
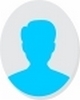
- Open Access
- Authors : M.Abhinaya, B.Krishna Varun, K.Keerthana, J.Spandana
- Paper ID : IJERTV13IS030191
- Volume & Issue : Volume 13, Issue 03 (March 2024)
- Published (First Online): 30-03-2024
- ISSN (Online) : 2278-0181
- Publisher Name : IJERT
- License:
This work is licensed under a Creative Commons Attribution 4.0 International License
New Born Baby Face Identifier
M.Abhinaya, B.Krishna Varun, K.Keerthana, J.Spandana
UG Student, Department of CSE, CMR College of Engineering & Technology, Hyderabad
Assistant Professor, Department of CSE,CMR College of Engineering & Technology,Hyderabad,Telangana
Abstract Recognizing babies or newborn children faces holds fore most significance over different divisions. It serves as a significant degree in deflecting occurrences such as infant swapping in healthcare settings, guaranteeing exact distinguishing proof and gathering with their natural guardians. Moreover, it plays a basic part in finding lost children, helping law authorization offices through exact facial acknowledgment. In addition, it encourages the
proficient dissemination of fundamental benefits and administrations custom fitted to babies, counting healthcare, instruction, and social welfare programs. Integration of progressed calculations like VGG-16 and show such as CNN and ANN assist improves the viability and exactness of infant confront acknowledgment frameworks, in this manner fortifying the security, security and welfare of babies.
IndexTerms Exact Recognizable proof, Convolution Neural Systems (CNN), VGG-16, Progressed Calculations, Client involvement, Client interface.
-
INTRODUCTION
Biometric acknowledgment of newborns, little children, and pre-school children points are vital to inquire about challenge with applications in distinguishing infant swapping, lost kids, and disbursing benefits. In this investigate, we propose a representation learning calculation to extricate appealing and invariant highlights from confront pictures of newborns and little children, to plan a proficient confront acknowledgment calculation. Particularly, we propose a profound learning show which applies class-based punishments whereas learning the channels of a convolution neural organize.
Biometric modalities are these days utilized in a few character administration applications such as national recognizable proof ventures, law authorization; get to control, and reconnaissance. Be that as it may, theres exceptionally constrained scholarly proof to demonstrate the unwavering quality of these modalities for acknowledgment of newborns and toddlers, particularly within the age bunch of 0-4 a long time. In a later occurrence in India, 14 children were protected from places where they were found asking and picking clothes as portion of constrained labor.
Fig 1: This study is all about recognizing the faces of newborns and toddlers. It's tricky because babies and young children don't always cooperate when you try to take their pictures. Plus, there aren't many datasets available with pictures of babies and toddlers, which makes it harder to do research in this area
-
LITREATURE REVIEW
A.K. Jain &S. S. Arora [1] in this paper they examined around developing require for utilizing biometrics, like fingerprints, to recognize children, particularly in places where they do not have any other shape of ID. This will offer assistance keep track of when kids require inoculations, discover lost children, anticipate extortion in nourishment help programs, and halt babies from being switched in clinics.
O.Bellon [2]in this article they proposed a novel biometric recognizable proof strategy for infant babies utilizing their palm prints unused tall determination optical sensor was created, which gets pictures with sufficient edge particulars to interestingly distinguish the infant. The palm and impression pictures of 106 newborns were examined, driving to the conclusion that palm prints surrender more point by point
pictures at that point impressions. Unique mark specialists from the Identification Organized of Parana State performed two coordinating tests, coming about in a redress recognizable proof rate of 63.3% and 67.7%, more than three times higher than that gotten on comparable tests depicted on writing. They proposed picture procurement strategy moreover opens the viewpoint for the creation of a programmed recognizable proof framework for newborns.
Within the past few a long time, thinks about have been conducted to decide the unwavering quality of fingerprints [1], impressions [2], palm prints [5], confront [6], [7], [8], iris [9], and a combination of a few of these modalities for biometric acknowledgment of newborns and little children independently.
T. Ahonen, A. Hadid, and M. Pietikainen[3]describe a new method for representing facial images using a technique called Local Binary Pattern (LBP) on neighboring parallel plan surface highlights. They divide the face picture into parts, extract LBP histograms from each part, and then combine them into one vector to describe the face. They check how well this method works for recognizing faces under different conditions and also discuss other possible uses and improvement.
G. Goswami, R. Bhardwaj, R. Singh, and M. Vatsa[4] clarify that recordings contain a parcel of data within the shape of outlines, which can be utilized for highlight extraction and coordinating. Be that as it may, not all confront pictures in these outlines are valuable or important. In this manner, utilizing all outlines accessible in a video for acknowledgment doesn't essentially make strides exactness.
W. Jia, H. Cai, J. Gui, R. Hu, Y. Lei, and X. Wang [5] in this paper, they proposed a novel online infant individual confirmation framework for this issue based on impression acknowledgment. Infant and newborn child individual confirmation may be a basic issue for healing center, birthing centers, and other teach where different births occur, which has not been well considered within the past.
S. Bharadwaj, H. S. Bhatt, R. Singh, M. Vatsa, and S. K. Singh
[6] in this paper, a multiobjective developmental granular calculation is proposed to coordinate confront pictures some time recently and after plastic surgery. The calculation to begin with creates non-disjoint confront granules at numerous levels of granularity.In another consider, Bharadwaj et al. [7] proposed a two-stage domain-specific learning for infant confront acknowledgment. P.Basak et al. [8] created standard comes about for little child confront acknowledgment by assessing existing apparatuses and calculations.
In expansion to giving the preparatory approaches, Bharadwaj et al. and Basak et al. [9] too presented the Infant Confront Database and CMBD, separately. Accessibility of these databases has encourage persuaded us to proceed the inquire about in this space and building up the unwavering quality of confront a compelling methodology for one of a kind distinguishing proof of newborns and little children.
L. Best-Rowden, Y. Hoole, and A.K.Jain [8] moreover presented the Newborns, Newborn children, and little children Longitudinal (NITL) confront picture database and illustrated about of commercial off-the-shelf (Bunks) confront matcher on this database. Be that as it may, this database isnt freely accessible.
Galton et al. [10] collected inked unique mark impression of a single child and physically assessed the believability of unique finger impression acknowledgment.
F. Schroff, D. Kalenichenko, and J. Philbi [11] This methodology businesses a basic convolution organize organized to particularly optimize the embeddings itself, rather than a center of the street bottleneck layer as in past vital learning approaches. To organize, we utilize triplets of for the first parcel balanced organizing / non-matching stand up to patches made utilizing a novel onlne triplet mining strategy. The advantage of this approach is much more essential representational reasonability.
-
Proposed methods:
VGG-16: which consists of 13 convolution layers and 3 dense layers.The VGG-16 architecture is a specific convolution neural network (CNN) architecture proposed by the Visual Geometry. It is characterized by its simplicity and effectiveness in image classification tasks
Fig 2: Cnn structure
CNNs, or Convolution Neural Systems, are interesting in that they combine both feature extraction and classification. Envision a basic CNN spoken to in Figure 1, which comprises of five layers: an input layer, a convolution layer, a pooling layer, a fully-connected layer, and an yield layer. These layers are partitioned into two parts:
Feature extraction and classification. Feature extraction:
This portion incorporates the input layer, the convolution layer, and the pooling layer. The input layer indicates the measure of
the input pictures, which may be resized in the event that essential. At that point, the picture is handled through different convolution layers where it is convolved with learned parts utilizing shared weights. Another, the pooling layer decreases the picture measure whereas protecting imperative data. The yields of this portion are called include maps.
Classification:
In this portion, the extricated highlights from the highlight extraction portion are combined within the fully-connected layers. Each question category has its own yield neuron within the yield layer. The yield of the classification portion is the classification result, demonstrating the anticipated category of the input picture.
The last three dense layers typically serve different purposes:
-
First Dense Layer (Fully Connected Layer): This layer often acts as a bottleneck layer to reduce the dimensionality of the feature representation before the final classification. It usually has a large number of neurons, often in the order of several hundreds.
-
Second Dense Layer (Fully Connected Layer): This layer further refines the feature representation learned from previous layers and prepares it for the final classification. It usually has fewer neurons compared to the first dense layer.
-
Third Dense Layer (Final Output Layer): This layer is the output layer of the network and typically contains the number of neurons equal to the number of classes in the classification task. It applies an appropriate activation function (such as softmax for multi-class classification) to produce the final probability distribution over the classes.
-
METHODOLOGY
The proposed confront acknowledgment framework may be an profound convolution neural organize VGG-16(CNN) engineering that consolidates class-based punishments amid channel learning. It is particularly outlined to address the challenges of tall varieties in infant and little child confront pictures, whereas leveraging the representation learning capabilities of profound systems. The CNN contains 13 layers with interleaves convolution, ReLU enactment and max pooling layers. Class-specific punishments are included to the optimization objective amid preparing that actuates class-discriminative channels and dodges over fitting to the little datasets. The organize is to begin with pre-trained on a expansive grown-up confront dataset at that point fine-tuned utilizing expanded information from infant and little child confront datasets. Skip associations permit preparing more profound models. Amid testing, pictures are forward passed through the CNN layer highlight extractor and confront layouts are coordinated in a 1: 1 confirmation setting. At long last, rank-1 recognizable proof rates of 62.7% for newborns and 85.1% for little children are accomplished employing a single reference picture per child. The compact engineering can coordinate pictures in 0.1 seconds making it reasonable for real-time applications. In rundown, it leverages profound learning with customizations like course punishments to address challenges special to newborn child confront acknowledgment. The proposed methods lead to state-of-the-art exactness on this difficult problem.
Data Set:
The dataset consists of images of babies collected from Instagram. Instead of directly downloading the images, screenshots were taken from Instagram posts that featured pictures of babies. The database consists of four categories of baby images based on age: one month, six months, one year, and ten months. Each category offers a unique glimpse into the growth and development of babies at different stages of their first year of life. The database provides a diverse collection of baby images, allowing for various analyses, research, and applications related to infant development and childcare. These images may depict babies of different ages, in various settings, poses, or activities, depending on what was shared by the Instagram users. The dataset aims to gather a diverse collection of baby images from Instagram to be used for various purposes, such as research, analysis, or application development related to babies or childcare.
Data Preprocessing:
Image preprocessing in CNN layer involves resizing images to a standard size, normalizing pixel values to a common range (usually 0 to 1), and converting color images to grayscale if needed. Data augmentation techniques such as rotation, flipping, and cropping are applied to increase dataset diversity. Preprocessing also includes center cropping or padding to ensure consistent input dimensions. Specialized libraries like OpenCV, Pillow, or scikit-image in Python are commonly used for these tasks. Overall, image preprocessing enhances data quality and usability, improving performance and accuracy.
Feature Extraction:
Feature extraction in CNN layer for newborn baby face identification involves: Convolution Layers: Detect patterns like edges and shapes in baby faces. Activation Functions: Apply ReLU to capture complex relationships. Pooling Layers: Down sample feature maps to retain important information. Flattening: Convert feature maps into a one-dimensional vector. Fully Connected Layers: Extract high-level features for classification. Output Layer: Assign probabilities to different baby classes for identification.
The VGG-16 architecture:
The design utilized within the show generally compact. This streamlined plan essentially decreases memory and computational requests, upgrading the model's availability and empowering sending over a wide extent of stages and gadgets with shifting computational assets. Design the architecture of the vgg-16 model for newborn baby face identification. This may include specifying the number of convolution layers, pooling layers, and dense layers, as well as choosing appropriate activation functions and optimization algorithms.
Training the dataset:
Through pre-training on an outside dataset and leveraging information expansion methods, the vgg-16 show viably mitigates the challenge of constrained preparing information for newborns and little children. By consolidating an assorted dataset and expanding existing information, that demonstrate accomplishes progressed generalization, improving its capacity to precisely recognize faces with changing highlights. Train the
Implementation of Block diagram:
Start
Importing dataset (images) postgresql
Data Set
Data Preprocessing
Feature Extraction
Training the model
CNN VGG-1 6
Classification
Testing
Login& Register
Input
Upload image
Suggests vaccine
Face detection
Final
vgg-16 model using the training set of newborn baby face images. During training, the model learns to recognize distinctive features and patterns in the images and adjusts its parameters to minimize the prediction error
Classification:
Classification in a newborn baby face identifier involves using a fully connected layer to assign age group labels, such as one month, six months, one year, or ten months, to input images. The dense layer extracts high-level features and maps them to the corresponding age groups through a softmax activation function. During training, the model learns to minimize the difference between predicted probabilities and actual age group labels, enabling accurate classification of new images based on their facial features.
Web Application:
The system emphasizes client comfort and security. A strong enrollment framework is executed to gather fundamental client data, whereas client confirmation components defend get to the framework. Client interaction modules, home, upload picture, view results and logout functionalities, are planned to supply a consistent experience for users interacting with the face recognition system. An admin board is additionally joined, highlighting authoritative functionalities such as client administration and framework oversight.
Fig.3: Block diagram of proposed systems
Implementation
-
RESULT AND DISCUSSION
-
Comparison of Algorithms
Algorithms
Accuracy
LBP
28.8
LDA
71.3
PCA
38.8
Fine-tune-VGG-Face
83.0
VGG-16
85.1
Table 1: Rank-1 recognizable proof correctness and standard deviation (%)
Local Binary Patterns (LBP) is a technique used in face recognition because it's not affected by changes in lighting. It works by looking at each pixel in an image and comparing it to its neighboring pixels. A circular LBP version extends this idea by considering a circular region around each pixel. It then assigns a number to each pixel based on whether its neighbors have higher or lower intensity values. This process creates a binary representation of the image.
The best part is, there's no need for a specific training process with LBP. Instead, it directly analyzes the features of test images. These features are then compared using a statistical method called the chi-square distance to determine how similar or different they are. Overall, LBP is effective for recognizing faces because it's robust to changes in lighting conditions and doesn't require extensive training.
Linear Discriminant Analysis (LDA) is like PCA but focuses on preserving important differences between different classes of data while reducing the number of dimensions. It transforms images by projecting them into a space called Fisher space, where the features that distinguish between different classes are emphasized. This transformation results in a new representation of the images with the extracted features. Just like PCA, LDA uses Cosine Similarity to compare these features for matching purposes. In essence, LDA helps in reducing the complexity of data while keeping the essential information needed to distinguish between different classes.
Principal Component Analysis (PCA) is a statistical method used to simplify complex data by reducing its dimensionality. In face recognition, PCA is applied to lessen the number of image variables by focusing on the features that vary the most across different faces. Each image in the training set is broken down into a combination of fundamental patterns called eigenvectors. From these, a set of significant eigenvectors known as "Eigen faces" are chosen to represent the data in a lower-dimensional space. This transformation helps in extracting key features from the images. To compare faces, Cosine Similarity is used to measure how similar their feature representations are. In essence, PCA helps in capturing the essential facial
characteristics while reducing the computational burden of processing large amounts of data.
Fine-tuned VGG Face involves adjusting a pre-existing model called VGG-Face, which is already trained to recognize faces. In this process, the last six layers of the model, which specialize in identifying intricate facial features, are refined using images of newborns and toddlers. This adaptation ensures that the model becomes more adept at recognizing the unique characteristics present in infant and toddler faces, improving its accuracy for these age groups.
The proposed deep learning algorithm's effectiveness is evaluated by comparing it with traditional methods and other learning-based techniques. We measure its performance alongside algorithms like PCA, LDA, LBP, and Fine-tuned VGG Face using two databases: the Newborn Face Database and the Children Multimodal Biometric Database. We summarize the accuracy results in Table I to understand how well the algorithm performs in distinguishing between different faces.
-
-
-
2 Figures:
Fig 4: (a) Graphical Representation of Training and Validation: Preparing the method of altering the parameters of neural arrange to play down a fetched function. Validation is the method of measuring the precision of a show on a subset of the information
(b) Graphical Representation of Training and validation Loss: Preparing and approval gives vital data since they allow us distant better; a much better; a higher; a stronger; an improved">a much better understanding into how the learning execution changes over the number of ages and makes a difference us analyze any issues with learning that can lead to an underfit or an overfit show.
4.3 PROPOSED METHOD OUTPUT IMAGES:
Fig 5: Home page of proposed system
Fig 6: Registration page of proposed for user.
Fig 7: Face recognized by proposed system which suggests vaccine for the baby and baby age.
Fig.8: Graph represents overview of the model performance across different age groups.
-
CONCLUSION
-
Infant exchanging and snatching are exceptionally critical problems over the world. A few biometric and non-biometric methods have been assessed to diminish the number of such frequencies. Biometric methods incorporate impression, and palm print with human specialists performing the coordinating. This investigates progresses state-of-the-art for coordinating confront pictures of newborns, little children and preschool children. We to begin with show a novel definition to join class-based punishment for channel preparing within the CNN design. The proposed approach is expanded for confront acknowledgment for newborns, toddlers, and pre-school children. The proposed profound learning approach outperforms existing handcrafted and profound learning based approaches and accomplishes state-of-the-art confirmation and recognizable proof exactnesses for the databases.
In future, we arrange to extend the database in measure to perform a more expansive scale evaluation of the procedure. Moving forward, we arrange to work on video based confront acknowledgment for recognizing confront pictures relating to newborns, little children and preschool children. Calculations such as outline choice in recordings, joint-feature learning, and combination can offer assistance progress the execution of confront acknowledgment in practical conditions.
REFERENCE
-
A. K. Jain, S. S. Arora, K. Cao, L. Best-Rowden, and A. Bhatnagar. Unique mark acknowledgment of youthful children.
IEEE Exchanges on Data Forensics and Security, 12(7): 15011514, 2017.
-
O. Bellon, L. Silva, and M. N. L. Cat. Newborn's biometric distinguishing proof: Can it be worn? Out 3rd Universal Conference on Computer Vision Hypothesis and Applications, volume 1, pages 200205, 2008.
-
T. Ahonen, A. Hadid, and M. Pietikinen. Confront portrayal with neighborhood parallel designs: Application to confront acknowledgment. IEEE Exchanges on Design Examination and Machine Insights, 28(12): 20372041,
2006.
-
G. Goswami, R. Bhardwaj, R. Singh, and M. Vatsa. Mdlface: Memorability increased profound learning for video confront acknowledgment. In IEEE Worldwide Joint Conference on Biometrics, pages 17, 2014.
-
W. Jia, H. Cai, J. Gui, R. Hu, Y. Lei, and X. Wang. Newborn child impression affirmation utilizing presentation highlight. Neural Computing and Applications, 21(8):18551863, 2012.
-
S. Bharadwaj, H. S. Bhatt, R. Singh, M. Vatsa, and S. K. Singh. Confront acknowledgment for newborns: A preparatory ponder. In IEEE Worldwide Conference on Biometrics: Hypothesis, Applications and Frameworks, pages 16, 2010.
-
L. Best-Rowden, Y. Hoole, and A. Jain. Programmed confront acknowledgment of newborns, newborn children, and little children: A longitudinal assessment. In 2016 Worldwide Conference of the Biometrics Uncommon Intrigued Gather (BIOSIG), pages 18, 2016.
-
P. Basak, S. De. M. Agarwal, A. Malhotra, M. Vatsa, and
R. Singh. Multimodal biometric acknowledgment for little children and pre-school children. IEEE Universal Joint Conference on Biometrics, 2017.
-
F. Galton. Finger prints of youthful children. Report of the British Affiliation for the Headway of Science, 69: 868869, 1899.
-
F. Schroff, D. Kalenichenko, and J. Philbin. Facenet: A bound together embedding for go up against affirmation and clustering. In IEEE Conference on Computer Vision and Plan Affirmation, 2015.