
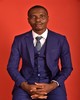
- Open Access
- Authors : Ifeanyichukwu U. Onyenanu, Miracle C. Ogbogu, C. J. Nwadiuto
- Paper ID : IJERTV13IS080031
- Volume & Issue : Volume 13, Issue 08 (August 2024)
- Published (First Online): 18-08-2024
- ISSN (Online) : 2278-0181
- Publisher Name : IJERT
- License:
This work is licensed under a Creative Commons Attribution 4.0 International License
Performance Optimization of an Improved Biomass Gasifier Charcoal Stove using Response Surface Method (RSM)
Ifeanyichukwu U. Onyenanu (Phd), Miracle C. Ogbogu, C. J. Nwadiuto
Department of Mechanical Engineering, Chukwuemeka Odumegwu Ojukwu University, Uli Nigeria.
Abstract
Biomass is widely used as a cooking fuel in many developing countries due to limited access to modern energy sources. Traditional biomass stoves are often inefficient and emit high levels of harmful pollutants. This study aimed to optimize the performance of an improved biomass gasifier charcoal stove designed to address these issues. A prototype stove developed at NUTABOLTS Technologies LTD was tested using response surface methodology (RSM) with thermal efficiency and time to boil 5kg of water as response variables. Key design parameters investigated included fan control level, charcoal weight, and charcoal type. Experiments were conducted according to an I-optimal design and responses were modeled using ANOVA. Results showed fan control and charcoal weight significantly affected efficiency and boiling time. Optimal settings were found to be a fan control of 3.1, charcoal weight of 0.65kg, and use of natural lump charcoal, achieving an efficiency of 40.2% and boiling time of 11.6 minutes. The strong correlation between predicted and actual results validated the developed models. This study demonstrates how RSM can be employed to optimize combustion stove performance for improved fuel use and reduced emissions.
Keywords: Stove, Biomass stove, Charcoal Stoves, Response Surface Method, Optimization
INTRODUCTION:
In Africa, renewable energy holds its position as the primary source of energy, and within this domain, wood resources play a significant role in the realm of biomass energy. While constituting a mere 10% of the global primary energy supply, wood fuel plays a pivotal role in the daily lives of approximately 2.8 million people who depend on it for cooking and heating (Piabuo et al., 2020).
Domestic cooking, a cornerstone of daily existence worldwide, stands not only as an essential aspect of daily life but also as a fundamental requirement for a healthy lifestyle that enhances human sustenance. Globally, around
2.8 million people rely on solid wood fuel as their primary energy source, with developing nations accounting for over 90% of this demographic. Remarkably, in Sub-Saharan Africa, nearly 70% of households depend on wood fuel for their energy needs (Piabuo et al., 2020). Despite abundant energy resources, an estimated 84% of Nigerian households lack access to decent cooking and lighting fuels (Cunha, 2019). Most rural houses lack access to gas or electricity, oil is scarce, and kerosene can only be used by a privileged few, largely in metropolitan areas (FAO, 2023).
There has been a strong call globally for the adoption of safe, clean, and environmentally friendly fuels, and quite several efforts currently drafted for the development of clean fuels regarding the human and environmental health association with some fuels (Okedere et al., 2022), up to two-thirds of the population of developing countries over the years still rely primarily on bio-fuels for domestic cooking, frying, roasting, and other uses. Above seventy percent of Nigerias population depends solely on the cookstove which is the most popular and widespread in both urban and rural communities among the various technologies introduced for efficient household heating and cooking methods (Chanchangi et al., 2023).
However, the utilization of biomass as a source of energy has long been a critical component of sustainable energy systems, particularly in developing nations where access to conventional energy sources is limited or impractical. Among the various technologies developed to harness the energy potential of biomass, biomass gasification stoves have emerged as a promising solution. These stoves offer a cleaner and more efficient way to convert biomass materials, such as wood, agricultural residues, and charcoal, into useful energy forms, such as heat and gas.
Traditional open-fire cooking methods using biomass fuels not only contribute to deforestation and environmental degradation but also pose significant health risks due to the release of harmful pollutants, including particulate matter and carbon monoxide. Biomass gasifier charcoal stoves represent a technological advancement aimed at addressing these challenges by improving combustion efficiency, reducing emissions, and enhancing overall cooking performance.
Performance optimization of an improved biomass stove is important to maximize fuel efficiency, minimize pollutant emissions, and increase cooking performance. Improved stoves aim to reduce fuel consumption by up
to 50% compared to traditional open fires through better combustion air control and heat retention (Ahmed & Iqbal, 2018). Key parameters like combustion chamber geometry, air inlets, heat shields, etc. need to be optimized to achieve the stove’s design objectives. Response Surface Methodology (RSM) is a widely used optimization technique that relates the response variable (stove performance metrics) to the design variables through statistical experimental design techniques (Erhimona et al., 2023). This research aims to use RSM to optimize the performance of an improved biomass stove by studying the effect of key design variables on fuel efficiency and time taken to boil water.
Literature Review
Biomass is a widely used fuel for cooking and heating in developing countries. Traditional stoves have thermal efficiencies as low as 5-15% with high levels of pollutant emissions (Khan et al., 1995). Studies have shown that indoor air pollution from traditional biomass stoves contributes significantly to health issues like pneumonia, chronic obstructive pulmonary disease, and lung cancer (WHO, 2023). Improved biomass stoves aim to address these issues through more advanced combustion technology. Stove performance depends on factors like fuel properties, combustion chamber design, air flow rate, and heat transfer design. Optimization of these parameters is necessary to maximize efficiency and increase cooking time. Several studies have used experimental design techniques like RSM to optimize stove designs. Komolafe & Awogbemi, (2010) worked on fabrication and performance evaluation of an improved charcoal cooking stove. However, from the study, the thermal efficiency value of 34% was obtained for the improved stove. Ndécky et al., (2018) studied the application of statistical design of experiments to performance analysis of charcoal cook stoves. They used Fischers statistical test (F- test) and Newman-Keuls test, to determine the effect of fuel type, fuel initial load, secondary air inlet, and ventilation on thermal efficiency and CO emission of two biomass fire cookstoves during boiling or simmering. Kyuvi, (2022) researched on optimization of ceramic insulation of cooking stoves using carbonized organic waste. Kshirsagar & Kalamkar, (2020) worked on the application of multi-response robust parameter design for performance optimization of a hybrid draft biomass cook stove. The study utilized RSM models for robust parameter optimization of the stove performance to maximize efficiency and minimize emissions and propagation of error. Abasiryu et al., (2016) worked on Performance Evaluation of Some Locally Fabricated Cookstoves in Mubi, Adamawa State, Nigeria. Natrayan et al., (2023) developed a study on the optimization process of potassium carbonate-activated carbon through jute-based core materials by using an artificial neural network with esponse Surface Methodology. Thus, this study is aimed at the performance optimization of an improved biomass gasifier charcoal stove using RSM.
3. MATERIALS AND METHODS
Material Specification
In the study, a prototype of an improved biomass stove developed by NUTABOLTS Technologies Limited was utilized (as shown in Figure 1). This stove consists of various components, including the stove body, liner, combustion chamber, insulator, grate, stove base, handle, stove top, liner base, stove stand, fan, fan housing, air inlet fan circuit, and battery. The stove body possesses specific dimensions, with a height of 300mm, a diameter of 250mm, and a thickness of 0.8mm. The combustion chamber within the stove is constructed from 22-gauge stainless steel, featuring dimensions of 202mm in height, 122mm in diameter, and a thickness of 1mm. To facilitate primary air intake, a fan measuring 92x92x25mm is used, directing airflow through a circular channel port with a 75mm diameter situated at the bottom front of the stove. Additionally, a fixed cylindrical insulation panel, comprised of briquette rice husk ash and a binder, sized 249 x 210 x 53.5mm, is affixed to the sides and top of the combustion chamber. Figure 1 provides a visual representation of the 3D CAD model created using SOLIDWORKS Software version 2021.
Figure 1: 3D model drawing of the gasifier charcoal stove
Stove Fabrication
(a)
(b)
NUTABOLTS Technologies Limited, located in Enugu State, Nigeria, constructed the biomass gasifier charcoal stove. Figures 2a, 2b and 2c illustrate various stages of the fabrication process that the gasifier stove underwent during its manufacturing. Table 1 provides a detailed description of the materials used for each part of the stove.
(c)
Figure 2: (a) Casting and adding the composite mixtures to the top and side of the stove. (b) fabrication of the combustion chamber (c) marking out of the primary air intake channel.
Table 1: Materials used for the gasifier stove
S/N | Parts | Material | Description |
1 | stove body | Mild steel | 0.5mm thickness |
2 | liner | Stainless steel | 1mm thickness |
3 | combustion chamber | Stainless steel | 1mm thickness |
4 | Insulator | Briquetted rice husk ash and
binder(cement) |
53.5mm thickness |
5 | stove base | Mild steel | 1mm thickness |
6 | handle | Mild steel | 1mm thickness |
7 | stove top | Mild steel | 1mm thickness |
8 | liner base | Stainless steel | 1mm thickness |
9 | fan | Plastic | 3.5E-230HB High-temperature AC
Axial cooling fan |
10 | fan housing | Mild steel | 1mm thickness |
11 | battery | Lithium | 3.7V Rechargeable battery |
12 | grate | Mild steel | 1mm thickness |
13 | Stove stand | Stainless steel | 1mm thickness |
14 | Air intake channel | Mild steel | 1mm thickness |
15 | Ashtray | Mild steel | 1mm thickness |
Sample Preparation/Experimental Setup
In this study, four distinct types of charcoal samples were employed: coconut charcoal, activated charcoal, natural lump charcoal, and briquettes. To ensure fair comparisons, the quantities of these charcoal samples were adjusted based on their respective weight variations, as detailed in Table 2. To assess the thermal efficiency and cooking performance of the biomass gasifier stove under controlled conditions, the fan speed was regulated accordingly. The experimental setup featured several essential instruments, including a digital weighing scale and thermocouples. To capture crucial response variables, such as temperature readings at various locations within the gasifier stove, including the cooking surface, bottom, and sides, K-type thermocouples were used. Additionally, the digital weighing scale was used to measure the weight of the different charcoal samples accurately.
Experimental Design
To achieve the results, we utilized a specialized randomized design called I optimal design, which is derived from the surface response method (RSM). I optimal designs, also known as IV or Integrated Variance designs aim to minimize prediction variance, in the region (Erhimona et al., 2023). This is crucial for predictions in response surface methods (RSM). The algorithm selects points that minimize prediction variance across the design space (Erhimona et al., 2023). In our study, we focused on optimizing the performance of the biomass charcoal stove. Measured three performance attributes as responses, in our experimental design. We created a design table consisting of 17 runs using Design Expert version 13.
Therefore, the performance parameters were derived from the design table, as presented in Table 2
- Response 1: Efficiency (%)
- Response 2: Time taken to Boil 5kg of water (sec)
Again, three primary factors that affect the performance of the stove vary as shown:
1. 1 A (Fan control level) 4 .@ (4 levels)
- 0.1Kg B (Mass of charcoal in the combustion chamber) 1Kg .@ (4 levels)
- C (Type of Charcoal-Activated, Coconut shell, Natural lump, briquettes) .@ (4 levels)
Table 2. Experimental Design and Response Results
Factor 1 Factor 2 Factor 3 Response 1 Response 2 Run A: Fan control value B: Weight of the charcoal C: Type of Charcoal Efficiency Time taken to boil 5kg of water Kg % Mins 1 3 1 Coconut Shell 39.1 10.8 2 3 0.5 Coconut Shell 38 12 3 4 0.5 Activated Charcoal 40 11 4 4 0.8 Briquette 46 10 5 2 0.8 Natural lump charcoal 38 13 6 3 0.1 Natural lump charcoal 39 11.8 7 2 0.1 Activated Charcoal 36 14.3 8 4 0.1 Coconut Shell 43 9.5 9 3 0.1 Natural lump charcoal 39 11.8 10 3 1 Activated Charcoal 37 13 11 1 0.1 Natural lump charcoal </td
38.2 14.7 12 2 0.8 Natural lump charcoal 38 13 13 1 1 Briquette 38.5 14.7 14 2 0.5 Briquette 38.5 13.7 15 4 1 Natural lump charcoal 45.7 10.3 16 4 0.1 Activated Charcoal 40 11.7 17 1 0.8 Activated Charcoal 40 15 RESULTS
Analysis of Variance (ANOVA)
Analysis of variance (ANOVA) comprises a set of statistical models and related estimation techniques. It’s used to examine variations both within and between groups, especially when analyzing differences in group means within a sample. ANOVA was employed in this study to determine if there was a noteworthy difference in the experiment’s mean values. Tables 3 and 4 showcase the outcomes of ANOVA concerning the thermal efficiency and the cooking performance, derived from the experimental data. These tables detail the statistical significance of the factors under consideration and also provide information about the coefficients of the respective models.
Source Sum of Squares df Mean Square F-value p-value Model 151.29 14 10.81 38.97 0.0004 significant A-Fan control value 39.60 1 39.60 142.83 < 0.0001 B-Weight of the charcoal 2.09 1 2.09 7.55 0.0405 C-Type of Charcoal 23.76 3 7.92 28.57 0.0014 AB 0.1566 1 0.1566 0.5649 0.4862 AC 14.48 3 4.83 17.41 0.0045 BC 0.3771 3 0.1257 0.4534 0.7263 A² 32.17 1 32.17 116.04 0.0001 B² 0.1720 1 0.1720 0.6202 0.4667 Residual 1.39 5 0.2773 Lack of Fit 1.39 2 0.6932 Pure Error 0.0000 3 0.0000 Cor Total 152.68 19 I: For the thermal efficiency, the ANVOA for the selected factorial model is seen below: Table 3. Analysis of Variance results for thermal efficiency
The F-value of 38.97 indicates the model’s significance, with only a 0.04% chance of noise. P-values below 0.0500 highlight significant terms (A, B, C, AC, A²), while values over 0.1000 are insignificant. Simplifying the model can improve quality, except for hierarchy needs. The R² at 0.9909 shows a strong link between significant terms and thermal efficiency. The Predicted R² of -0.2427 suggests the mean may be a better predictor than the current model, and higher-order models might perform better
II: For the time taken to boil 5kg of water, the ANVOA for the selected factorial model is seen below: Table 4. Analysis of Variance results for the time taken to boil 5kg of water
Source Sum of Squares df Mean Square F-value p-value Model 53.73 14 3.84 19.39 0.0020 significant A-Fan control value 38.60 1 38.60 195.01 < 0.0001 B-Weight of the charcoal 0.3330 1 0.3330 1.68 0.2512 C-Type of Charcoal 5.95 3 1.98 10.02 0.0148 AB 0.0928 1 0.0928 0.4686 0.5240 AC 0.3808 3 0.1269 0.6412 0.6207 BC 0.1954 3 0.0651 0.3290 0.8054 A² 0.2915 1 0.2915 1.47 0.2791 B² 0.0392 1 0.0392 0.1982 0.6748 Residual 0.9898 5 0.1980 Lack of Fit 0.9898 2 0.4949 Pure Error 0.0000 3 0.0000 Cor Total 54.72 19 The Model F-value of 19.39 implies a mere 0.02% chance of noise influence. P-values below 0.0500 identify significant terms (A, C) in the model, while values above 0.1000 denote insignificance. Streamlining the model, except for hierarchy essentials, may improve it if many terms lack significance. The R² of 0.9819 effectively represents the relationship between significant model terms and boiling time for 5kg of water. However, the Predicted R² of -3.1497 suggests that the overall average might be a better predictor than the current model.
Predicted and Actual Results for the two (2) Responses:
- Efficiency
Using the design expert software, the experimental design table was improved through optimisation. For each scenario, coded equations were created that were then used to estimate the expected outcomes of the experiment. Figure 3 displays a graph that compares the predicted values with the actual results from the experiment. The graph confirms a strong resemblance between the predicted and actual values, especially in the case of the thermal efficiency experiment. The exact and predicted data for the charcoal stove’s thermal efficiency were organised in Table 5.
Run Order Actual Value Predicted Value Residual Leverage Internally Studentized Residuals Externally Studentized Residuals Cook’s Distance Influence on Fitted Value DFFITS Standard Order 1 39.10 38.87 0.2316 0.929 1.65 2.187 2.374¹ 7.909¹ 14 2 38.00 38.67 -0.6658 0.413 -1.650 -2.187 0.128 -1.834 13 3 40.00 40.31 -0.3108 0.698 -1.074 -1.095 0.178 -1.665 3 4 46.00 45.84 0.1600 0.981 2.208 12.550² 16.849¹ 90.355¹ 9 5 38.00 37.97 0.0333 0.493 0.089 0.079 0.001 0.078 18 6 39.00 38.98 0.0222 0.497 0.059 0.053 0.000 0.053 16 7 36.00 36.25 -0.2489 0.888 -1.412 -1.629 1.053¹ -4.585¹ 1 8 43.00 42.71 0.2895 0.889 1.650 2.187 1.454¹ 6.190¹ 11 9 39.00 38.98 0.0222 0.497 0.059 0.053 0.000 0.053 17 10 37.00 36.99 0.0122 0.988 0.215 0.193 0.261 1.777 5 11 38.20 38.26 -0.0592 0.978 -0.760 -0.722 1.718¹ -4.827¹ 15 12 38.00 37.97 0.0333 0.493 0.089 0.079 0.001 0.078 19 13 38.50 38.87 -0.3733 0.897 -2.208 -12.550² 2.829¹ -37.026¹ 10 14 38.50 38.22 0.2800 0.442 0.712 0.672 0.027 0.598 8 15 45.70 45.75 -0.0518 0.983 -0.760 -0.722 2.255¹ -5.531¹ 20 16 40.00 39.61 0.3856 0.646 1.230 1.318 0.184 1.780 2 17 40.00 39.84 0.1619 0.962 1.583 2.004 4.259¹ 10.120¹ 4 18 38.50 38.22 0.2800 0.442 0.712 0.672 0.027 0.598 7 19 39.00 38.86 0.1447 0.972 1.650 2.187 6.361¹ 12.946¹ 12 20 45.00 45.35 -0.3466 0.911 -2.208 -12.550² 3.333¹ -40.189¹ 6 Figure 3: Showing the graphical representation of the predicted and actual values for thermal efficiency Table 5: Showing the actual and predicted data sets of the thermal efficiency
- Time taken to boil 5Kg of water
The visual representation in Figure 4 illustrates the comparison between the predicted and actual outcomes of the experiment measuring the time required to boil 5 kg of water. Once again, this graph emphasizes a strong resemblance between the projected and observed values, particularly in the context of this experiment. The concrete datasets encompassing both the real results and the projected values for the physical tests related to thermal performance have been meticulously organized in Table 6. This table serves as a valuable reference, offering a comprehensive insight into the experiment’s findings and results.
Figure 4: Showing the predicted and actual values for the time taken to boil 5Kg of water Table 6: Showing the actual and predicted data sets of the time taken to boil 5kg of water
Run Order Actual Value Predicted Value Residual Leverage Internally Studentized Residuals Externally Studentized Residuals Cook’s Distance Influence on Fitted Value DFFITS Standard Order 1 10.80 11.05 -0.2507 0.929 -2.114 -5.809 3.898¹ -21.008¹ 14 2 12.00 11.28 0.7208 0.413 2.114 5.809 0.210 4.872¹ 13 3 11.00 11.30 -0.2957 0.698 -1.209 -1.286 0.225 -1.955 3 4 10.00 10.05 -0.0457 0.981 -0.747 -0.708 1.926¹ -5.100¹ 9 5 13.00 13.08 -0.0819 0.493 -0.258 -0.233 0.004 -0.229 18 6 11.80 11.85 -0.0546 0.497 -0.173 -0.155 0.002 -0.154 16 7 14.30 14.43 -0.1263 0.888 -0.848 -0.820 0.380 -2.307 1 8 9.50 9.81 -0.3134 0.889 -2.114 -5.809 2.387¹ -16.441¹ 11 9 11.80 11.85 -0.0546 0.497 -0.173 -0.155 0.002 -0.154 17 10 13.00 12.91 0.0890 0.988 1.852 2.957 19.367¹ 27.210¹ 5 11 14.70 14.55 0.1455 0.978 2.210 13.066² 14.548¹ 87.324¹ 15 12 13.00</> 13.08 -0.0819 0.493 -0.258 -0.233 0.004 -0.229 19 13 14.70 14.59 0.1066 0.897 0.747 0.708 0.323 2.090 10 14 13.70 13.78 -0.0800 0.442 -0.241 -0.216 0.003 -0.193 8 15 10.30 10.17 0.1273 0.983 2.210 13.066² 19.101¹ 100.060¹ 20 16 11.70 11.42 0.2785 0.646 1.052 1.066 0.134 1.439 2 17 15.00 14.95 0.0545 0.962 0.631 0.588 0.677 2.970¹ 4 18 13.70 13.78 -0.0800 0.442 -0.241 -0.216 0.003 -0.193 7 19 13.80 13.96 -0.1567 0.972 -2.114 -5.809 10.443¹ -34.388¹ 12 20 10.70 10.60 0.0990 0.911 0.747 0.708 0.381 2.268 6 Effect of the investigated biomass gasifier charcoal stove parameters on the Response:
- Efficiency
The result generated (Figure 5), shows the contour diagram of the relationship between three (3) biomass gasifier variables, namely fan control value (A), weight of the charcoal (B), Activated charcoal (C), and the thermal efficiency. The contour plot reveals an interesting interaction between the weight and size of the charcoal about thermal efficiency. When the charcoal’s weight increases and its size decreases simultaneously, the efficiency of the charcoal stove also increases. This means that as the charcoal becomes heavier and smaller, the stove performs better in terms of efficiency. Figure 6 shows the 3D diagram of the relationship between three (3) variables to thermal efficiency.
Figure 5: Contour plots of the response (efficiency)
Fig. 6: 3D diagram of the relationship between three (3) variables to the thermal efficiency
- Time taken to boil 5Kg of water
- Efficiency
Figure 7 illustrates a similar analysis, but this time it focuses on the duration required to boil 5 kilograms of water. The results displayed in Figure 7 depict a contour diagram representing the relationship among three key biomass gasifier variables: fan control value (A), charcoal weight (B), and activated charcoal (C), concerning the time taken to boil 5 kilograms of water. The results reveal that both the fan control value (A) and the weight of the charcoal (B) have a significant impact on reducing the time required to boil this amount of water. This suggests that an increase in both charcoal weight and fan control level leads to a decrease in the time needed for water to reach its boiling point. Figure 8, on the other hand, presents a three-dimensional diagram illustrating the relationship among these three variables and the time required to boil 5 kilograms of water
Fig. 7: Contour plots of the response (Time taken to boil 5Kg of water)
Fig. 8: 3D diagram of the relationship between three (3) variables to the Time taken to boil 5Kg of water
Desirability Plot
From the solution of the combination of the 3 categoric factor levels, the selected or optimal values were found to be Fan control value (3.1), the weight of the charcoal (0.65), Charcoal type (Natural lump), which will give an efficiency of 40.262% and Time taken to boil 5Kg of water to be 11.579 minutes.
Fig 9: Optimal Desirability plot
CONCLUSION
This study aims to use RSM to optimize the performance of an improved biomass stove by studying the effect of key design variables on fuel efficiency and time taken to boil water. For the optimization procedure, the I-optimal design (RSM) was employed. In both responses, the actual and predicted values had a high similarity, particularly in the context of this experiment. Furthermore, the regression coefficient (R2) values revealed a significant agreement between the experimental and anticipated values for the optimization. The ideal settings were discovered to be Fan control value (3.1), charcoal weight (0.65), Charcoal type (Natural lump), and time taken to boil 5 kg of water (11.579 minutes).
RECOMMENDATION
Additional research should explore the possibility of identifying improved materials for components like the insulator and combustion chamber, to potentially enhance both the thermal efficiency and the lightweight technology of the charcoal stove. Furthermore, it would be beneficial to investigate the introduction of a high- speed fan into the charcoal stove to increase its thermal efficiency further.
REFERENCES
Abasiryu, T., Ayuba, A., & Zira, A. E. (2016). Performance evaluation of some locally fabricated cookstoves in Mubi, Adamawa State, Nigeria. Nigerian Journal of Technology, 35(1), 4853.
Ahmed, N., & Iqbal, Z. (2018). Benefits of Improved Cook Stoves: Evidence from Rural Bangladesh. The Bangladesh Development Studies, 41(4), 127.
Chanchangi, Yusuf. N., Adu, F., Ghosh, A., Sundaram, S., & Mallick, Tapas. K. (2023). Nigerias energy review: Focusing on solar energy potential and penetration. Environment, Development and Sustainability, 25(7), 57555796. https://doi.org/10.1007/s10668-022-02308-4 Cunha, J. D. (2019, February 20). SEFA to help scale up innovative clean cooking business in Nigeria [Text]. Banque africaine de développement – Faire la différence; African Development Bank Group. https://www.afdb.org/fr/news-and-events/sefa-to-help-scale-up- innovative-clean-cooking-business-in-nigeria-13842
Erhimona, G. O., Onyenanu, I. U., & Utu, O. (2023). Optimization of a locally Fabricated Palm Fruit Digester using the Response Surface Method (RSM). International Journal of Engineering Research, 12(07).
FAO. (2023). The fuelwood problem. https://www.fao.org/3/q4960e/q4960e02.htm
Khan, A., Eusuf, M., Prasad, K. K., Moerman, E., Cox, M., Visser, A. M. J., & Drisser, J. A. J. (1995). The development of improved cooking stoves adapted to the conditions in Bangladesh. Final Report of Collaborative Research Project between IFRD, BCSIR, Bangladesh, and Eindhoven University of Technology, Eindhoven, the Netherlands.
Komolafe, C. A., & Awogbemi, O. (2010). Fabrication and performance evaluation of an improved charcoal cooking stove. The Pacific Journal of Science and Technology, 11(2), 5158.
Kshirsagar, M. P., & Kalamkar, V. R. (2020). Application of multi-response robust parameter design for performance optimization of a hybrid draft biomass cook stove. Renewable Energy, 153, 11271139.
Kyuvi, E. M. (2022). Optimization of Ceramic Insulation of Cooking Stoves Using Carbonized Organic Waste [PhD Thesis]. University of Nairobi.
Natrayan, L., Niveditha, V. R., Kaliappan, S., Patil, P. P., Pandian, C. K., Rao, Y. S., & Murugan, P. (2023). Optimization Process of Potassium Carbonate Activated Carbon through Jute-Based Core Materials by Using Artificial Neural Network with Response Surface Methodology. Adsorption Science & Technology, 2023.
Ndécky, A., Gamache, S., Barro, F.I., & Youm, I. (2018). Application of statistical design of experiments to performance analysis of charcoal cooks stoves. International Journal of Clean Coal and Energy, 7(3), 3957.
Okedere, O. B., Oyelami, S., Oyewole, K. A., Rabiu, K. O., Lasisi, H. O., Atanda, N., & Omojola, S. (2022). A Comparative Investigation of the Impact of Two Different Charcoal Stove Configurations on Polycyclic Aromatic Hydrocarbons and Particulate Matter Emissions. Journal of Energy and Safety Technology (JEST), 5(1), 5361.
Piabuo, S. M., Puatwoe, J. T., Piabuo, S. M., & Puatwoe, J. T. (2020). Public Health Effects of Wood Fuel in Africa: Bioenergy from Tree Commodities as a Sustainable Remedy. In Public Health in Developing CountriesChallenges and Opportunities. IntechOpen. https://doi.org/10.5772/intechopen.90603
WHO. (2023). Household air pollution. Household Air Pollution. https://www.who.int/news-room/fact-sheets/detail/household-air-pollution- and-health