
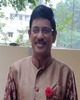
- Open Access
- Authors : G. V. Ramanaiah , L. Krishna Kavya , P. V. Rajya Lakshmi , V. Sai Kumar, S K. Shahed Ali
- Paper ID : IJERTV9IS060769
- Volume & Issue : Volume 09, Issue 06 (June 2020)
- Published (First Online): 03-07-2020
- ISSN (Online) : 2278-0181
- Publisher Name : IJERT
- License:
This work is licensed under a Creative Commons Attribution 4.0 International License
Optimal Energy Efficiency in Backhauled 5G Small Cells with Massive MIMO Enabled MM Wave Communication
1G.V. Ramaniah
Associate Professor,
Department of Electronics and Communication Engineering, PSCMR College of Engineering and Technology Vijayawada, Andhra Pradesh, India
2L Krishna Kavya
UG Student
Department of Electronics and Communication Engineering PSCMR College of Engineering and Technology Vijayawada, Andhra Pradesh, India
3P Venkata Rajya Lakshmi
UG Student
Department of Electronics and Communication Engineering, PSCMR College of Engineering and Technology Vijayawada, Andhra Pradesh, India
4V Sai Kumar
UG Student
Department of Electronics and Communication Engineering PSCMR College of Engineering and Technology Vijayawada, Andhra Pradesh, India.
5S K Shahed
UG Student
Department of Electronics and Communication Engineering PSCMR College of Engineering and Technology Vijayawada, Andhra Pradesh, India
AbstractWith the increase of recent operator(s) for cell equipments making use of 4G services, those 4G services increased rapidly. Presently focus is moved to 5G advances in particular meeting higher data rates. So as to satisfy the necessary datarates of network ,densification is clear manner. Combination of Small cells in ultra dense with Massive MIMO enabled base stations using mm Wave backhauling instead of conventional microwave backhauling is a successful method for accomplishing network densification but it to should be designed in an Energy effective manner. In this paper we will discuss an optimal Energy Efficient(EE) tractable version for designed Small cell DPSN(Digitally Phase Shifter Network) structure integrating Massive MIMO primarily based mm wave backhauled network. In this paper we observe the deployment practicality of DPSN based 5G Small cell network with Massive MIMO based mm Wave backhauled network. Next, uplink general Energy efficiency(EE) optimized frame work which is tractable is calculated for discussed network with respective to density of Small Base Station(BSs),the transceiver hardware impairments and the pilot reuse factor. Important feature of proposed network is concurrently supporting several Small Base Stations(SBS) in an energy efficient way.
Keywords 5G, Network densification, mm Wave, DPSN, Massive MIMO, Optimal Energy Efficiency
-
INTRODUCTION
The explosive data demand is certainly one of important issues in contemporary cellular networks, which includes the advanced fourth technology (4G) network. There is a need for next technology of wireless communication networks (5G) which could be useful resource for ever-increasing needs of higher data rates and additionally can make certain graded Quality of service (QoS) through the entire network. Simultaneously the power
consumption of the Information and communication technology (ICT) enterprise and the corresponding energy related pollution have become important societal and economical concerns. To meet such a 1000_factor higher data rates without increment in the ICT footprint, new technologies that improve the over all energy performance through 100_ need to be developed. It has been the consensus that destiny 5G networks must recognise the goals of 1000-fold system capacity, 100-fold energy efficiency, and 10-fold decrease latency. To build such a highly aggressive 5G network two promising technologies are small cells and Massive MIMO. Combination of two technologies allows us to gain the network densification in an energy efficient manner. Due to ultra-dense small-cell BSs, better frequency reuse can be acquired, and energy performance also can be advanced substantially because of the reduced route loss in small cells. To get access to ultra-dense small cell base stations in network, a dependable, price affordable, gigahertz bandwidth back haul connecting the macro cell BS and the related small-cell BSs is prerequisite. It has been validated that backhaul with 1~10 GHz bandwidth can sufficiently assist small cellular network in an effective way. Conventional optical fiber enjoys large bandwidth and reliability, however its application to ultra-dense network as backhaul might not be a low-price preference for operators due to the restrict of deployment and installation. Hence, wireless backhaul, is promising solution for operators to over come the geographical constraints. The benefits of mm Wave backhaul are: A massive amount of underutilized band in mm Wave can be leveraged to offer the capability of gigahertz transmission bandwidth, which differs from scarce microwave band in conventional mobile networks. Many number of antennas can be deployed without issue in mm Wave communications due to the
small wavelength of mm Wave, that can enhance the signal directivity (reduce the co-channel interference) and link reliability (mitigate the massive route loss) for mm Wave backhaul. This paper considers the combination of mm Wave with massive range of antennas, that's also referred to as mm Wave massive Multiple Input Multiple Output (MIMO), to provide wireless backhaul for future ultra-dense 5G small cellular network. However when network includes massive MIMO base stations, the area throughput is increased by the use of the multiplexing gain, even as the array gain from coherent processing lets in for primary decrementation within the emitted energy. Similar to small- mobile networks, however, the potential throughput gain from massive MIMO come from deploying more hardware (i.E., multiple antenna branches for one BS), which in turn increases the circuit energy intake of one BS. In short, each densification technologies can improve the area throughput and reduce the radiated power, but on the cost of deploying extra hardware infrastructure. The EE of the network may be improved if those advantages and costs are properly balanced. The major objective of this paper is to optimize proposed network i.E. Integration of those technology for maximal EE.
-
Related Works.
The EE of cell networks has been defined from numerous perspectives. One of the maximum commonplace definitions is a benefit-cost ratio, wherein the service quality per area unit(a. U.) is compared with the associated energy expenses. In this paper, the following desired definition is used
Area spectral efficiency
=
+ .
Most of the previous works that calculated the EE, as described above, have focused at the single-cell case in which the interference from other cells is neglected. But the EE calculation of multi-cell networks is much greater concerned than in the single cell case due to the complex network topology and the arising inter-cellular interference. The only method is to rely on heavy Monte Carlo simulations. But, Monte Carlo simulated effects are frequently anecdotal given that one can't separate essential properties from behaviors caused by way of parameter selection. In an other way network topologies like Wyner version or the symmetric grid-based deployment can be considered. But those models cannot stop the irregular shape of deploying small mobile base stations. The necessity for growing tractable models for future dense networks has extended the focus in random spatial concepts; precisely, in using tools from stochastic geometry, in which the BS locations forms attention of a spatial point processtypically a Poisson point process (PPP). A huge benifit of this technique is the capability of providing tractable expressions for key performance metrics together with the coverage probability and the average SE of the network. Afew previous works have additionally derived EE- associated overall performance metrics and showed how those depend upon the BS and UE densities.This paper makes a speciality of Up Link (UL) analysis in contrast to other papers, the bulk of work at the EE in multi-cellular networks using stochastic geometry has targeted
on the Down Link(DL). Furthermore, previous works commonly goals at calculating the premiere Energy efficient parameters for the proposed network as shown in fig 1. making use of the DPSN transceiver structure.
Figure1: mm Wave massive – MIMO- based wireless backhaul for 5G Ultra dense small cells network
-
Major Contributions:
This paper considers the UL of a multi cellular multi user environment of network and calculating optimal EE.To achieve multi cell multi user network,P2MP dependable backhauling is necessary. So, considering Digital Phase Shifter Network (DPSN) based totally definitely mm wave Massive MIMO network is better solution. Because conventional mm wave multi-antenna systems utilize single RF chain and analog phase shifters for precoding / combining are constrained to Single- User[SU]-MIMO with a single circulation. Full digital precoding in microwave massive MIMO aids multi-user[MU] MIMO but it desires for one precise RF chain to be connected to every antenna which can be unaffordable in mm wave communications. However combining of each analog and digital i.e., the hybrid precoding / combining scheme is validated to be effective for mm Wave massive MIMO with reduced value and complexity of transceiver. Yet it's far supported by using MU-MIMO with single stream for each person but for the MU-MIMO with multiple streams DPSN based totally hybrid precoding / combining scheme verified to offer the better spectral efficiency MU-MIMO with multiple of streams. In this network we're considering approximately the distribution of BS in keeping with a homogenous PPP of depth . Each BS is equipped with M antennas and communicates with K single-antenna UEs uniformly dispensed within its coverage region. The expression of recent lower bound of the common SE that is computed from equipment of stochastic geometry and classical records is used to outline the EE metric through additionally the use of the power intake version developed , which not debts for the radiated energy however additionally for the operating energy required by
(amongst others) analog transceiver chains, digital processing, and backhaul infrastructure.EE maximization problem formulated beneath the idea that a given common SE target per UE ought to be met with equality, to guarantee good service
= (1
) log2(1 + )
exceptional.
-
Outline
The paper is categorised as follows section-II introduces the
To specify the APC, we start by observing that with the adopted power-control policy the average radiated energy in line with UE is
System model of the network this is underneath investigating. Section III presents the generalized work maximization of EE of network with respective to optimization variables pilot reuse
( 1)
{ } = (1
1
( + 1)
) 2 ()/2
element, BS density, transmission power in addition to the sort of UEs and antennas according to BS. The presented optimized EE formulae are calculated and analyzed numerically for the proposed network in Section IV. Finally, the foremost conclusions and implications of the paper are drawn in Section- V.
-
-
SYSTEMMODEL
We keep in mind the UL of a mm wave backhauled Massive MIMO enabled ultra-dense small cell network that is designed to
where we have used the truth that each user transmits one pilot symbol and S-K information symbols according to coherent block of total length S. Then, we test that the APC ought to account now not only most radiated power, but additionally for the dissipation in analog and digital hardware, digital signal processing, backhaul signaling, and other overhead costs. A particular and generic model that takes all those elements into attention the currently proposed and the APC is computed as
serve a dense heterogeneous distribution of UEs. This is modeled the usage of the stochastic geometry framework adopted in
= ((1
1
( + 1)
) 2 + 0 + 1 + 0
which the Small cellular BSs are distributed spatially in2in line with a homogeneous PPP of intensity .More precisely,in any area of length A the wide variety of Small cellular BSs is a
()/2
Poisson allotted stochastic variable with mean cost A. The BSs are uniformly and independently allotted over the location. Each of them is ready with an array of M antennas and serves K single antenna UEs, which can be decided on at random from a very huge set of UEs within the cellular region that we deal with K additionally taken into consideration as considered one of optimization variable. We considering that every UE connects to its nearby BS such that coverage area of a BS is given by its Poisson Voronoi cellular. The UEs are considered to be disbursed uniformly within the Poisson- cell in their respective serving BS. It is clear that the geographic areas of UEs and BSs are correlated in this version, such that small cells serve more number of UEs in unit area compared to large cells. We interpret this as a complex network deployment wherein the BSs are matched to a heterogenous distribution of UEs. The translation
+ 1) + .
where (0, 1] is the linear power amplifier efficiency, C0 models the static power intake at a BS, and and D0M is the power consumption of the BS transceiver chains which scales with the quantity of BS antennas. Moreover, C1K +D1MK represents the power fed at the UEs and also with the aid of the signal processing tasks on the SBS. Optimizing of EE problem considered in the network is structured as follows, for a frame length(S), propagation variables (,), and hardware impairment characteristics (,,A,C0,C1,D0,D1) for a tuple of parameters
=(, , , K, M ) the EE maximization problem is
()
max () =
invariance of PPPs permits us to carry out statistical overall performance assessment for a normal UE (located for instance within the origin), that is statistically consultant for every other UE in the network . Assume that this preferred UE has the
subject to SINR =
()
arbitrary index k and is connected to a BS this is known as standard BS and is denoted as BS0
-
FRAMEWORK
As discussed in Section II, we focus on the UL EE defined because the benefit-cost ratio between the Area spectral performance (ASE) [bit/symbol/km2] and the vicinity electricity intake (APC)[J/symbol/km2]. The ASE may be described as
=
in which SE discussing right here is lower bound accomplished on UL average SE defined in line with UE.
Where is the feasible parameter defined as
= { : 0, 0, 1, (, ) +, }
With K S is the upper limit on pilot signaling overhead. The parameter > zero is used to impose on average SE constraint
2(1 + ) [ bit / symbol / user ] . Using those conditions we acquire maximize EE of network with a suitable throughput. Unconstrained EE maximization often results in operating elements with very low SE per UE.
-
Feasibility
Due to the unavoidable inter-cellular interference in cell networks, the optimization problem is best feasible for a few
values of .This viable variety is obtained as follows .The pleasant SINR constraint is chosen via
<
( 1)(1 2) 1 + 2( 1)
Assume as an example that = 0:05 and consider the particularly conservative propagation parameters = three and S = 200.
For these numbers, the upper restrict of the common SE consistent with UE is log2(1 + 199.5) = 7.65, this is substantally better than the SE of contemporary systems. This approach that the optimization problem is possible in maximum instances of practical interest.
-
Optimization with respective to BS Density
Optimizing with respective to BS density and radiated power the EE maximization trouble reduces to
With EE(*) as given below
The most advantageous values for the BS density and the power conrol
coefficient is probably given with the useful resource of defining for = and thinking about any set of M,K for which maximization problem is feasible then EE(*) is a monotonically developing function of and is maximized as ->.
The average transmit power then, is going to zero. This shows that from an EE perspective it is predominant to have as excessive BS density as viable.
EE(*) =
Firstly, Recall that increasing translates into allocating a bigger portion of every UL block for pilot transmission, so that every pilot symbol is on average best applied in 1/ of the cells within the network. This consequences in higher channel estimation accuracy and much less coherent pilot contamination. Secondly, * is an increasing characteristic of B1 and also of B2, for the motive that a larger B2 makes the denominator smaller. Consequently, The above equation shows that to assure average SINR, * need to grow with K. This is intuitive thinking about that larger UEs for one cell indicating more inter-cell interference, which can be suppressed to certain amount by improving the estimation accuracy and decreasing the pilot contamination; namely, the usage of a more . Similarly, * decreases with because better transmit powers reduce the detrimental impact of noise, leads to higher estimation accuracy and to a extra interference-constrained regime.
(1
1
) (1 + )
1
( + 1)
(1 2)2 2 2
(1 +
1 )
(1 2) 2
2
()/2
+ 0 + 1 + 0 + 1 + (1
1
(1 2)2 2
)2(1 + )
This is probably surprising thinking about smaller cells motive greater interfering UEs inside the area of each cellular, but this trouble is resolved with the aid of the assumed power control policy that steadily reduces the transmit power as the BS density will increase. The most crucial result of letting grow infinitely is consequently that the transmit energy becomes negligible in assessment to the circuit strength in each cell.
-
Optimal Pilot Reuse Factor
For calculation of optimal value of the pilot reuse element with the other optimization variables are fixed, thinking about any set of {, , M, K} for which the optimization problem is feasible
Moreover, * is a reducing characteristic of M thinking about the reality that an improved array gain makes the system much less sensitive to interference and estimation errors. Increment in the pathloss exponent results to a less * this conventional considering intercell interference decays faster. The reality that 1 implies that we can acquire best values of for
1 1which in any other case even = 1 would
(12)22
provide an SINR more than.
-
-
RESULTS
Calculating the Energy Efficiency with respective to the proposed optimized variables mentioned in frame work in mm wave enabled Massive MIMO 5G small cell network produced the results of effects on Energy Efficiency (EE) as follows
-
Optimizing the Energy Efficiency of network:
Three different SINR constraints are taken into consideration in Fig. 1: = 1, 3, 7 which corresponds to the average SEs log2 (1+) 1; 2 ; three . In all 3 values, the EE is computed with the use of the lower bound at common SE. From Fig. 2 within the proposed network, Energy Efficiency Maximization problem solved when BS density is infinitely huge. EE as a function (BS density) will be improved as tends to infinity. It can be drawn from the obtained result that the EE decreases as SINR constraint will increase
Figure 2: Energy efficiency(in Mbit/J) as a characteristic of the BS density in BS/Km2,for distinct SINR constraints.
-
Optimal Pilot Reuse Factor
At = 3 or, equivalently, the average SE per information symbol at log2(1+) = 2. Fig. 3 indicates the EE lower bound as a characteristic of M and K while is optimized. The global EE most gives an EE of 32.74 Mbit/j. and is carried out by manner of (M; K) = (91; 10) the usage of the pilot reuse aspect *= 7:08. The intuition of this quit end result is that the strong inter-cell interference in dense deployments is effectively mitigated by MRC while the BSs are equipped with many antennas and also with the aid of the use of a considerable pilot reuse component. The results also reveal the global optimum solution of network can be studied on basis of power consumptions.Fig.4 reveals the corresponding size of each factor in APC also the power of transmission is considered to be negligible effect. The authoritative terms to be considered are the static power consumption 0 of the transceiver chains of small base station .It can be drawn from results to make hardware more energy efficient 0, 1, 0, 1
are the important terms to be varied to change results at various
conditions.
Fig:3 Energy efficiency (in Mbit/J) for = 3. The global optimum is star- marked with respective to
Fig.4 Representation relative size of each term in APC
-
Impact of Transceiver Hardware Impairments Next, considering the saturation regime in which -> and exemplify the impact of transceiver hardware impairments on the EE. Fig. 4 indicates the EE as a function of hardware impairments ( ) in DPSN community proposed , the level of hardware impairments. As expected, the EE decreases with due to decay of desired signal strength as (1-2)2. The loss is marginal for = 1, however it could be pretty huge while will increase.
Fig4: Energy efficiency(in Mbit/J) as a characteristic of the extent of transceiver hardware impairments, , for different SINR constraints
This result draws that hardware impairments greatly have an effect on the channel capacity in the high SNR regime (i.E., for massive ) while their effect is negligible inside the low SNR regime. The outcomes of Fig. 4 indicates that for the discussed network the EE loss because of impairments can be neglected for low . Since those values correspond to the operating factors that supply the best EE (see Fig. 1), we moreover conclude that proposed DPSN network have a negligible impact of high EE for modest stages of hardware impairments
-
-
CONCLUSION
Achieving Network densification in an Energy Efficient way is a major contribution for 5G Network. The most effective forward way to fulfill the necessities of higher data rates with less traffic is densifying mobile network with mm wave backhauling massive MIMO enabled 5G small cellular network. In this paper we mentioned the feasibility of this network with DPSN transceiver structure and frame work of optimal Energy Efficiency calculation for cell networks. Later, the the usage of the frame work the Optimized EE is calculated for the proposed DPSN primarily based mm Wave backhauled massive MIMO enabled future small cells network. The outcomes acquired shows with growth in small cell base stations, growth in inter cell interference, increase in pilot reuse factor and the increase in value of transceiver hardware impairments effect on EE of the network is optimized at certain level which lets in us to attain an optimized EE through DPSN based 5G network. These insights additionally tested that proposed network can concurrently support more than one Small Base Stations in energy effective way. In destiny this premier EE can be carried out even at the macro Base Station further to in this paper the Massive MIMO enabled at macro base station for simplified calculation, in addition futureworks can put into effect the massive MIMO on the small cellular base stations additionally so that more customers can concurrently be served with larger gain.
REFERENCES
-
Gao, Z., Dai, L., Mi, D., Wang, Z., Imran, M. A. and Shakir, M. Z. (2015) MmWave massive MIMObased wireless backhaul for the 5G ultradense network. IEEE Wireless Communications, 22(5), 1321.
-
Singh, S., Kulkarni, M. N., Ghosh, A. and Andrews, J. G. (2015) Tractable model for rate in self backhauled millimeter wave cellular networks. IEEE Journal on Selected Areas in Communication, 31(10), 21962211.
-
J. Hoydis, M. Kobayashi, and M. Debbah, Green small-cell networks,IEEE Veh. Technol. Mag., vol. 6, no. 1, pp. 3743, 2011.
-
S. Mohammed, Impact of transceiver power consumption on the energy efficiency of zero-forcing detector in massive MIMO systems, IEEE Trans. Commun., vol. 62, no. 11, pp. 38743890, 2014.
-
E. Bjornson, L. Sanguinetti, and M. Kountouris, Designing wireless broadband access for energy efciency: Are small cells the only answer?, in Proc. of IEEE International Conference on Communications (ICC), 2015.
-
E. Bjornson, L. Sanguinetti, and M. Kountouris, Energy-efcient future wireless networks: A marriage between massive MIMO and small cells, in Proc. of IEEE 16th International Workshop on Signal Processing Advances in Wireless Communications (SPAWC), June 2015, pp. 211 215
-
G. Miao, Energy-efcient uplink multi-user MIMO, IEEE Trans. Wireless Commun., vol. 12, no. 5, pp. 23022313, 2013.
-
GreenTouch Green Meter Research Study, Reducing the net energy consumption in communications networks by up to 90% by 2020, Tech. Rep., Jun. 2013.
-
E. Bjornson, L. Sanguinetti, J. Hoydis, and M. Debbah, Optimal design of energy-efcient multi-user MIMO systems: Is massive MIMO the answer?, IEEE Trans. Wireless Commun., vol. 14, no. 6, pp. 3059 3075, 2015.