
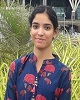
- Open Access
- Authors : Himal Chitara , Prof. Raksha K Patel , Prof. Tejas V Bhatt
- Paper ID : IJERTV9IS040090
- Volume & Issue : Volume 09, Issue 04 (April 2020)
- Published (First Online): 09-04-2020
- ISSN (Online) : 2278-0181
- Publisher Name : IJERT
- License:
This work is licensed under a Creative Commons Attribution 4.0 International License
Prediabetic Condition of Patient Detection System using Fuzzy Set Theory
Himal Chitara
Dept. of Biomedical Engineering
U. V. Patel College of Engineering Ganpat University
Kherva, Mehsana, Gujarat, India
Prof. Raksha K Patel
Dept. of Biomedical Engineering
U. V. Patel College of Engineering Ganpat University
Kherva, Mehsana, Gujarat, India
Prof. Tejas V Bhatt
Dept. of Biomedical Engineering
-
V. Patel College of Engineering Ganpat University
Kherva, Mehsana, Gujarat, India
Abstract In the current era, the diabetes mellitus which causes of unbalanced diet, busy schedule and standard of living. The main reason to produce diabetes is either deficiency to produce insulin or deficiency in use of insulin by the specific tissues. Pre-deterministic method is to diagnose the risk assessment of diabetes to patient. The main purpose is fuzzy set theory used for prediction and forecasting of diabetes diagnostic. An author has certain 6 input parameters such as BMI, RBS, FBS, OGTT, PPBS, Hemoglobin A1c and single output parameter such as pre-diabetic and diabetic conditions for this diagnostic system. This diagnostic system is based on fuzzy rules. Output defines pre-diabetes, or type 1 diabetes or type 2 diabetes conditions helpful for diagnosis procedure in day to day routine.
Keywords Diabetes mellitus, Fuzzy set theory, Prediabetes, Body mass index, Haemoglobin A1c test
-
INTRODUCTION
Diabetes mellitus is one of the most common diseases in the current era[1]. An oldest disease in the world is a diabetes disease. Diabetes is a metabolic disorder characterized by the presence if the chronic hyperglycemia accompanied by greater or lesser impairment in the metabolism of carbohydrates, lipids, and proteins[2]. Pancreas is part of endocrine, produces
2 types of hormones such as insulin and glucagon for balancing blood sugar level. Diabetes is a condition in which either blood sugar is increases due to insufficient secretion of insulin or blood sugar is decreases due to not use of insulin properly. Person which have symptoms like polyuria, polydipsia, polyphagia, vision threatening, weight loss, impaired healing and exhaustion. People with type 1 diabetes cant produces insulin properly and type 2 diabetic patients doses not produce enough insulin[3]. From this disease, patients also suffering from other diseases such as retinopathy, cardiovascular, nephropathy, and neuropathy. Types of diabetes such as type 1, type 2, pre-diabetes, gestational diabetes, diabetes jenuvile and diabetes insipidus[1].
457 million people worldwide suffering from diabetes as per the WHO states[4]. As per the WHO , in 2004, 3.4 million people died from FBS; and in 2015, 457 million people; in 2014, 4.2 million people suffering from diabetic retinopathy. In India, 1lakh children are suffering from diabetes because of unbalanced diet, and living standards. In 2019, as per the newspaper survey 1 lakh children are suffering from diabetes in current era; 1lakh from every 10 children are suffering from
type 1 diabetes. In Chennai 26% childrens are diagnosed by diabetes diseases in 2019. In the current era, old men, children and young aged peoples are suffering from diabetes diseases. If diabetic patients cant cure properly then patient may go in coma. If diabetic patients can give proper treatment at a time then it has a chance to cure sometimes.
Artificial intelligence has subset of fuzzy set theory, machine learning, data science and image processing, and these techniques are developing in current era. It is used in every field such as mechanical, electrical, aerospace and medical applications[5]. For the medical field, easy and accurate technique is fuzzy set theory.
Author has do retrospective study in Ahmedabad city. Diabetes diagnosed in 508 people every month from that 80 people is suffering from this disease.
Fig1: Prevalence of diabetes in ahmedabad.
From the 80 people of diabetic 23people are suffering from diabetes diseases. In 2018-2019, ratio of pre-diabetic patients is 19%, type 1 diabetes is 50% and type 2 diabetes is
31%.
Fig2: Ratio of prediabetes, type 1 and type 2 diabetes in Ahmedabad.
Author has study that in children, young and women find the prediabetes, and diabetic conditions. In children because of their living standards, unbalanced diet and inactivity in daily routine so that it may find out the prediabetes more n more seen and also type 1 seen more n more and if they are not treated then type 2 diabetes is also seen. In ahmedabad, 22.08% prediabetes, 60.07% diabetes prevalence shown.
Fig3: Prevalence of dibates in children, men, and women.
-
DIABETES DIAGNOSTIC TECHNIQUES Person who has experience symptoms of diabetes such as polyphagia, polydipsia, polyuria, weight loss, vision threatening, etc. are diagnosed by different methods.
-
Blood Glucose Measuremnt
Doctor can use blood glucose level using hospital glucometer or continuous glucometer. Diabetes and prediabetes diagnose blood test for random blood sugar, fasting blood sugar and postprandial blood sugar. In laboratory test it is diagnosed. The range of random blood sugar normal values is less than 100mg\dl. Fasting blood sugar ranges are normal is less than 100mg\dl, prediabetes is 100 to 125mg\dl and diabetes is 126 mg\dl and above. Post prandial blood sugar test takes after 2hours of meal. PPBS ranges are normal is 140mg\dl, prediabetes is 180mg\dl and diabetes is 180mg\dl and above.
In women, blood sugar level ranges are normal 70 to 140mg\dl, random blood sugar value is 70 to 140mg\dl, normal fasting blood sugar range is 70 to 100mg\dl and PPBS range 2hours after meal is 135 to 145mg\dl and after 1hour of meal is 180mg\dl.
In men, blood sugar level ranges are normal 70 to 140md\dl, random blood sugar value is 70 to 125mg\dl,
fasting blood sugar range is 70 to 100mg\dl and post pardial blood sugar value is 140mg\dl.
In children, blood sugar level normal range is 70 to 100mg\dl, low blood sugar range is below 70mg\dl, and high blood sugar range is over 140mg\dl.
-
Haemoglobin A1c test
Hemoglobin A1c is also known as glycated Hemoglobin or HbA1c test. Iron containing molecule in red blood cells is hemoglobin which is responsible for transporting oxygen around the whole body. Glucose binds permanently with hemoglobin through a process known as glycation. Glucose is attached with hemoglobin is known as glycated hemoglobin, HbA1c or A1c. HbA1c is used for measurement aid for the management of diabetes because red blood cells have life span of 120days for measuring the level of glucose in hemoglobin in our blood that shows the average blood glucose level for the previous 3months or 6 to 8 weeks. From this test patients treatment plan makes better using regular blood glucose monitoring and HbA1c test. The range of HbA1c test are normal value is below 5.6%, prediabetes is 5.7 to 6.4% and diabetes value is 6.55% or above.
-
Glucose Tolerance Test
Oral glucose tolerance test performed in the morning after 8 to 10hours of overnight. It is measured when type 2 diabetes is presented in person. Firstly before not giving glucose, fasting blood test is taken. Then patients gives 75gm glucose drinks in 250 to 300ml of water measuring the blood glucose level. After 2hours blood glucose level will be measured.
-
Body Mass Index
Body mass index is useful for visualizing ranges for normal, underweight, overweight and obesity weights of a persons height. It is very easy tool. Body mass index formla:
BMI (Kg/m2) = mass(Kg)/height(m2) (1)
The range of BMI are normal weight is 18.5 to 24.09, underweight is less than 18.05, over weight is 25.0 to 29.9, obesity is 30 to 39.9 and morbidity obesity is 40 or above.
-
-
PATIENT DETECTION METHOD
Author has made a pre-deterministic method is to diagnose for risk assessment of diabetes to patients. This method is made for patient detection system of diabetes using fuzzy set theory. Laboratory test results are used for data sheet and fuzzy rules based system is made for the detection system. This system diagnosed prediabetes in a person.
-
DATA
From the hospital, collections of 508 medical records are collected for clustering. From the clustering, symptoms, blood glucose level and previous 3months of glucose level in the body is analyses. It results that nin children more prediabetes and type 1 diabetes are shown and in this current era, young people have chances of cardiovascular diseases because of this diabetic condition, and standard of living.
-
FUZZY SET THEORY
The fuzzy set theory designed for patient detection system for diabetic patients. It is easy to excess and gives better
result from the non-experienced person. Author has designs 6 input parameters such as BMI, RBS, FBS, PPBS, HbA1c, and OGTT and single output is prediabetes and diabetes. Fig 4 shows a design flow chart of patient detection system using fuzzy set theory.
Data Set
Data Set
Patient Detection System
Input Data Processing
Input Data Processing
BMI, RBS, FBS, PPBS, HbA1c, OGTT
Fuzzification Process
Fuzzification Process
Membership Function
Fuzzy Rules
The ranges of PPBS membership functions are normal [120 129 135 140], medium [1136 154
161 180], high [173 207 258 300].
-
Oral Glucose Tolerance Test
The ranges of OGTT membership functions are fasting [59.9 74 84.7 100], after 2hours [96.9 113
127 145.4], 1hour [141 161 179 201].
-
HbA1c
The ranges of HbA1c membership functions are normal [3 3.72 4.587 5.47], medium [5.32 5.64
6.06 6.4], high [6.22 6.88 7.37 8.01].
Fig6: Input data processing for BMI parameters.
-
Output
The ranges of output are prediabetes [100 140 180], type 1 [171 221 261], type 2 [249 316
400].
Defuzzification Process
Defuzzification Process
Prediabetes or diabetes
Prediabetes or diabetes
Fig5: Flow chart of Patient Detection system.
Lofti A Zadeh introduced the theory of fuzzy logic in the late 1960s[3]. Fuzzy logic look like the human decision making approach[3]. Second step is fuzzy set theory author named as patient detection system.
In the input data processing, 6 input parameters and single output parameters. Input data processing with its membership functions are as follows:
-
Body Mass index
The range of body mass index membership functions are normal weight is [18.05 20.7 22.6
24.9], over weight is [24.2 26.1 28 29.9], obesity
weight is [29 32.6 36.4 40].
-
Random Blood Sugar
The ranges of random blood sugar (RBS) membership functions are normal [50 84.32 107
140], medium [130 159 178 200], high [190 228
260 300].
-
Fasting Blood Sugar
The ranges of fasting blood sugar (FBS) membership functions are normal [50 69.87 82.6
100], medium [94.2 108 116 128.2], high [123
178 237 300].
-
Post Prandial Blood Sugar
Fig7: Input data processing for output parameetrs.
-
-
-
Fuzzy Rules
The membership functions used trapezoid membership functions for medical use, and it gives better result in medical field. The mamdani is used as a fuzzy set theory system. And operation is used for fuzzy rules.
These patient detections system have 108 fuzzy rules are as follows:
-
If BMI is Normal and RBS is normal and FBS is normal and OGTT is fasting and HbA1c is normal then output is prediabeets.
-
If BMI is normal and RBS is medium and PPBS is normal and OGTT is fasting and HbA1c is medium then prediabetes.
-
If BMI is normal and RBS is medium and FBS is medium and PPBS is medium and OGTT is fasting and HbA1c is medium then output is prediabetes.
-
If BMI is normal and FBS is medium and PPBS is none and OGTT si fasting then output is prediabeets.
-
If BMI is normal and RBS is medium and FBS is high and PPBS is high and OGTT is fasting and HbA1c is medium then output is prediabetes.
-
If BMI is normal and RBS is medium and FBS is high and PPBS is high and OGTT is 1hour and HbA1c is medium then output is prediabetes.
-
If BMI is normal and RBS is medium and FBS is high and PPBS si high and OGTT is 2hour and HbA1c is medium then output prediabetes.
-
If BMI is normal and RBS is high and FBS is medium and PPBS is high and OGTT is fasting and HbA1c is medium then output is prediabetes.
-
If BMI is normal and RBS is high and FBS is medium and PPBS is high and OGTT is 2hour and HbA1c is medium then output is prediabetes.
-
If BMI is normal and RBS is high and FBS is medium and PPBS is high and OGTT is 1hour and HbA1c is medium then output is prediabetes.
-
If BMI is normal and RBS is high and FBS is medium and PPBS is high and OGTT is fasting and HbA1c is high then output is prediabetes.
-
If BMI is normal and RBS is high and FBS is high and OGTT is fasting and HbA1c is high then output is prediabetes.
-
If BMI is normal and RBS is high and FBS is high and OGTT is 2hour and HbA1c is high then output is prediabetes.
-
If BMI is normal and RBS is high and FBS is high and OGTT is 1hour and HbA1c is high then output is prediabetes.
-
If BMI is over and RBS is normal and FBS is normal and PPBS is normal and OGTT is fasting and HBA1c is normal then output is prediabetes.
-
If BMI is over and RBS is normal and FBS is normal and PPBS is normal and OGTT is fasting and HbA1c is medium then output is type 1.
-
If BMI is over and RBS is normal and FBS is normal and PPBS is normal and OGTT is fasting and HBA1c is high then output is type 1.
-
If BMI is over and RBS is normal and FBS is normal and PPBS is medium and OGTT is fasting and HbA1c is medium then output is type 1.
-
If BMI is over and RBS is normal and FBS is medium and PPBS is medium and OGTT is fasting and HbA1c is medium then output is type 1.
-
If BMI is over and RBS is medium and FBS is medium and PPBS is medium and OGTT is fasting and HbA1c is medium then output is type 1.
-
If BMI is over and RBS is medium and FBS is medium and PPBS is medium and OGTT is fasting and HbA1c is medium then output is type 1.
-
If BMI is over and RBS is medium and FBS is medium and PPBS is medium and OGTT is 2hour and HbA1c is medium then output is type 1.
-
If BMI is over and RBS is medium and FBS is medium and PPBS is medium and OGTT is 1hour and HbA1c is medium then output is type 1.
-
If BMI is over and RBS is medium and FBS is medium and PPBS is medium and OGTT is fasting and HbA1c is high then output is type 1.
-
If BMI is over and RBS is medium and FBS is medium and PPBS is medium and OGTT is 1hour and HbA1c is high then output is type 1.
-
If BMI is over and RBS is medium and FBS is medium and PPBS is medium and OGTT is 2hour and HbA1c is high then output is type 1.
-
If BMI is obesity and RBS is high and FBS is high and PPBS is medium and OGTT is fasting and HbA1c is medium then output is type 2.
-
If BMI is obesity and RBS is high and FBS is high and PPBS is medium and OGTT is 2hour and HbA1c is medium then output is type 2.
-
If BMI is obesity and RBS is high and FBS is high and PPBS is medium and OGTT is 1hour and HbA1c is medium then output is type 2.
-
If BMI is obesity and RBS is high and FSB is high and PPBS is high and OGTT is fasting and HbA1c is medium then output is type 2.
-
If BMI is obesity and RBS is high and FSB is high and PPBS is high and OGTT is 2hour and HbA1c is medium then output is type 2.
-
If BMI is obesity and RBS is high and FSB is high and PPBS is high and OGTT is 1hour and HbA1c is medium then output is type 2.
-
If BMI is obesity and RBS is high and FBS is high and PPBS is high and OGTT is fasting and HbA1c is high then output is type 2.
-
If BMI is obesity and RBS is high and FBS is high and PPBS is high and OGTT is 1hour and HbA1c is high then output is type 2.
-
If BMI is obesity and RBS is high and FBS is high and PPBS is high and OGTT is 2hour and HbA1c is high then output is type 2.
And many more fuzzy rules.
-
-
Defuzzification process
Defuzzification is the process in which fuzzy sets converts into crisp sets for understanding a human language[1]. This patient detection system used centroid in defuzzification process and converts in crisp sets for the output.
-
-
RESULT
This result gives the output of prediabetes or type 1 diabetes or type 2 diabetes present or not in a person is diagnosed. This patient detection system is a diagnostic technique for medical practitioner and doctor to diagnosed diabetes diseases in patients. In result it shows the prediabeets and diabetes condition in the patients from the laboratory tests.
Fig8: RBS and BMI output.
-
CONCLUSION
-
In this current era, artificial intelligence is used in everywhere and also in medical field for diagnostic techniques, monitoring and telemetry also. Fuzzy set theory is used for diagnostic technique of diabetes and it is easy to operate and accurate result comes from any manual techniques.
ACKNOWLEDGMENT
I am thankful to Dr. Smita Swamminarayan who helps me in providing information, complications for diabetes mellitus. I am also thankful to my guide Prof. Raksha K Patel and Prof. Tejas V Bhatt for giving me such a great guidance and their knowledge to me.
REFERENCES
-
H. Chitara, R. K. Patel, and T. V Bhatt, Complications of Diabetes with Cardiovascular Diseases Diagnostic Using Fuzzy Set Theory, Int. Res. J. Eng. Technol., pp. 30203026, 2020, [Online]. Available: www.irjet.net.
-
H. W. Baynest, Classification, Pathophysiology, Diagnosis and Management of Diabetes Mellitus, J. Diabetes Metab., vol. 06, no. 05, 2015, doi: 10.4172/2155-6156.1000541.
-
H. Chitara, R. K. Patel, and T. V Bhatt, Diabetic Retinopathy Diagnosing using Fuzzy Image Processing, no. 6, pp. 42104215, 2020, doi: 10.35940/ijrte.F9240.038620.
-
World Health Organization, TADDS: Tool for assessment of diabetes and diabetic retinopathy, 2015.
-
Original Research Paper Engineering AI BASED EXPERT SYSTEM FOR PREDICTION OF DIABETIC EYE Himal Chitara Dr . Hitesh Gilder * Tejas V Bhatt Raksha K Patel, no. 3, pp. 1013, 2020.
-
Z. Niswati, F. A. Mustika, and A. Paramita, Fuzzy logic implementation for diagnosis of Diabetes Mellitus disease at Puskesmas in East Jakarta, J. Phys. Conf. Ser., vol. 1114, no. 1, 2018, doi: 10.1088/1742-6596/1114/1/012107.
-
Central Council For research in Ayurvedic Sciences, Guidelines for prevention and management of Diabetes, no. 61, pp. 116.
-
N. Chandgude and S. Pawar, Diagnosis of diabetes using fuzzy inference system, Proc. – 2nd Int. Conf. Comput. Commun. Control Autom. ICCUBEA 2016, 2017, doi: 10.1109/ICCUBEA.2016.7860001.
-
S. Kumar and G. Kaur, Detection of Heart Diseases using Fuzzy Logic, Int. J. Eng. Trends Technol., vol. 4, no. 6, pp. 26942699, 2013.
-
E. Diderholm et al., ST depression in ECG at entry indicates severe coronary lesions and large benefits of an early invasive treatment strategy in unstable coronary artery disease; the FRISC II ECG
substudy, Eur. Heart J., vol. 23, no. 1, pp. 4149, 2002, doi: 10.1053/euhj.2001.2694.
-
I. Contreras and J. Vehi, Artificial intelligence for diabetes management and decision support: Literature review, J. Med. Internet Res., vol. 20, no. 5, 2018, doi: 10.2196/10775.
-
L. Wu, Classification of diabetic retinopathy and diabetic macular edema, World J. Diabetes, vol. 4, no. 6, p. 290, 2013, doi: 10.4239/wjd.v4.i6.290.
-
S. Sangam, A. Naveed, M. Athar, P. Prathyusha, S. Moulika, and S. Lakshmi, International Journal of Health Sciences and Research, vol. 5, no. 1, pp. 156164, 2015.
-
R. L. Thomas, S. Halim, S. Gurudas, S. Sivaprasad, and D. R. Owens, IDF Diabetes Atlas: A review of studies utilising retinal photography on the global prevalence of diabetes related retinopathy between 2015 and 2018, Diabetes Res. Clin. Pract., vol. 157, p. 107840, 2019, doi: 10.1016/j.diabres.2019.107840.
-
A. Gordon, Z. Buch, V. Baute, and R. Coeytaux, Use of Ayurveda in the Treatment of Type 2 Diabetes Mellitus, Glob. Adv. Heal. Med., vol. 8, p. 216495611986109, 2019, doi: 10.1177/2164956119861094.
-
I. No and M. Kalpana, Available Online at www.ijarcs.info Diagnosis of Diabetes using Correlation fuzzy logic in Fuzzy Expert System, vol. 3, no. 1, 2012.
-
L. B. O. Abdulfatai B. Olokoba, Olusegun A. Obateru, Type 2 Diabetes: a Review of Current Trends -, Int. J. Curr. Res. Rev., vol. 7, no. 18, pp. 6166, 2015.
-
A. Rungta and T. Nadu, A Review on Classification of Diabetes using Fuzzy Logic and Optimization Technique, vol. 13, no. 8, pp. 2143 2150, 2017.
-
W.: Www, R. P. Ambilwade, R. R. Manza, and R. Kaur, International Journal of Emerging Technology and Advanced Engineering Prediction of Diabetes Mellitus and its Complications using Fuzzy Inference System, Certif. J., vol. 9001, no. 7, 2008, [Online]. Available: www.ijetae.com.
-
M. Rahmani Katigari, H. Ayatollahi, M. Malek, and M. Kamkar Haghighi, Fuzzy expert system for diagnosing diabetic neuropathy, World J. Diabetes, vol. 8, no. 2, p. 80, 2017, doi: 10.4239/wjd.v8.i2.80.
-
H. Chitara, P. R. Patel, and P. T. Bhatt, Diabetes Diseases Diagnosed Support System Using Artificial Intelligence, pp. 17, 2020.
-
R. B. Lukmanto, Suharjito, A. Nugroho, and H. Akbar, Early detection of diabetes mellitus using feature selection and fuzzy support vector machine, Procedia Comput. Sci., vol. 157, pp. 4654, 2019, doi: 10.1016/j.procs.2019.08.140.
-
I. K. Mujawar and B. T. Jadhav, Web-based Fuzzy Expert System for Diabetes Diagnosis, Int. J. Comput. Sci. Eng., vol. 7, no. 2, pp. 995 1000, 2019, doi: 10.26438/ijcse/v7i2.9951000.
-
S. Swati and P. Agarwal, Diabetes Mellitus: An Ayurvedic View, J. Sci. Innov. Res., vol. 4, no. 4, pp. 193196, 2015, [Online]. Available: www.jsirjournal.com.