
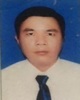
- Open Access
- Authors : Duong Van Dao , Anh Tuan Lai , Tien Lap Do , Van Tuan Pham, Cong Doan Le, Tan Loc Ngo
- Paper ID : IJERTV10IS060254
- Volume & Issue : Volume 10, Issue 06 (June 2021)
- Published (First Online): 23-06-2021
- ISSN (Online) : 2278-0181
- Publisher Name : IJERT
- License:
This work is licensed under a Creative Commons Attribution 4.0 International License
Optimization of High-Speed Cutting Parameters Oriented Improves the Dimensional Accuracy of Alloy Steel SKD11
Duong Van Dao1
1Dong Nai Technical College, Vietnam
Anh Tuan Lai2, Tien Lap Do2, Van Tuan Pham2, Cong Doan Le2, Tan Loc Ngo2
2Advanced Technology Center, Le Quy Don Technical University, Hanoi, Vietnam
Abstract This paper focuses on evaluating the influence of cutting parameters on dimensional accuracy when high-speed finishing milling (HSFM) of alloy steel SKD11 after heat treatment (55HRC). At the same time, the problem of finding the optimal cutting parameter that ensures the smallest dimension error is solved through the genetic algorithm (GA). The optimization problem has constraints both on the value limit of the variables and the limit of the objective function. The experiments were carried out based on the orthogonal experimental method of level 2 with the construction of a system of regression equations describing the relationship between cutting parameters and dimensional accuracy. The research results show the reliability of the regression equation and contribute to improving the machining quality when HSFM of alloy steel SKD11 after heat treatment.
Keywords- High speed milling, alloy steel, dimensional accuracy, optimization.
-
INTRODUCTION
High-speed machining (HSM) is a metal cutting method with a cutting speed of 5 to 10 times that of conventional machining, the temperature at the cutting area will be reduced because it is transferred to the chip [1]. The HSM method has many outstanding advantages when gradually increasing the cutting speed such as increased cutting productivity, increased surface quality, and reduced cutting force. However, as the cutting speed increases, the tool life decreases. Compared with traditional machining, HSM has outstanding advantages. High-speed machining can reduce machining times by up to 50% and reduce machining costs by 30-50%, depending on the case [2].
In metalworking and cutting, processing hard materials in general and hard alloy steel, in particular, is always a difficult problem, complicated by physical phenomena of the cutting process as well as different processing requirements. distinguished from HSM of aluminum, low and medium hardness alloys (below 30HRC), or traditional machining. However, in practice, if the basic characteristics of the cutting process are understood and the problems are solved correctly, hard alloy HSM becomes a predictable process. At this time, the problem of processing and cutting hard materials and hard alloy steel will become more favorable and bring high economic profits.
It is easy to see that alloy such as titanium alloys, alloy steels after heat treatment (hardness above 40HRC) are difficult materials to cut due to their high hardness, high thermal strength, chemical abrasion resistance, and high-
temperature resistance. Temperature and stress are always high in the cutting zone during machining due to poor thermal conductivity, affecting tool wear and chip morphology, increasing machining costs. In other words, when it comes to the HSM of hard alloys, we are often interested in the ability to machine them. Machinability refers to the ease with which a material is cut to the required texture. Factors such as cutting force, cutting heat, vibration, surface quality, tool wear, and wasted energy all affect the finish of the structure, so it can be considered as the basis for evaluating machinability [3]. The problem of HSM of hard alloys has been interested in research in the world for many years. Studies on cutting force can be considered in [4], [5], cutting temperature in [6], [7], vibration
in [8], [9], cutting tool wear in [10], [11], roughness in [12],
[2], [13].Dimensional accuracy is a key indicator in machining accuracy and is the basis of product quality assessment. This factor is directly related to the ability to assemble in the system and affects the working condition of the whole system. However, few studies mention this issue, only a few case studies such as [14], [15], [16], [17], [18], [19], [ 20], [21] but
these studies are not high-speed machining. The influence of technological parameters on the dimensional accuracy when turning aluminum materials is studied in [14]. The results show that when increasing the cutting speed and decreasing the feed rate or vice versa, the dimensional accuracy is guaranteed. The dimensional accuracy is highest when the cutting speed is high, the depth of cut is large and the feed rate is small. the problem of tool wear, surface roughness, and dimensional accuracy when machining AISI 4140 alloy using low-temperature coolant are considered in [15], [18]. The results show that the cold coolant helps to reduce tool wear, increase durability, and significantly reduce the dimensional deviation.
The method of measuring and analyzing the cutting force and vibration during the steel alloy turning to determine the surface roughness and dimensional deviation based on the Neural algorithm is presented in [16]. the causes of dimensional deviation including error in machine geometry, cutting heat, control system, and cutting force are described in [17]. The study concludes that shear force has the most influence on dimensional deviation. A model which is established in [19] to predict geometric roughness and dimensional accuracy through imaging technology during machining. The parameters obtained by taking pictures are put into the simulation environment, combined with technological
parameters, the author can predict the roughness and dimensional accuracy. Experimental verification for some cases confirms the feasibility of the method. The optimal tooling strategy to ensure dimensional accuracy when high- speed milling of very small parts (micromachining) is used in [20]. The influence of technological parameters on dimensional accuracy when machining AISI 4340 hardened steel dry turning is studied in [21]. The author concludes that cutting speed and CBN-coated turning tip radius have the most influence. to dimensional accuracy.
Due to the limitation of manufacturing technology of machines, cutting tools, and other equipment, there are not many publications on the issue of dimensional accuracy when high-speed processing for alloy steel after heat treatment with high hardness. This paper focuses on studying the dimensional accuracy when high-speed finishing milling of alloy steel SKD11 after heat treatment with hardness above 55HRC. The relationship between the shear parameters and the dimensional accuracy is shown through the construction of the regression equation. Level 2 orthogonal empirical method is applied to build this mathematical equation. The genetic algorithm with the objective function which is constrained is used to find the optimal set of cutting parameters that minimize the dimension error.
The measuring device is a CMM CONTURA G2. The machine can scan actively and multi-sensor. Scan points up to 200 points/s. The variable measuring force from 50 to1000 mN ). Max needle length is 500 mm, minimum needle diameter is 0.5 mm. The linear error of the MPE measurement length according to ISO 10360-2: 2009 is 1.8 µm with the measuring length 300mm, the repeatability error is 1.4 µm. The strategy for finish milling is the Profile cycle. The diameter of the circular pocket before finishing is 21.8 mm. The required pocket diameter is 22mm. Pocket depth reaches 11mm.
Fig. 1. FR-1E/Hard hardness tester and SKD11 55HRC workpiece
-
MATERIALS AND METHODS
-
Experiment set up
The experimental objective of HSFM of alloy stee SKD11 after heat treatment (55HRC) is to determine the relationship between cutting parameters (depth of cutting, feed rate, and spindle speed) in HSFM with dimensional error through the regression equation. This equation is built based on the level 2 orthogonal empirical method. The workpiece material is SKD11 (Japanese Standard JIS G4404) with the composition described in Tab. I.
Ingredient
C (%)
Si (%)
Mn (%)
Cr (%)
Mo (%)
V (%)
P (%)
SKD11
1.4-1.6
0.6
0.6
11-
13
0.7-
1.2
1.1
0.03
Ingredient
C (%)
Si (%)
Mn (%)
Cr (%)
Mo (%)
V (%)
P (%)
SKD11
1.4-1.6
0.6
0.6
11-
13
0.7-
1.2
1.1
0.03
TABLE I. CHEMICAL COMPOSITION OF SKD11 ALLOY STEEL WORKPIECE
Fig. 2. Carbide Endmill tool YG SGN09080H and experimental model
The level 2 orthogonal experimental method
This is a nonlinear planning method, the structure has a center, the number of influencing factors is 3 factors (cutting depth, feed rate, and spindle speed), the stepover parameter is fixed. The total number of experiments to be performed is 15. The alpha quantity is the distance from the center of the experimental structure to the position of points on the coordinate axis of the structure. Conventional influencing factors according to the variables are as follows, cutting depth
SKD11 workpiece was heat-treated in the Turbo-IPSEN
t(mm )
is x1 , the feed rate F(mm/min) is x2 , spindle speed
furnace and hardness measured on an FR-1E/Hard machine (Fig.1). Hardness measurement results with the average value
n(rev min) is x3 . Note that the spindle speed can be related
of SKD11 alloy steel workpiece after heat treatment reached 55 HRC. Regarding machining machines, experiments were carried out on HSM machines DMC1450V manufactured in 2018. The maximum tool speed according to X, Y, Z reaches
42.000 mm/min, the spindle speed reaches the maximum of
to the cutting speed (Vc (m
V Dn
c 1000
(1)
V Dn
c 1000
(1)
given below
min) ) through the calculation
20.000 rpm, the spindle power reaches 35 kW, the maximum load force on the X, Y, Z axes reached 6.5 KN. The maximum position accuracy reaches 6µm (According to VDI/DGQ 3441).
where, D is the tool diameter, min)
speed.
-
Perform experiments
is the spindle
The cutting tool used for the experiment is a specialized high-speed carbide Endmill tool YG SGN09080H. The cutting tool code is NX5070 SGNF09080H, cutting diameter is 8mm, shank diameter is 8mm, cutting length is 20mm, total tool length is 65mm with 4 cutting teeth (Fig. 2a). The experimental model is depicted in Fig. 2b.
Based on the study of previous publications on the HSM for different materials, different hardness, assessment of the machining ability of SKD11 with HRC55, the machining ability of CNC machines, the domain of cutting parameters recommended by the cutting tool manufacturer, and test machining results, the range of experimental cutting
parameters value are specifically selected as shown in Tab. II. The experimental matrix table is described in Fig. 3.
TABLE II. LIMITING DOMAIN OF EXPERIMENTAL CUTTING PARAMETERS VALUE
compared with nominal pocket diameter based on orthogonal level 2 method is obtained as follows
Err 0.02365 0.02961x 0.01234x
1 3
0.018456x x 0.01521x x 0.01989x 2 1 2 2 3 1
(2)
The regression equation reconstructed on the ANOVA module of Design Expert 12 software also gives similar results (Fig. 4).
Change the variable back to the cutting parameters
x t t0 ;x F F0 ;x n n 0 1 t 2 F 3 n
(3)
Cutting parameter
Limit lower
( )
Low (-1)
Basic (0)
High (+1)
Limit upper
( )
Variables
t
2.25
3
6
9
9.75
x1
F
575
600
700
800
825
x2
n
3800
4000
4800
5600
5800
x3
Vc
95.5
100.5
120.6
140.7
145.7
x3
Cutting parameter
Limit lower
( )
Low (-1)
Basic (0)
High (+1)
Limit upper
( )
Variables
t
2.25
3
6
9
9.75
x1
F
575
600
700
800
825
x2
n
3800
4000
4800
5600
5800
x3
Vc
95.5
100.5
120.6
140.7
145.7
x3
Where,
t0, F0
and n0
are the cutting parameters at the
basic level. t, F and n are the range of the cutting parameter variation. The regression equation is rewritten according to the cutting parameters as follows
(t t ) (n n )
Y 0.02365 0.02961 0 0.01234 0
Saiso t n
0.018456 ( t t0 ) (F F0 ) 0.01521 ( F F0 ) (n n 0 )
t F F n
(t t ) 2
0.01989 0
t
(4)
where, t 6; t 3;F 700; F 100;n
4800 ,
0 0 0
n 800
and limited values
2.247 t 9.753(mm ) ;
Fig. 3. Experimental matrix table
-
Collect experimental results
The finished part is put on the CMM gauge to measure the diameter size. Each hole was measured 3 times in diameter at three positions 120 degrees apart on 2 positions 3mm and 6mm from the hole mouth. The value of each hole diameter obtained is the average of the measurements. Accordingly, the
575 F min); 3800 n min) .
-
Optimization of cutting parameters
Objective: it is necessary to find the optimal technological parameters to ensure that the machining size error is the smallest.
1 2 3
1 2 3
Boundary conditions include the limit value of variables and the limit value of the objective function. The algorithm is built on the basis of the following conditions
real 0
real 0
error of pocket diameter is determined as Err Y Y . In
1.215 x , x , x
1.215 , respectively limit the
which, Y real is the experimental diameter value, Y 0
is the nominal diameter value (Tab III).
TN
Y 0(mm)
Yreal (mm )
Err(mm )
1
22
21.999996
-0.000004
2
22
21.952938
-0.047062
3
22
22.037531
0.037531
4
22
21.953934
-0.046066
5
22
22.034254
0.034254
6
22
22.047239
0.047239
7
22
22.048250
0.048250
8
22
21.950125
-0.049875
9
22
22.040890
0.040890
10
22
21.951627
-0.048373
11
22
22.018668
0.018668
12
22
22.020412
0.020412
13
22
22.026044
0.026044
14
22
22.025781
0.025781
15
22
22.029272
0.029272
TN
Y 0(mm)
Yreal (mm )
Err(mm )
1
22
21.999996
-0.000004
2
22
21.952938
-0.047062
3
22
22.037531
0.037531
4
22
21.953934
-0.046066
5
22
22.034254
0.034254
6
22
22.047239
0.047239
7
22
22.048250
0.048250
8
22
21.950125
-0.049875
9
22
22.040890
0.040890
10
22
21.951627
-0.048373
11
22
22.018668
0.018668
12
22
22.020412
0.020412
13
22
22.026044
0.026044
14
22
22.025781
0.025781
15
22
22.029272
0.029272
TABLE III. EXPERIMENTAL RESULTS
22mm
technological parameters are given as
95.5 V 145.7(m min) ;
c
3800 n 5800(rev min) 575 F 825(m m min)
2.25 t 9.75(mm )
(5)
95.5 V 145.7(m min) ;
c
3800 n 5800(rev min) 575 F 825(m m min)
2.25 t 9.75(mm )
(5)
Point to note, since this is an objective function of dimensional accuracy, machined dimensions always have a tolerance. The condition for the objective function value should be set so that finding the minimum value must be within the allowable dimensional tolerance. Suppose, in this case, set the round hole diameter dimensional tolerance to D 22 0.02(mm ) . This means the error objective function
value must meet condition 0.02 Err 0.02(mm ) .
Mathematically, condition 0.02 Err 0.02(mm ) is
To evaluate the accuracy of the diameter when HSFM of the pocket, a regression equation for error of pocket diameter
assumed to determine the minimum value
Errmin 0.02(mm) .
Fig. 4. Verification results on ANOVA/DESIGN EXPERT software
Technologically, the error value is smallest when it approaches 0. Therefore, in this study, the problem has been
1 2 3
1 2 3
reduced to the form: find 1.215 x , x , x 1.215
such
that Errmin
0.02(mm) . The objective function is nonlinear
and conditional, so the GA [22] is used and is described in Tab. IV.
TABLE IV. GA PARAMETERS
No
Parameters
Symbols
Value
1
Number of generations
MaxGen
50
2
Initial population size
npop
100
3
Random selection rate
Se
0.5
4
Mutation probability
Mu
0.05
Optimal results
Fig. 5. Effect of depth of cut and feed rate
The minimum value Errmin 0.02(mm)
corresponds to
Corresponding to the optimal value
x3 0.6671 , the
1
1
2
2
3
3
x 0.772 , x 0.4172 , and x 0.6671 . These
dimensional error equation reflecting the influence of depth of cut and feed rate has the form as follows
Err 0.015418 0.02961x
1
0.018456x x 0.01014x 0.01989x 2
1 2 2 1
(6)
Err 0.015418 0.02961x
1
0.018456x x 0.01014x 0.01989x 2
1 2 2 1
(6)
optimal values correspond to the cutting parameters
t 3.68(mm ), F 658.3(mm ph), n 5534(vg ph)
and
c
c
V ph) .
-
Results and Discussion
Evaluation of the effect of depth of cut ( t ) and feed speed ( F )
As shown in Fig. 5, the error value increases in the negative direction as the depth of cut is decreased (because the error is gradually approaching 0 it is the best, but it will never reach the ideal 0). The error value approaches 0 when keeping the feed rate constant and gradually increasing the cutting depth but only increasing to a moderate extent (near the 0 level), further increasing this parameter will increase the error. Increasing the feed rate value does not have much effect on the dimensional error.
Evaluation of the effect of depth of cut ( t ) and cutting speed (Vc )
For the optimal value of
x2 0.4172 , the dimensional
error equation reflecting the influence of cutting depth and cutting speed has the form as follows
Err 0.02365 0.03731x 1 0.006x 0.01989x 2 3 1 |
III. CONCLUSIONS (7) In summary, the influence of cutting parameters on dimensional accuracy through the dimensional error when |
Fig. 6. Effect of depth of cut and cutting speed
Based on Fig. 6, the change in cutting speed does not have much effect on the dimension error. The depth of cut has a great influence. Similar to Fig. 6, the error decreases with a moderate increase in the depth of cut, but continues to increase, the error also increases.
Evaluation of the effect of feed speed ( F ) and cutting speed (Vc )
1
1
Corresponding to the optimal value x 0.772 , the
dimensional error equation reflecting the influence of cutting depth and cutting speed has the form as follows
Fig. 7. Effect of the feed rate and cutting speed
Fig. 7 shows that, when feed rate and cutting speed go to the average level, the error also gradually approaches 0. The minimum or maximum cutting speed and the maximum feed speed create the largest error.
high-speed finishing milling of alloy steel SKD11 after heat treatment has been considered. The optimal cutting parameters are lso found according to the constraint condition of the variables and the objective function. Some evaluation results show that the smaller the depth of cut, the larger the error. Conversely, increasing the depth of cut to a reasonable value may reduce the error, but should not increase it too much. Feed speed and cutting speed have little influence on dimensional error. Note that if the depth of cut is constant, then with high feed rates, the maximum or minimum cutting speeds in the limit region will produce the largest error. These results have contributed positively to the assessment of the influence of cutting parameters on dimensional accuracy, serving as a basis for selecting appropriate cutting parameters when high-speed machining of hardened alloy steel with dimensional accuracy on request.
REFERENCES
-
H. Schulz, The History of High-Speed Machining, Revista de Ciencia and Tecnologia, 13, 1999, pp. 9-18.
-
X. Cui, J. Zhao, Cutting performance of coated carbide tools in high- speed face milling of AISI H13 hardened steel, Int J Adv Manuf Technol, 2014, pp. 18111824.
-
S. W. Kim, Evaluation of machinability by cutting environments in high-speed milling of difficult-to-cut materials, Journal of Materials Processing Technology, 111, 2001, pp 256-260.
-
Z. Zhao, Y. Xiao, Y. Zhu, Bai Liu, Influence of Cutting Speed on Cutting Force in High-speed Milling, Advanced Materials Research, 139-141, 2010, pp. 835-838.
Err 0.01107 0.01425x
2
0.01234x 0.01521x x
3 2 3
(8)
Err 0.01107 0.01425x
2
0.01234x 0.01521x x
3 2 3
(8)
-
A.N. Fang, P. S. Pai, N. Edwards, A comparative study of high-speed machining of Ti6Al4V and Inconel 718 – part I: effect of dynamic tool edge wear on cutting forces, Int J Adv Manuf Technol, 2013.
-
Y. Karpat, Analytical and Thermal Modeling of High-Speed Machining with Chamfered Tools, Journal of Manufacturing Science and Engineering, Vol. 130 / 011001, 2008, pp. 01-15.
-
M. Iqbal, M. Konneh, M. H. Bin, K. A. Abdallah, M. F. B. Binting, Cutting temperature in high-speed milling of silicon carbide using diamond coated tool, International Journal of Mechanical and Production Engineering, 2015, pp. 62-66.
-
Z. Kang, S. Honghua, H. Linjiang, L. Yingzhi, Vibration control of HSM of thin-wall titanium alloy components based on finite element simulation, Materials Science Forum, 2016, pp. 304-310.
-
M. Sekar, Machining Chatter Analysis for High-Speed Milling Operations, IOP Conf. Series: Materials Science and Engineering, 247, 2017, pp. 1-12.
-
R. B. Silva, A. R. Machado, E. O. Ezugwu, J. Bonney, W. F. Sales, Tool life and wear mechanisms in high-speed machining of Ti-6Al-4V alloy with PCD tools under vaious coolant pressure, Journal of Materials Processing Technolory, 213, 2013, pp. 1459-1464.
-
X. Tian, J. Zhao, Jiabang Zhao, Z. Gong, Y. Dong, Effect of cutting speed on cutting forces and wear mechanisms in high-speed face milling of Inconel 718 with Sialon ceramic tools, Int J Adv Manuf Technol, 2013.
-
R. S. Pawade, Suhas S. Joshi, Multi-objective optimization of surface roughness and cutting forces in high-speed turning of Inconel 718 using Taguchi grey relational analysis (TGRA), Int J Adv Manuf Technol, 56, 2011, pp. 47-62.
-
SU Honghua, LIU Peng, FU Yucan, XU Jiuhua, Tool Life and Surface Integrity in High-speed Milling of Titanium Alloy TA15 with PCD/PCBN Tools, Chinese Journal of Aeronautics, 25, 2011, pp. 784- 790.
-
A. S. Shouckry, The effect of cutting conditions on dimensional accuracy, Wear, 80, 1982, pp. 197-205.
-
N. R. Dhar, Machining of AISI 4140 steel under cryogenic cooling- tool wear, surface roughness and dimensional deviation, Journal of Materials Processing Technology, 123, 2002, pp. 483-489.
-
K.A. Risbood, Prediction of surface roughness and dimensional deviation by measuring cutting forces and vibrations in turning process, Journal of Materials Processing Technology, 132, 2003, pp. 203-214.
-
T. L. Schmitz, Part Accuracy in High-Speed Machining: Preliminary Results, MSEC2006-2110.
-
N. R. Dhar, Cutting temperature, tool wear, surface roughness and dimensional deviation in turning AISI-4037 steel under cryogenic condition, International Journal of Machine Tools & Manufacture, 47, 2007, pp. 754-759.
-
H. H. Shahabi, M. M. Ratnam, Prediction of surface roughness and dimensional deviation of workpiece in turning: a machine vision approach, Int J Adv Manuf Technol, 27, 2010, pp. 213226.
-
A. Dadgari, D. Huo, D. Swailes, The effect of machining toolpath on surface roughness and dimensinal accuracy for high-speed micro milling, Solid State Phenomena, 261, 2017, pp. 69-76.
-
S. Yousefi, M. Zohoor, M. Faraji, The variations of dimensional accuracy in dry hard turning operation, The 25th Annual International Conference on Mechanical Engineering ISME2017-2352 ISME2017, Tarbiat Modares university, Tehran, Iran, 2017, pp. 415-416.
-
Bies, Robert R.; Muldoon, Matthew F.; Pollock, Bruce G.; Manuck, Steven; Smith, Gwenn; Sale, Mark E. (2006). "A Genetic Algorithm- Based, Hybrid Machine Learning Approach to Model Selection". Journal of Pharmacokinetics and Pharmacodynamics. 33 (2): 196221.