
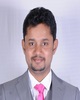
- Open Access
- Authors : Manoj H M , Mohammed Zaid Siddiqui , Saurav Kumar Yadav , Sanjeev Kumar Suman
- Paper ID : IJERTV12IS040271
- Volume & Issue : Volume 12, Issue 04 (April 2023)
- Published (First Online): 03-05-2023
- ISSN (Online) : 2278-0181
- Publisher Name : IJERT
- License:
This work is licensed under a Creative Commons Attribution 4.0 International License
Revelation of Fraud and Malicious Account Online
Manoj H M
Assistant Professor,
Dept. of CSE, BMS Institute of Technology and Management, Bangalore, India
Mohammed Zaid Siddiqui
Student,
Dept. of CSE, BMS Institute of Technology and Management, Bangalore India.
Saurav Kumar Yadav
Student,
Dept. of CSE, BMS Institute of Technology and Management, Bangalore, India.
Sanjeev Kumar Suman
Student,
Dept. of CSE, BMS Institute of Technology and Management, Bangalore, India.
Abstract:- People's social life have gotten increasingly enmeshed with online social networks (OSNs) in recent years. They utilize OSNs to communicate with one another, exchange information, arrange events, and even conduct their own e- commerce. Because of the rapid rise of OSNs and the vast quantity of personal data gathered from its users, attackers and imposters have sought to steal personal data, spread fake news, and engage in illicit activities. However, researchers have strongly disagreed. Do you read? This exchange? It looks to be adequate. Continue reading to discover how to detect aberrant behavior and fraudulent accounts using account characteristics and classification algorithms. However, some of the exploited account attributes have a negative influence or have no impact, suggesting that employing alone classification methods may not always yield suitable results. In order to efficiently identify fake Instagram accounts, four feature selection and dimension reduction algorithms were used in this work. To determine whether the target accounts were real or fake, three machine learning classification algorithms were used; these algorithms supported Decision Tree (DT), Logistic regression (LR), and Random forest (RF) and correctly classified approximately 89% of the accounts in our training dataset, with DT providing 91% accuracy.
Keywords: Fake accounts, Detection, Social media.
INTRODUCTION:
Popular online social networks (OSNs) include Facebook, Twitter, LinkedIn, and Google+. People use OSNs to engage with one another, share information, arrange events, and even start their own e-commerce business. Nonprofits spent approximately 2.53 million US dollars on Facebook political advertising between 2014 and 2018. OSNs are vulnerable to Sybil attacks due to their open nature and enormous quantities of personal data for their users. Facebook admitted platform abuse in 2012, including the spread of fake news, hate speech, sensational and provocative material, and so on. Academics, on the other hand, have expressed a keen interest in online Social Networks (OSNs) for mining and analyzing huge amounts of data, studying and evaluating user behavior, and uncovering deviant conduct. By finding the most effective cognitive traits that predict their consumers' attitudes, researchers were able to anticipate, analyze, and explain customer loyalty to a social media-based online brand community. With over 2.2 billion
monthly active users and 1.4 billion daily active users, Facebook's user base is growing 11% year on year. In the second quarter of 2018, Facebook reported total revenue of
$13.2 billion, with $13.0 billion coming entirely from advertising. Twitter claimed about one billion Twitter members in the second quarter of 2018, with 335 million monthly active users. Twitter reported a 2.44 billion US dollar gain in sales in 2017, but a profit loss of 108 million US dollars from the previous year. In 2015, Facebook projected that over 14 million of its monthly active members were truly unwanted as a result of harmful false identities formed in violation of the website's terms of service. Facebook released a report in the first quarter of 2018 documenting their internal processes for enforcing community standards from October 2017 to March 2018. The amount of unacceptable content deleted by Facebook is divided into six categories, according to this study: graphic violence, adult nudity and sexual activity, terrorist propaganda, hate speech, spam, and fake accounts. 837 million spam postings were eliminated, and about 583 million bogus accounts were deactivated. Facebook has eliminated over 81 million things that were infringing. Despite the eradication of millions of bogus Facebook identities, over 88 million accounts are still thought to exist. False accounts cause marketers, developers, and creative minds to doubt their reported user data, reducing profitability. Banks and financial organizations in the United States have recently began to analyze loan applicants' Twitter and Facebook sites before giving a loan. Attackers see OSN user accounts as "keys to walled gardens." As a result, they imitate others by using photographs and accounts that are either taken without the knowledge of actual individuals or created artificially in order to spread bogus news and steal personal information. These phony accounts are also known as imposters. False identities endanger customers and serve no useful purpose, since they regularly flood spam messages or steal sensitive data. They are prepared to entice unwary people into deceptive relationships that lead to sex fraud, human trafficking, and even political astroturfing. According to polls, 40% of parents in the United States and 18% of teens are concerned about the use of bogus accounts and bots to sell or influence
products on social media. Another example is the unexpected spike in followers on Romney's Twitter account during the 2012 US presidential election campaign. The great majority of them were eventually proved to be forgeries. These rogue accounts are typically equipped with hidden automatic tweeting programs known as bots, which imitate real individuals. Adrian Chen, a New Yorker journalist, saw that many of the Russian accounts he was examining had shifted to pro-Trump behavior in December 2015. Many of those identities, on the other hand, were actual people's troll accounts designed to imitate American social media profiles. Similarly, in the run-up to the Italian general elections in February 2013, online blogs and media outlets published data on a predefined proportion of major politicians' false followers. Detecting fake accounts in OSNs has become crucial in order to avoid a variety of damaging behaviors, ensure the security of user accounts, and safeguard personal information. Researchers are working to create automated detection approaches for identifying fake accounts, which would be time-consuming and expensive to perform manually. The study's findings may enable an OSN operator to detect phony accounts quickly and efficiently, improving the user experience by deleting annoying spam messages and other undesirable information. By allowing third parties to study its user accounts, the OSN operator may improve the dependability of its user metrics. For social network users, the most important criteria are information security and privacy; meeting and exceeding these requirements improves network reputation and, as a result, income. OSNs are using different detection algorithms and mitigation measures to combat the growing problem of fake/malicious accounts. Researchers focus on identifying fake accounts by evaluating user activity and acquiring data from recent users, such as the number of posts, followers, and profiles. They utilize a trained machine learning method to distinguish between authentic and bogus accounts. Another approach is to employ graph level structure, which depicts the OSN as a graph with nodes and edges. Each node represents an item (such as a bank account), and each edge represents a connection (such as friendship). Despite the fact that Sybil accounts conceal their behavior with patterns similar to legitimate accounts, they exhibit a wide range of profile features and activity patterns. As a result, automated Sybil detection is not always immune to adversarial attacks and may not always achieve ideal accuracy. In this paper, a hybrid classification method was employed by executing the Neural Network (NN) classification algorithm on the Decision tree decision values. While properly identifying around 98% of the accounts in our training sample, our approach uses fewer characteristics. Furthermore, as shown in, we tested our classifiers' detection abilities on two additional sets of real and fraudulent accounts that were segregated from the original training dataset. The rest of this work is organized as follows. Provides an overview of the Twitter network analysis as well as previous studies on bogus profile identification. Shows how the collected data was pre-processed and used to classify the accounts as fake or legitimate. The total accuracy rates were determined and compared to all other strategies used in this inquiry. This section outlines our findings.
1. RELATED WORKS:
1. S. Fong, Y. Zhuang, and J. He, Not every friend on a social network can be trusted: Classifying imposters using decision trees, : According to recent media estimates, 8.7 percent of Facebook users are impersonators, totaling more than 83 million accounts globally. As a result of a large number of unverified fake accounts, users and their families face hazards ranging from espionage, theft of identities, information abuse, and loopholes to breaches of privacy. With the growth of online social networks (OSN), it is increasingly simple to discover a potential victim using publicly available information on the Internet. Anyone can just pretend to be someone else they claim to be before verifying to see if the information is genuine. For example, it is relatively simple to forge one's identity on OSN by supplying false photographs and names, which Facebook would ignore. This paper describes an early experiment that used decision tree classification algorithms to identify imposters within a pool of "friends" on Facebook. The classification approach is similar to that used to separate spam from legitimate emails, except that instead of text-mining the message contents, the attributes of a user's account are considered. The classification addresses were determined to be 92.1% correct.
-
Y. Boshmaf, I. Muslukhov, K. Beznosov, and M. Ripeanu, The socialbot network: when bots socialize for fame and money,: Social Networks (OSNs) have grown to be an important part of today's Internet. OSNs are used by politicians, artists, revolutionaries, and others to reach millions of active internet users. Unfortunately, in the hands of the wrong people, OSNs may be used to undertake misinformation and propaganda campaigns. Typically, such campaigns begin with a large-scale entry of a certain OSN. In this paper, we look at how OSNs may be hacked on a big scale by socialbots, which are computer programs that administer OSN accounts and mimic genuine people. We built a Socialbot Network (SbN) using a common web-based botnet design: a network of adaptive social bots that are managed by a command-and-control protocol. For around 8 weeks, we ran a comparable SbN on Facebooka 750 million-user OSN. As a consequence of a broad-scale infiltration in which social bots were employed to interact with a significant number of Facebook users, we gathered user activity data. Our findings show that (1) OSNs, such as Facebook, can be infiltrated with up to 80% success, (2) a successful infiltration can result in privacy breaches that expose even more users' data than purely public access, and (3) OSN security defenses, such as the Facebook Immune System, are ineffective in detecting or stopping a large-scale infiltration as it occurs.
-
J. Ratkiewicz, M. Conover, M. Meiss, B. Gonc¸alves,
S. Patil, A. Flammini, and F. Menczer, Truthy: mapping the spread of astroturf in microblog streams: Online social media are supplementing, and
in some cases replacing, face-to-face social interaction and redefining information dissemination. Microblogs, in particular, have become critical battlegrounds for public relations, marketing, and political confrontations. We provide a web application that follows political memes on Twitter and aids in the detection of astroturfing, smear campaigns, and other disinformation in the context of US political elections. We also show some examples of abusive behavior discovered by our service. Our web application is built on an expandable architecture that will allow for real- time meme dissemination analysis in social media by mining, visualizing, mapping, categorizing, and modeling enormous streams of public microblogging events.
-
K. Thomas, C. Grier, D. Song, and V. Paxson, Suspended accounts in retrospect: an analysis of twitter spam: In this study, we investigate spammers' use of online social networks via the lens of the tools, methods, and support infrastructure on which they rely. Over the span of seven months, we discovered nearly
1.1 million Twitter accounts that were deleted for disruptive activity. During the process, we get a dataset of 1.8 billion tweets, 80 million of which belong to spam accounts. We use our data to identify the behavior and duration of spam accounts, the campaigns they run, and the widespread use of legal internet services such as URL shorteners and low-cost site hosting. We also identify a new marketplace of unlawful programs run by spammers, such as Twitter account dealers, ad-based URL shorteners, and spam affiliate schemes, which help to diversify the underground market. According to our data, 77% of Twitter spam accounts are deactivated within a day of their debut tweet. Because of these limits, less than 9% of accounts form social ties with frequent Twitter users. Instead, 17% of accounts employ trend hijacking to get followers, while 52% use nasty remarks. Despite daily account attrition, we show how five spam operations comprising 145 thousand accounts may endure for months at a time, with each campaign adopting a unique spamming method. Surprisingly, three of these advertising provide spam while leading visitors to trustworthy businesses, blurring the line between what is and is not spam on social media.
-
R. Kaur and S. Singh, A survey of data mining and social network analysis based anomaly detection techniques,: With the increasing popularity of online social networks across a variety of industries, social network analysis has recently been a focus of research. Researchers are interested in Online Social Networks (OSNs) because they may be used to analyze use and detect suspicious activities. Anomalous actions in social networks involve uncommon and unlawful behaviors that differ from others in the same framework. This study examines many forms of anomalies as well as their innovative classification based on several aspects. This Spaper provides a discussion of a number of
approaches for preventing and detecting anomalies, as well as the underlying assumptions and causes for the appearance of such anomalies. The paper provides an overview of many data mining methodologies for detecting abnormalities. Priority is given to the research of social network-centric anomaly detection algorithms, which are broadly characterized as behavior-based, structure-based, and spectral-based. Each of these classes has a number of techniques that are discussed in the research. The study concludes with many future paths and research topics that might be addressed and worked on.
-
METHODOLOGY
PROPOSED SYSTEM:
We recommend this application as a helpful solution since it assists in lowering the limits derived from traditional and other existing ways. The purpose of this study is to develop a rapid and dependable method for detecting emotion. To build this system, we used acomplex algorithm in a Python- based environment.
Fig. block diagram of the proposed system
-
IMPLEMENTATION:
DECISION TREE:
A decision tree is a machine learning classification technique which is also capable of performing regression process. It can be employed to the wide range of applications in the day to day as it can perform classification as well as regression. It is normally employed in making decisions and also for analyzing those decisions. It can reflect the decision made in a graphical manner. Similar to the name referring, it applies a tree like method for making accurate decisions by using various if and else conditions. A typical decision tree is represented in fig. 1
Fig. 1 Representation of a typical decision tree
Normally, the root of a decision is mostly present in the top position as seen in the above figure. Based on the condition node, it separates into various branches which normally depicts the decisions made. This is termed as the decision and this further divides into various different decisions and this serves as the final decision that is made. The finally obtained branches of the tree present at the bottom are referred to as the leaf node. Since various branches are being divided, a sub tree is formed as a result which is also depicted in the above figure.
What is truly occurring in the background, then? Making decisions on the characteristics to employ, the circumstances to employ for dividing, and when to stop are all part of developing a decision tree. You will need to cut it down to make it seem lovely because trees often expand at random.
LOGISTIC REGRESSION
An established technique for calculating adjusted odds ratios is logistic regression. ML (i.e., Maximum Likelihood) software is usually always used to fit logistic models [24]. If the model is roughly accurate and the sample size is sufficient (e.g., a minimum of 4-5 variables are present in single parameter available in every single level of the result) [25-27], it offers meaningful statistical judgments. However, ML estimation can fail in the presence of small or sparse data sets, an unusual exposure or outcome, or significant underlying effects, particularly when these issues coexist [28-30]. Because of this, ML estimates of finite odds ratios may be infinite and ML estimators are not even roughly unbiased. The covariates are responsible for separating the outcomes and this might result in the emerging of infinite estimates [31, 32].
The relation among many independent variables that are either continuous or categorical and a dichotomous dependent variable is modelled using binary logistic regression [33]. Binary logistic regression has various guesses that must be addressed in order to get a reliable outcome [34].
-
Linearity: The connection between the explanatory factors and the response variables logit should be linear.
-
Independent mistakes: Correlation between the mistakes is inappropriate.
-
Explanatory variables shouldnt have a lot of correlation with one another to avoid multicollinearity.
-
There shouldnt be any anomalies, values with significant leverage, or points with a lot of influence.
Explanatory variables shouldnt have a strong correlation with one another, according to one of the premises of logistic regression. For the findings to be considered legitimate, the assumptions of the logistic regression model must be fulfilled. Invalid statistical conclusions may result from issues with the model, like excessively inflated standard errors, inaccurately low or high t-statistics, and parameter estimates with nonsensical signs [35]. While it may be feasible to construct experiments where the explanatory factors are orthogonal to one another, observational data does not allow for this. Then, according to another study paper [36] , collinearity is a basic rule in the data set originating from the uncontrolled processes of the data spawning system and is simply a harsh and inevitable life event in no experimental sciences. Variables with strong correlations are frequently gathered for examination in reviews. [37] In order to stabilize the estimates in situations of multicollinearity, Firths penalized likelihood equation was combined with the idea of a double penalized maximum likelihood estimator. [38] A novel technique for calculating the shrinkage parameters of the Liu-type logistic estimator was put forward. Additionally, [39] a ridge type estimator was presented, which under some circumstances has lower total mean squared error than the maximum likelihood estimator.
RANDOM FOREST:
Numerous of decision trees are grown and merged onto produce a "forest" in the RF supervised ML technique. Issues related to regression along with the classification may be solved utilizing it in Python and R programming languages. Identical to what its name reveals, a RF is an ensemble of numerous of unique kinds of decision trees. The class with the most votes become the class forecasted by our prototype that is created by the individual trees in the RF as shown in the below fig. 2. In the below figure, six predictions are made as 1 and remaining three predictions are made as 0.
Fig. 2 Representation for making a prediction using visualization of a random forest model
Specifications of Random Forests
The important specifications of the random forests are listed below [10]:
-
It is the most accurate algorithm currently in use.
-
On huge data bases, it operates effectively.
-
Without deleting any variables, it can manage a huge number of input variables.
-
Estimates of the variables' significance for categorization are provided.
-
As the forest grows, it produces a neutral internal estimate of the generalisation error.
-
It has a mechanism for accurately guessing missing data and keeps its accuracy even when a significant amount of the data is missing.
-
It includes techniques for balancing inaccuracy in uneven data sets for a class population.
-
For usage on different data in the future, generated forests can be stored.
-
Prototypes are created in order to understand more about the link between categorization and factors.
-
It calculates the distances between instances, which may be used for grouping, finding outliers, or constructing aesthetically appealing data representations (by scaling).
-
By extending the aforementioned capabilities to unlabelled data, unsupervised clustering, data views, and outlier identification are possible.
It provides a testable technique for identifying interactions of the variables.
-
-
-
RESULTS AND DISCUSSIONS
Data: View all the content in the dataset.
Neural Networks: Apply Neural Network model.
Prediction: we have to pass values to predict that person commit to Fraud or not.
Output1: View output if the person commited to Fraud.
Home Page:
This is the home page of the project. Its like a brief introduction of traffic prediction.
Graph: view graph accuracy.
-
CONCLUSION
After proper analysis and comparison, it was found that Decision tree, Logistic regression and Random forest reported the maximum accuracy .The accuracies for prediction could be further improved
-
Size of dataset changes in future (presently a constraint).
-
Class Distribution of the target variable gets balanced.
-
-
REFERENCES
-