect and ANOVA
Since the experimental design is orthogonal, it is then possible to separate out the effect of each machining parameter on the grey relational grade at different levels 1, 2 and 3 can be calculated by averaging the grey relational grade for the experiment 1to 3, 4 to 6 and 7 to 9 as respectively as shown in Table VIII
The mean of the grey relational grade for each level of the other machining parameters namely Duty cycle, peak current, pulse on time and tool material can be computed in the same manner. The mean of the grey relational grade for each level of the machining parameters is summarized and shown in Table VII. In addition, the total mean of the grey relational grade for the nine experiments is also calculated and presented in Fig.2. Shows the grey relational grade obtained for different process parameters. The mean of grey relational grade for each parameter is shown by horizontal line. Basically, the larger the grey relation grade is, the closer will be the product quality to the ideal value. Thus, larger grey relational grade is desired for optimum performance. Therefore, the optimal parameters setting for better MRR and lesser TWR are (A1B1C3) as presented in Table VII. Optimal level of the process parameters is the level with the highest grey relational grade
Table VII
Response table for grey relational grade
Level |
Peak Current (A) |
Pulse On Time (B) |
Tool Material(C) |
1 |
-3.255* |
-3.789* |
-3.755 |
2 |
-5.192 |
-4.832 |
-7.609 |
3 |
-4.275 |
-4.102 |
-1.358* |
Delta |
1.9370 |
1.043 |
6.251 |
RANK |
2 |
3 |
1 |
*levels of the optimum grey relational grade-A1B1C3 |
Table VIII
Analysis Of Variance for Grey Relational Grade
Source |
Df |
SeqSs |
F |
P |
% Contribution |
IP |
2 |
5.63 |
18.82 |
0.05 |
8.4 |
TON |
2 |
1.71 |
5.74 |
0.14 |
2.5 |
Material |
2 |
59.66 |
199.3 |
0.005 |
89.0 |
Error |
2 |
0.29 |
0.4 |
||
Total |
8 |
67.31 |
100% |
Percent Contribution
Percent Contribution
0% 8%
3%
0% 8%
3%
CURRENT
T-ON MATERIAL
Residual Error
CURRENT
T-ON MATERIAL
Residual Error
89%
89%
Fig.2.Percentage Contribution of Parameters
Furthermore, ANOVA has been performed on grey relational grade to obtain contribution of each process parameter affecting the two process characteristics jointly and is discussed in the forthcoming section. ANOVA for grey relational grade is presented in Table VIII. Percentage contributions for each term affecting grey relational grade are shown in Fig.3, Fig.3 clearly shows that tool material is the dominant parameter that affects grey relational grade and hence contributes in improving MRR and reducing TWR It can be seen from Figures 2 and 3 that current is the most significant factor that affects the grey relational grade. Metal removal is directly proportional to the amount of energy applied by the current.
Fig.3. Main Effect Plot
-
CONFIRMATION TEST
Confirmation test has been carried out to verify the improvement of performance characteristics (MRR & TWR) in EN 353 using EDM the optimum parameters are selected for confirmation test as represent in Table IX
Table IX
Theoretical and grey optimal level
Optimal machining parameters
Machining parameters in seventh trial of OA
Grey theory prediction design
Level
A1B3C3
A1B1C3
MRR
0.430
0.4863
TWR
0.0213
0.0158
Improvement in MRR = 0.0563 g/min (approx.13.09%) Improvement in TWR = 0.0055 g/min (approx.25.82%)
Table X which shows the comparison of the experimental results using the initial Orthogonal ArrayA1B3C3and optimal (grey theory prediction design, A1B1C3) machining parameters. Based on Table, MRR is accelerated from 0.430 to 0.4863 g/min; the TWR is decreased from 0.0213 to 0.0158 g/min. The corresponding improvements in MRR and TWR are 13.09 % and 25.82% respectively. It is clearly shown that the multiple performance characteristics in the EDM process are greatly enhanced through this study
-
CONCLUSION
The GRA based on the Taguchi methods response table has been proposed as a way of studying the optimization of EDM process parameters for EN 353. The optimal machining parameters have been determined by the grey relational grade for Multi performance characteristics that are MRR and TWR.
Nine experimental runs based on OAs have been performed. The following conclusions can be drawn from this study.
-
The work has successfully evaluated the feasibility of EDM of EN 353.
-
From the response table IX of the average grey relational grade, it is found that the largest value of grey relational grade for Tool material, peak current and pulse on time are Copper, 5A and 50µs, respectively. These are the recommended levels of contrllable process factors when better MRR and lesser TWR simultaneously obtained.
-
The ANOVA of grey relational grade for multi- performance characteristics reveals that the Tool material and peak current are the most significant parameter.
-
Based on the confirmation test, the improvements in MRR and TWR are 13.09% and 25.82% respectively.
It is shown that the performance characteristics of the EDM process such as MRR and TWR are improved together by using the method proposed by this study. The effectiveness of this approach has been successfully established by validation experiment.
REFERENCE
[1]. M. Durairaj, D. Sudharsun, N. Swamynathan, Analysis of Process Parameters in Wire EDM with Stainless Steel using Single Objective Taguchi Method and Multi Objective Grey Relational Grade, International Conference On DESIGN AND MANUFACTURING, IConDM 2013,Procedia Engineering 64 ( 2013 ) 868 877. [2]. Y. H. Guu& H. Hocheng, Effects of Work piece Rotation on Machinability during Electrical Discharge, Machining materials And Manufacturing Processes (2001) 16 (1), 91101. [3]. M Manohara, T Selvarajb, D Sivakumara, Experimental study to assess the effect of Electrode bottom profiles while machining Inconel 718 through EDM Process,Procedia Materials Science 6 (2014), 92-104. [4]. Vikas, Apurba Kumar Roy, Kaushik Kumar, Effect and Optimization of various Machine Process Parameters on the Surface Roughness in EDM for an EN41 Material using Grey- Taguchi, 3rd International Conference on Materials Processing and Characterization (ICMPC 2014), Procedia Materials Science 6 ( 2014 ) 383 390. [5]. ShaileshDewangan,Chandan Kumar Biswas and SoumyaGangopadhyay, Optimization of the Surface Integrity Characteristics of EDM Process using PCA based Grey Relation Investigation, 3rd International Conference on Materials Processing and Characterization (ICMPC 2014), Procedia Materials Science 6 (2014) 1091 1096. [6]. Hitesh B Prajapati, V. A. Patel, Hiren. R. Prajapati, Experimental Investigation of Performance Of Different Electrode Material In Electro Discharge Machining For Material Removal Rate And Surface Roughness International Journal of Engineering Research and Applications (IJERA) ISSN: 2248-9622, Vol. 2, Issue 3,May-Jun 2012, 3072-3076.
[7]. C. R Barik, N. K Mandal, Parametric Effect And Optimization Of Surface Roughness Of EN 353 In CNC Dry Turning international journal of lean thinking, volume 3, issue 2 (December 2012), 54-66.
[8]. A text book by R. Ranjit, A Primer on the Taguchi Method, van Nostrand Reinhold, New York (1990).