
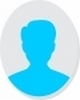
- Open Access
- Authors : M. Srikala , D. Meghan Chowdary , D. V. Sathwik Reddy
- Paper ID : IJERTV12IS040197
- Volume & Issue : Volume 12, Issue 04 (April 2023)
- Published (First Online): 26-04-2023
- ISSN (Online) : 2278-0181
- Publisher Name : IJERT
- License:
This work is licensed under a Creative Commons Attribution 4.0 International License
Predictive Cancer Therapy: Characterization using Magnetic Resonance and Machine Learning
M. Srikala (Asst.Professor) Department of Computer Science & Engineering
CMR College of Engineering & Technology, Hyderabad, Telangana
D. Meghan Chowdary
(B.Tech. Computer Science & Engineering) CMR College of Engineering & Technology, Hyderabad, Telangana
D. V. Sathwik Reddy
(B.Tech. Computer Science & Engineering) CMR College of Engineering & Technology, Hyderabad, Telangana
Abstract – Cancer predictive therapy has become an important area of research in recent years as researchers seek to develop more effective and personalized treatments for patients. A promising approach is to use magnetic resonance imaging (MRI) and machine learning algorithms to predict treatment outcomes and guide treatment selection. This paper provides an overview of the current state of research on predictive cancer therapy using MRI and machine learning, with a focus on characterizing cancer tissue and identifying biomarkers that can be used to predict treatment response . We also discuss the main challenges and limitations of this approach, including the need for large and diverse datasets, the potential for bias and overfitting, and the difficulty of interpreting complex machine learning models. Finally, we highlight some of the most promising applications of predictive cancer therapy using MRI and machine learning. This includes identifying new drug targets, developing individualized treatment plans, and optimizing treatment sequences. Overall, we believe that predictive cancer treatment using MRI and machine learning has the potential to revolutionize cancer care in the years to come, and we look forward to seeing how this field evolves.
Keywords – Predictive cancer therapy,magnetic resonance imaging,machine learning,cancer characterization,treatment response prediction.
INTRODUCTION
Cancer is one of the leading causes of death worldwide and despite significant advances in cancer treatment, cancer remains a major challenge for patients and healthcare professionals. One reason for this is the heterogeneity of cancer. It is difficult to predict which patients will respond to treatment. This has increased interest in the field of predictive cancer therapy, which aims to develop more individualized and effective treatments based on the specific characteristics of each patient's cancer.In recent years, a significant amount of research has focused on magnetic resonance imaging (MRI) and the use of machine learning algorithms for predictive cancer treatment. MRI is a noninvasive imaging technique that can provide high- resolution images of cancer tissue, and machine learning algorithms can be used to analyze these images to identify patterns and biomarkers that can be used to predict treatment outcome. By combining these techniques, researchers hope to develop more accurate and reliable methods to predict treatment response and guide treatment selection.This article provides an overview of the current state of research on
predictive cancer treatment using MRI and machine learning. We first describe the basic principles of MRI and machine learning and the major challenges and limitations of using these techniques for predictive cancer therapy. We then examine some of the most promising applications of predictive cancer therapy using MRI and machine learning. This includes identifying new drug targets, developing individual treatment plans, and optimizing treatment processes. Finally, we discuss future directions in this field and the potential impact of predictive cancer therapy on cancer treatment and patient outcomes.
LITERATURE REVIEW
Ashleen et. al. has said in his paper that machine learning techniques can be effective in detecting early-stage cancers. The study described in the article used a machine learning algorithm to analyze blood samples from patients with various types of cancers and identified specific molecular biomarkers that were associated with the presence of cancer. The authors suggested that these biomarkers could potentially be used in a blood test to screen for early-stage cancers in a non-invasive and cost-effective manner.
The authors also acknowledged that further research is needed to validate the accuracy and reliability of the machine learning algorithm and to optimize the biomarker panel for different types of cancers. Additionally, the authors noted that the use of machine learning in cancer detection raises ethical and social concerns, including issues related to data privacy, equity, and access to healthcare. Overall, the authors concluded that machine learning has the potential to improve early detection and diagnosis of cancer, but that it should be used in conjunction with existing clinical practices and regulatory frameworks.
Bendta et. al. has said in his paper that machine learning techniques have the potential to improve cancer diagnosis by identifying undiagnosable cancers. The article describes a study in which a machine learning model was trained on gene expression data from patients with known cancer diagnoses and then used to predict cancer diagnoses in patients with undiagnosable cancer-like symptoms.The results of the study showed that the machine learning model was able to correctly identify the cancer subtype in 75% of patients with undiagnosable cancer-like symptoms. The
author concludes that these results demonstrate the potential of machine learning to improve cancer diagnosis by identifying cancers that are currently difficult to diagnose.
The author also notes that machine learning techniques have the potential to improve cancer treatment by identifying new therapeutic targets and predicting patient responses to different treatments. However, the author emphasizes that further research is needed to validate these findings and to develop effective machine learning models for cancer diagnosis and treatment.
Sayed et. al. has said in his paper that machine learning has enormous potential for improving cancer prediction and patient outcomes. The author highlights that machine learning techniques can be used to analyze large and complex datasets, including genomic data, medical images, and clinical data, and to identify patterns and relationships that are difficult or impossible to discern with traditional statistical methods.
The article emphasizes the importance of interdisciplinary collaboration between data scientists, clinicians, and researchers to develop and validate machine learning models that are accurate, reliable, and clinically relevant. The author highlights several examples of successful machine learning applications in cancer detection, including the use of deep learning techniques to analyze medical images and the use of machine learning models to predict patient outcomes and identify potential therapeutic targets.
Overall, the author concludes that the integration of machine learning techniques into cancer prediction has the potential to revolutionize cancer diagnosis and treatment and to significantly improve patient outcomes. However, the author also notes that there are still several challenges to be addressed, including the need for larger and more diverse datasets, the potential for bias and overfitting, and the difficulty of interpreting complex machine learning models.
Charnpreet et. al. has says that a comprehensive survey of the recent research work related to the application of machine learning in the detection and diagnosis of COVID-19. The authors of the paper concluded that machine learning techniques have been widely applied to various aspects of COVID-19 detection and diagnosis, including medical imaging analysis, clinical data analysis, and text analysis.
The authors noted that machine learning techniques have shown promising results in detecting COVID-19 in medical images such as CT scans, X-rays, and ultrasound images. They also mentioned that machine learning models have been used to analyze clinical data such as vital signs and laboratory test results to predict COVID-19 severity and prognosis. In addition, the authors highlighted the potential of natural language processing techniques to analyze text data related to COVID-19, such as medical records and social media posts.
However, the authors also pointed out several challenges that need to be addressed in the development and deployment of machine learning models for COVID-19 detection and diagnosis. These challenges include the need for large and diverse datasets, the potential for bias and overfitting, and the difficulty of interpreting complex machine learning models.
Overall, the authors of the paper concluded that the integration of machine learning techniques into COVID-19 detection and diagnosis has the potential to improve the accuracy and efficiency of the diagnostic process, and to support clinical decision-making. However, further research and validation are needed to ensure that these techniques can be effectively deployed in clinical settings.
Kaur et. al. has said in his paper that machine learning techniques have the potential to improve the accuracy of bone cancer detection, and that different techniques, including feature extraction and classification algorithms, can be used to analyze bone scans for the detection of bone cancer.
The authors also highlight the challenges associated with the accurate detection of bone cancer, such as the need for large and diverse datasets, the selection of appropriate features, and the optimization of machine learning algorithms for bone cancer detection. The paper concludes that the use of machine learning techniques in bone cancer detection is a promising area of research that requires further exploration and development.
It is important to note that the conclusion of a research paper is the final section where the authors summarize their findings, highlight the limitations of their study, and suggest future directions for research. Therefore, the conclusion may vary depending on the research question, methodology, and findings of the study.
CHALLENGES IN SEGREGATION OF CANCER PATIENTS
One of the main challenges in segregating cancer patients is the heterogeneity of cancer. Cancer can vary widely in terms of its molecular and genetic profiles, as well as its clinical behavior. This can make it difficult to accurately classify patients based on tumor characteristics. For example, two patients with seemingly similar tumors may respond differently to the same treatment, making it difficult to predict treatment outcomes or select the most appropriate therapy.
In addition, there is a limited availability of data on cancer patients, especially for rare or less common types of cancer. This can make it difficult to develop accurate models for patient classification and treatment selection. Furthermore, there is currently a lack of standardization in the methods used for cancer classification and treatment selection. This can lead to variability in the accuracy of patient segregation and the effectiveness of treatments.
Ethical concerns also play a role in patient segregation, as there is potential for stigmatization or discrimination based on cancer type or severity. For example, patients with more advanced or aggressive cancers may be perceived as having a poorer prognosis or lower quality of life, leading to bias in treatment selection or clinical trial participation.
Resource constraints can also be a challenge in segregating cancer patients. Segregating patients can be resource- intensive, requiring specialized equipment and expertise that may not be available in all healthcare settings. This can limit access to effective treatment options for some patients, particularly those in under-resourced areas or countries.
Finally, patient preferences and values can also play a role in the segregation of cancer patients. Some patients may have personal beliefs or goals of care that influence their
treatment decisions or participation in clinical trials. For example, some patients may prioritize quality of life over survival, while others may be more willing to undergo aggressive treatments to achieve a cure. Understanding and respecting patient preferences and values is critical in achieving effective patient segregation and improving outcomes for cancer patients.
CLASSIFICATION OF MACHINE LEARNING TECHNIQUES USED IN CANCER DETECTION
Machine learning (ML) has become an increasingly popular tool in cancer detection and diagnosis, enabling physicians to analyze large datasets of patient information and identify
patterns that may indicate cancer. can. ML techniques can be loosely divided into her several main categories
In supervised learning, an ML algorithm is trained on a labeled dataset whose desired output is already known. The algorithm then uses this training data to predict outputs for new hidden data points. Supervised learning techniques are commonly used in cancer detection to classify patient samples as cancerous or non-cancerous based on certain characteristics or biomarkers. Common supervised learning algorithms used in cancer detection include support vector machines, random forests, and artificial neural networks.
Fig1: Supervised learning classification of machine learning techniques used in cancer detection
Unsupervised learning, on the other hand, trains algorithms on unlabeled datasets with the goal of identifying underlying patterns and structures in the data. This type of learning is particularly useful for cancer detection, where the complex interactions between different genes and signaling pathways are not yet fully understood. Unsupervised learning algorithms are used to group patient samples based on similarities in gene expression and other characteristics, and to identify key biomarkers and signaling pathways that may be associated with cancer development and progression. can be specified. Common unsupervised learning algorithms used for cancer detection include clustering algorithms such as k-means and hierarchical clustering, principal component analysis, and probabilistic neighborhood embedding of t distributions. In addition to supervised and unsupervised learning, other ML techniques such as reinforcement learning and deep learning are also being investigated in cancer detection. Whereas reinforcement learning trains algorithms to make decisions based on trial and error, deep learning uses artificial neural networks.
Feature selection techniques:
Using feature selection techniques, we identify the subset of traits that are most informative in predicting the presence or absence of cancer. A common feature selection technique is principal component analysis (PCA), which is used to determine the dimensionality of high-dimensional data such as: B. Gene expression data to reduce. PCA identifies the linear combinations of genes that explain the maximum
variance in the data and projects the data onto these new axes. Another feature selection technique is recursive feature elimination (RFE). It is used to iteratively remove the least informative features until the best subset is identified. RFE starts with all features, trains a machine learning model, then removes the least important features and repeats the process until it reaches the desired number of features. Feature selection techniques can improve the accuracy of machine learning models by focusing on the most informative features and reducing the dimensionality of the data. Removing irrelevant or redundant features allows the model to be trained with a smaller feature set, improving efficiency and generalization performance. Additionally, trait selection techniques can help identify important biomarkers or genes associated with cancer, leading to new insights into disease and potential therapeutic targets. However, it is important to note that feature selection is a difficult problem and the optimal subset of features may depend on the particular data set and machine learning algorithm used.
Ensemble learning techniques:
Ensemble learning techniques combine multiple machine learning models to improve overall prediction accuracy. A popular method for ensemble learning is random forest. This is a collection of decision trees trained on different subsets of data. Random Forest combines the outputs of Decision Trees to make final predictions that improve the overall accuracy of the model. Another method of ensemble learning is stacking. Stacking trains multiple machine
learning models on different subsets of data and combines their results using metamodels.
Ensemble learning techniques can improve the accuracy and generalization performance of machine learning models by reducing overfitting and combining the strengths of different models. By combining multiple models, the ensemble can capture a wider range of patterns and relationships in the data, improving predictive power. Furthermore, ensemble learning techniques can provide a measure of uncertainty in model predictions. This helps in clinical decision making. However, it is important to note that ensemble learning is computationally intensive and may require careful hyperparameter tuning.
Transfer learning method:
Transfer learning techniques involve applying knowledge learned from one task to another related task. A common method of transfer learning in cancer detection is fine- tuning. This method takes a pre-trained model on a large dataset and retrains it on a related smaller dataset. For example, a CNN model pretrained on natural images can be fine-tuned to detect cancer in medical images. Another technique of transfer learning is domain adaptation. This is adapted so that a model trained in one domain works well in another related domain.
Transfer learning techniques can improve the accuracy and generalization performance of machine learning models by leveraging knowledge learned from related tasks or domains. Starting with a pretrained model, you can initialize the model with weights that capture common patterns in your data. This speeds up the training process and improves performance on the target task or domain. In addition, transfer learning techniques are particularly useful for cancer detection where large datasets may not be available depending on cancer type and imaging modality. By leveraging pre-trained models, transfer learning can help overcome the limitations of small data sets and improve the accuracy of cancer detection models.
However, it is important to note that transfer learning techniques must carefully consider similarities between source and target tasks or domains. If the source and target tasks are too different, transfer learning is ineffective and can even impact performance. In addition, transfer learning can introduce bias if the pretrained model was trained on biased data or if the target domain has a different underlying distribution than the source domain. Therefore, it is important to carefully evaluate the performance of transfer learning models and generalize well to the target task or domain.
Deep learning technology:
Deep learning techniques involve training multilayer neural networks to automatically learn representations of input data. A popular deep learning technique for cancer detection is convolutional neural networks (CNNs), which are used to analyze medical images such as CT scans, MRI scans, and histopathological images. CNNs can automatically learn
features at multiple spatial scales and capture complex patterns and relationships in data. Another deep learning technique is recurrent neural networks (RNNs), which are used to analyze time series data such as gene expression data. RNNs can capture temporal dependencies and model the dynamic behavior of genes over time. Deep learning techniques can improve the accuracy and generalization performance of machine learning models by automatically learning representations of input data. By learning features at multiple spatial or temporal scales, deep learning models can capture more complex patterns and relationships in data and improve their predictive power. Additionally, deep learning techniques can be used for end-to-end learning. This reduces the need for manual feature engineering as the entire pipeline from raw input data to output prediction is learned automatically. However, it is important to note that training deep learning models is computationally intensive and may require large amounts of data to avoid overfitting.
Interpretability techniques:
Interpretability techniques are used to explain the predictions of machine learning models and provide insight into the mechanisms underlying cancer. A common interpretability technique is the saliency map, which is used to visualize the regions of the image that are most important for the model's predictions. Saliency maps help identify specific features and regions in cancer-related medical images and can provide insight into the underlying biological processes of disease. Another interpretability technique is decision trees, which are used to visualize the decision-making process of machine learning models. Decision trees help identify specific traits or biomarkers that are most powerful in predicting the presence or absence of cancer. Interpretability techniques can improve the transparency and trustworthiness of machine learning models by providing insight into the basis of their predictions. By describing specific features or regions of cancer-related images, interpretability technology can provide physicians with additional information to guide diagnostic and treatment decisions. Additionally, interpretability techniques can help identify potential biases and errors in machine learning models, improving reliability and generalization performance. However, it is important to note that interpretability techniques may not always lead to a complete understanding of the underlying mechanisms in cancer and may be limited by the complexity of machine learning models.
CONCLUSION
Applying machine learning techniques to cancer detection may improve early detection, diagnosis and treatment of the disease. A variety of machine learning techniques, including traditional machine learning techniques, transfer learning, deep learning techniques, and interpretability techniques, analyze medical images, genomic data, and clinical data to identify biomarkers and other characteristics associated with cancer. has been used to identify These techniques have shown promising results in accurately detecting cancer, predicting patient outcomes, and identifying potential therapeutic targets.
However, the development of effective machine learning models for cancer detection has many challenges, such as the need for large and diverse datasets, the possibility of bias and overfitting, and the difficulty of interpreting complex machine learning models. I am facing some challenges. Addressing these challenges requires clinicians, researchers, and data scientists to work together to develop and validate accurate, reliable, and transparent machine learning models. Overall, the integration of machine learning techniques into cancer detection has the potential to revolutionize cancer diagnosis and treatment and improve patient outcomes.
REFERENCES –
[1] Using machine learning to detect early-stage cancers – Berkeley Engineering. (n.d.). Berkeley Engineering. https://engineering.berkeley.edu/news/2021/08/using-machine- learning-to-detect-early-stage-cancers/ [2] | Koch Institute, B. S. (2022, September 1). Using machine learning to identify undiagnosable cancers. MIT News | Massachusetts Institute of Technology. https://news.mit.edu/2022/using-machine- learning-identify-undiagnosable-cancers-0901 [3] Sayed, S. (2018, December 10). Machine Learning Is The Future Of Cancer Prediction. Medium. https://towardsdatascience.com/machine-learning-is-the-future-of- cancer-prediction-e4d28e7e6dfa [4] Sruthi, G., Ram, C. L., Sai, M. K., Singh, B. P., Majhotra, N., & Sharma, N. (n.d.). Cancer Prediction using Machine Learning | Semantic Scholar. Cancer Prediction Using Machine Learning | Semantic Scholar. https://www.semanticscholar.org/paper/Cancer- Prediction-using-Machine-Learning-Sruthi-Ram/8cabf0293212214bc97fbbcc018f06cd06ecdb64
[5] Mallick, S., & Mishra, S. P. (2022, March 22). Machine Learning Algorithm for Detecting Lung Cancer: A Review. Machine Learning Algorithm for Detecting Lung Cancer: A Review | SpringerLink. https://doi.org/10.1007/978-981-16-8826-3_12 [6] Kaur, E. C., & Garg, U. (2020, January 1). Bone Cancer Detection techniques using Machine Learning | Semantic Scholar. Bone Cancer Detection Techniques Using Machine Learning | Semantic Scholar. https://www.semanticscholar.org/paper/Bone-Cancer- Detection-techniques-using-Machine-Kaur-Garg/a73af9ef048709bce4c93d79a12c1ac39213e8e
[7] Sharma, A., & Rani, R. (2021, January 25). A Systematic Review of Applications of Machine Learning in Cancer Prediction and Diagnosis – Archives of Computational Methods in Engineering.SpringerLink. https://doi.org/10.1007/s11831-021-09556-z
[8] Ippolito, P. P. (2020, September 17). Understanding Cancer using Machine Learning. Medium. https://towardsdatascience.com/understanding-cancer-using- machine-learning-84087258ee18 [9] goel, V. (2018, October 12). Building a Simple Machine Learning Model on Breast Cancer Data. Medium. https://towardsdatascience.com/building-a-simple-machine- learning-model-on-breast-cancer-data-eca4b3b99fa3 [10] Er. Charnpreet Kaur, Urvashi Garg,Bone Cancer Detection techniques using Machine Learning. (n.d.). Bone Cancer Detection Techniques Using Machine Learning | IEEE Conference Publication| IEEE Xplore. https://ieeexplore.ieee.org/document/10097354
[11] Tran, K. A., Kondrashova, O., Bradley, A., Williams, E. D., Pearson,J. V., & Waddell, N. (2021, September 27). Deep learning in cancer diagnosis, prognosis and treatment selection – Genome Medicine.
BioMed Central. https://doi.org/10.1186/s13073-021-00968-x
[12] H., Sharma, A., Yadav, D. P., Garg, H., Kumar, M., Sharma, B., & Koundal, D. (2021, December 20). Bone Cancer Detection Using Feature Extraction Based Machine Learning Model. Bone Cancer Detection Using Feature Extraction Based Machine Learning Model. https://doi.org/10.1155/2021/7433186 [13] Skin cancer detection and classification using machine learning. (2020, August 15). Skin Cancer Detection and Classification Using Machine Learning – ScienceDirect. https://doi.org/10.1016/j.matpr.2020.07.366 [14] K.manohar,Cancer Detection using Deep Learning. (n.d.). Cancer Detection Using Deep Learning. https://www.linkedin.com/pulse/cancer-detection-using-deep- learning-dr-manoharan-k [15] H., Ghaderzadeh, M., Asadi, F., Hosseini, A., Bashash, D., Abolghasemi, H., & Roshanpour, A. (2021, June 25). Machine Learning in Detection and Classification of Leukemia Using Smear Blood Images: A Systematic Review. Machine Learning in Detection and Classification of Leukemia Using Smear Blood Images: A Systematic Review. https://doi.org/10.1155/2021/9933481