
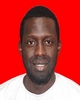
- Open Access
- Authors : Ebrima Jaw , Wang Xue Ming , Lang Loum , Lamin L. Janneh
- Paper ID : IJERTV10IS010197
- Volume & Issue : Volume 10, Issue 01 (January 2021)
- Published (First Online): 29-01-2021
- ISSN (Online) : 2278-0181
- Publisher Name : IJERT
- License:
This work is licensed under a Creative Commons Attribution 4.0 International License
Review on the Application of Medical Artificial Intelligence in The Gambia’s Health Care System
Ebrima Jaw1 2 Wang Xue Ming 1 Lang Loum 2 Lamin L. Jannep 2
1 College of Computer Science and Technology, Guizhou University (GZU), China.
Guizhou, Guiyang, 550025, China
2School of Information Technology and Communications, University of the Gambia (UTG), The Gambia.
Serekunda, P.O Box 3530, The Gambia
Abstract It is evident that quality health care increases the likelihood of desired health outcomes, leading to a healthy nation, and of course, preserving the Sustainable Development Goals (SDGs) and a healthful working population. Medical Artificial Intelligence (MAI) technologies have been radically transforming the health care systems of advanced countries such as China, the US, and India. However, The Gambia continues to struggle with the provision of equity and quality health care services, and very little or no research has been done to explore the advantage of adopting innovative technological solutions, such as MAI, to mitigate these challenges. Therefore, this paper evaluates the adoption of MAI technologies within The Gambia's health care system, intending to attain the quantifiable attributes of quality health care, such as equity, easy accessibility, timeliness, affordability, safety, and effectiveness. Additionally, the paper presents and discussed the motivation, use cases, and challenges of adopting these technologies. Most importantly, it also recommends the adoption of specific feasible MAI technologies for adoption. Finally, the findings presented in this paper will not only help to better understand the current challenges faced by Gambia's health care system but, most importantly, the solutions to these problems.
Keywords Medical Artificial Intelligences (MAI); Clinical Decision Support Systems (CDSS); Motivation; Challenges; Use Cases; The Gambia; Health Care Systems;
-
INTRODUCTION
The Gambia is a small West African country bordering on the Atlantic Ocean and surrounded on three sides by Senegal. It comprises 10,689 square kilometers of land, is home to an estimated 2.3 million people (UN, 2019), and has a density of 176 people per square kilometer [1], 39.8% of its population resides in the rural area while the whopping remaining 60.2% live in the urban areas. Interestingly, it has only 6% of its GDP as total health expenditure, life expectancy at birth 62.3, and
59.6 for women and men, respectively. Regarding the doctor- to-population ratio, the density of physicians across the country is 0.11 per 10,000 populations. Similarly, the density for midwives and nurses is 0.87 per 10,000 populations. Additionally, The Gambia has an infant mortality rate of 75/1000 live birth, an estimated maternal mortality ratio at 75/100,000 live births.
The Gambia has been among the poorest countries in the world, coupled with the factors mentioned earlier, a high-
income inequality rate of 0.47, a predicted rapid increase in population, the rapid rise in chronic diseases such as hypertension, obesity, heart disease, and diabetes. Consequently, the need for solutions to some of these challenges faced by the country, especially in health care, should be regarded as a priority. As a result, the use of MAI technologies to salvage the disheartened health care system to enhance the lives of Gambians is of great importance.
As a result of the recent widespread application of Computers, the Internet, Big Data, Cloud Computing, Machine Learning, Neural Networks, and explosive growth in handheld and smart devices such as smartphones, watches. Artificial Intelligence (AI) has drastically revolutionized various aspects of our day-to-day lives, specifically the health care systems [2]. Since its inception in 1950 by Alan Turing, Artificial Intelligence has been flourishing in a wide range of applications as a result of its ability to simulate human learning, collection, storing, and analysis of massive data, and most importantly its ability to make real-time decisions.
Therefore, the past decades have seen advanced countries harnessed Medical Artificial Intelligence's potential, which has played a massive role in creating and maintaining electronic health records (EHRs), diagnosis, treatment protocol development, patient monitoring and care, personalized medicine, robotic surgery, and health system management [2], [3], to improve health care. Consequently, yielding many positive results in cutting down health care expenditure by the government, easy accessibility to health care, improved diagnosis in terms of speed, and most importantly, physicians' efficiency. Furthermore, the author of [4] has estimated that by 2020, the health care systems expect to benefit from $45 billion in annual cost savings, which is a considerable cost saving, especially for an underdeveloped country like The Gambia.
The subsequent parts of this paper are organized as follows. Section II discusses the Motivation of applying Medical Artificial Intelligences in The Gambia's health care system. Also, Section III discusses the related work. Section IV presents some MAI use cases, while Section V highlights the challenges. Finally, Section VI summarized the methodology before the paper concludes in Section VII.
-
MOTIVATION
The challenges mentioned above, especially in the health care system, coupled with The Gambia been among the countries with the lowest doctor-to-population density of 0.11 per 10,000 populations, the need to mitigate these challenges is of great significance. Consequently, in this section, the motivations behind the application of Medical Artificial Intelligence within The Gambia's health care are discussed.
-
The Need for Timely Clinical Decision Making:
The rapid growth of medical innovation has led to a massive amount of data produced in medicine. It is stated in [2], [5] that the quantity of data in medical literature doubles every three years. Many studies have also found that for a physician to stay current, they should spend 29 hours per workday, which is practically impossible. Therefore, exclusively trusting physicians in clinical decision making can be very detrimental to primary health care. Therefore, the vibrant ecosystem of exiting Artificial Intelligence systems is a potential tool in mitigating these challenges.
A typical example is IBM's Watson, which is out- diagnosing doctors in certain situations, provide management plans for cancer patients, and helps physicians make timely and effective decisions [2], [6]. Additionally, an AI technology widely used in China called "Xiaoman" can answer 2,000 health-related questions a day compared to 600 by a front desk attending to patients in the hospital. In closing, the adoption of such AI systems within Gambia's health care system can go a long way in enhancing the quality, easy accessibility, affordability, and greater efficiency of health care services.
-
Solution to the massive number of Diagnostic Errors and Delay in Disease Detection:
Diagnostic errors have been a massive challenge for Global Health care quality and safety, and The Gambia is not an exception. Research has proven an estimated 5.08% of outpatient diagnostic errors in the United States, which is about 12 million adults yearly. Furthermore, half of these errors were estimated to be harmful to the patients [7].
According to the World Health Organization, The Gambia's lack of competent and sufficient health care workers has caused numerous diagnostic errors such as inappropriately delay or the wrong dagnostic, which in most cases, leads to death. [1]. Therefore, the use of Medical Artificial Intelligence to mitigate these challenges is of great concern. For instance, the adoption of technology such as Baidu's Clinical Decision Support System (BCDSS) in The Gambia's health care system. The system can detect and recommend treatment for 66 different diseases, which will not only record a significant impact in saving lives but will go a long way in improving the effectiveness, quality, and outcome of the country's health care system.
Additionally, a new study covering 563 cases has shown that Isabel, a web-based clinical decision support system, has achieved 98% accuracy in diagnostics [8]. The technology is available on platforms such as network computers, iPad, iPhones, and android based smartphones, making it easy to access by physicians and even patients where necessary. Finally, diabetes is among chronic killer diseases in The Gambia. Having an AI system such as IDx-DR for early detection of diabetes could be a real game-changer in the country's health care system. The above-mentioned medical AI systems have significantly enhanced the quality and efficiency of many developed country's health care systems, which could be the same for Gambia's health care system when adopted.
-
The Need for Electronic Health Records:
The Gambia is yet to have an Electronic Health Record (EHR) of her citizens, which has posed a great challenge for health workers in diagnosis and primary health care provision. Research has shown that physicians spend 34% to 55% in creating notes and reviewing medical records in Electronic Health Records [9], which reduces their productivity and the number of patients they can attend. Therefore, the need for using MAI in The Gambia's health care system will help create an EHR and enable the automation of the country's EHR, which is of paramount importance to the government and its citizens. Moreover, such systems can facilitate the collection, storing, analysis of both structured and unstructured data, and most importantly, make timely decisions, which in the long run will improve the quality and effectiveness of the health care system.
-
The Necessity for Precision Medicine:
In this booming era of Big Data, Machine Learning, Cloud Computing, and other revolutionary technologies, coupled with the availability of many biological datasets, precision medicine adoption is becoming much more comfortable than in previous decades. Precision medicine is a novel health care approach in which personal conditions such as psychosocial attributes, environment, lifestyles, and genetic information is used to provide patients with the specific treatments and prevention of disease [2]. In [10], the authors define it as the health care system that applies several tools to provide exclusively personalized diagnosis and treatment for patients. The Gambia can attain equity in health care, and most importantly, improve the country's quality of primary health care for citizens by adopting an AI technology such as Google's DeepVariant. It uses neural networks in attaining a precise genomic analysis [4], which will enable doctors to provide patients with tailored prevention and treatment based on their makeup (genetics).
-
Adoption of Medical Robots to Mitigate the Lack of Specialist in Certain Areas:
The Gambia is among the countries with the lowest specialist doctors. As a result, patients with particular critical
medical needs are referred to other countries, or they end up losing their lives. Therefore, the need to implement Medical Robotics such as Robotic surgery and other specialized medical areas will go a long way in improving Gambian citizens' primary health care needs. Medical Robotics such as Robotic surgery has revolutionized the known traditional open surgical approach, it is no longer the future wave, but it is here to stay. Advanced countries such as India, China, the United States, and many other countries have been widely adopting such AI systems, enabling doctors, especially surgeons, to attain efficiency and precision in their operations. Similarly, the patient's recovery time is much faster compared to traditional methods.
-
-
LITERATURE REVIEW
The adoption of AI-based diagnostic systems to assist health care providers in diagnostic has received much attention in recent years. During the past decades, several AI diagnostic systems were proposed, some of which play a significant role in improving physicians' effectiveness and precision in their day-to-day job. For that reason, many research works such as [11], [12], [10], have proposed, reviewed, enhanced, and, most importantly, implemented many AI-based diagnostic systems across many health care domains. According to [11], [13], [14], visual loss and blindness are a persistent threat for diabetic patients in the US and globally. The authors also stated that annually, 24,000 people lose their vision in the United States of American. The primary reason for blindness is Diabetic Retinopathy (DR). As a result, the diagnostic accuracy and efficiency of an automated AI system were evaluated and proposed by the authors of [11] for the detection of DR and DME, commonly denoted as mtmDR.
The paper's outcome was highly beneficial as it significantly improves the accuracy and timeliness of diagnostics, consequently improving the accessibility, affordability, and efficacy of patients' primary health care needs. However, the study was limited in its application as the authors choose to focus on a small size of 900 people in 10 different study sites. Likewise, the dominant effect for sex, race, age, and ethnicity was failed to be accounted for in their logistic regression model, consequently making their findings not fit for global application. The results might have been more applicable if a more extensive study group was chosen globally across different races, sex, and age. Also, the type of diabetic should have been accounted for, which was addressed in [12].
A similar study was carried out in [12]. Using a hybrid deep learning-enhanced (IDx-DR-EU-2.1) device with an improved sample size of 1,616 with type 2 diabetic, enhanced diagnostic accuracy and efficiency for vtDR and mtmDR was achieved. Nevertheless, their reading center's inferior quality and IDx-DR leads to the exclusion of 322 patients out of the
original sample, which is a considerable limitation in their findings. This paper recommends a decentralized improved sample size and a better-quality reading center and IDx-DR to mitigate the limitation mentioned above.
Moreover, the massive adoption of AI-based diagnostic systems could, in many ways, enhance the efficiency and productivity of health workers. Thus, an AI-based diagnostic system with a unique analytical process such as Watson was investigated in [6] by David Ferrucci. In [6], [5], Ferrucci elaborated on the two approaches adopted to train Watson, such as entering interpreted situations and cases by professionals within the health care system with potential best answers or diagnosis expected to be the outcome from Watson. Using an approach of feeding it with a vast amount of medical data and analysis enables it to analyze the patterns and relations within data such as doctor's notes, other significant archives such as laboratory, and genetic data [5]. As a result of which, Watson is now out-diagnosing physicians in some situations.
Nonetheless, the authors claimed that the mere inclusion of clinical presentations and phenotypes instead of scientific and biomarker data is a potential reason for Watson's less desirable outcome [6], [15]. He concludes that to attain the best objective of Watson, both scientific and clinic data have to be encompassed, which will enable a much reliable outcome. This paper's authors agree with Ferrucci's limitation and have concerned about the substantial financial expenses needed to have a system like Watson. Furthermore, a similar study was carried out by the authors of [8], [16], [17], [18] to quantify the sensitivity and swiftness of a web-based clinical decision system referred to as "Isabel" (Isabel Healthcare Inc., USA). Using 3 to 6 key findings on the system, Isabel correctly diagnosed 48 out of the 50 cases selected for the studies, which is estimated to be 96% accurate than the 95% of human doctors.
In contrast, using the copy and paste search method, "Isabel" could only make 37 correct diagnoses out of the 50 cases. The authors also highlighted that the system could produce 30 diagnoses presented on three different web pages with ten records for each. Equally, they conclude that both data entry approaches yielded speed and efficiency as the manual method took a minute to produce a result while the copy and paste required just 5 seconds.
However, the exclusion of 11 cases out of the first 61 cases is identified as a limitation. On a similar note, the presentation of the system's results is also not user-friendly, leading to missing results. "Isabel's" searching strategies are also not exhaustive, which undermines the credibility of the system's results. For this technology to fit for global use, the authors of this paper recommend the mitigation of the challenges mentioned earlier.
-
USE CASES
Out of the estimated 7 billion people living on this planet, only half receive minimal health service. Interestingly, this heartbreaking finding is predicted to get worst due to the exponential rise in the aging population, especially in Asia, the US, and other developed countries. According to the World Health Organization (WHO), the world will need an additional 5 million doctors to provide everyone with primary health care needs. Therefore, the need for innovation in dealing with these challenges should be of great concern to governments, local governments, and international organizations, which brings us to the adoption of MAI systems to solve these challenges faced by humanity. The robust ecosystem of already existing AI systems with potentially broad applications can enable the easy accessibility and affordability of health care needs in the hands of every person on earth. Therefore, leading to a reduction in error cost, shorter time in drug discovery procedure, and effective health care services [4].
Consequently, the use cases below can provide the Gambians with affordable health care and easy accessibility. However, since AI in medicine is still in its infancy, and most applications are centered on diagnosis and treatment plans, this paper will only focus on the use cases of diagnosis and treatment plans.
-
Adoption of Clinical Decision Support Systems to Help Health Care Workers:
To mitigate the escalating challenges faced by the health care systems, countries like India, China, the US, and other developed countries have been using clinical decision support systems that are enabled by the vibrant ecosystem of AI-based technologies in helping doctors and other health workers to make timely and efficient decisions. For instance, Baidu's Clinical Decision Support Systems (CDSS), which assists doctors in more than 1,000 hospitals across China, enables efficient, accurate, and timely decision-making [19]. The embracing of such a technology will play a significant role in The Gambia's health care system, such as enabling impartiality and quality health care services. Coincidently, this system is adopted in China's Tier 1 facilities, which serve as
the initial access point for primary health care services [19]. Therefore, this paper's authors believed this technology is fit for implementation in The Gambia because the country is still struggling in providing primary health care needs. A similar technology such as "Isabel" with 96% diagnostic accuracy, ease of use, most importantly, speed in diagnosis and recommendation of treatment plans can be equally adopted.
-
Adoption of Wearable Medical Artificial Intelligence Systems:
The increase in the computing and storage power of wearable devices has enabled the rapid rise of medical AI- based wearable systems such as wearable physiological monitors with vast functionalities that have never been imagined. Biobeat is an Israel based bio-medical technology company developing advanced sensing and remote 24-hour care solutions for patients at home or anywhere. On August 26, 2019, the Drug Administration (FDA) of the US granted a 510K clearance for Biobeat's ground-breaking devices, referred to as the Biobeat wearable suite. It includes a wristwatch, a single-use patch, a smartphone app for patients, and hospital screens and workstations. It is cable of reporting 16 vital health-related reports [20].
Additionally, both the watch and the Patch have a photoplethysmography (PPG) sensor, galvanic skin response (GSR) sensor, temperature sensing, and an accelerometer [20], [21].
Moreover, the devices can measure blood pressure, oxygenation and heart rate, saturation, respiratory rate, heart rate, consciousness, cardiac output, stroke volume, body temperature, steps, sweat, and much other cloud-based healthcare functionality. The device uses either a smartphone or a dedicated gateway [20], [21], which are already existing in The Gambia. One of the most significant challenges faced by The Gambia's health care system is the availability of accurate and timely diagnosis. Therefore, this paper's authors considered this technology suitably fit for adoption in The Gambia's health care system because it will enable equity and easy accessibility in primary health care needs for Gambians. Additionally, implementing such a technology might not be as hard as other complex systems because of the already existing infrastructure.
Fig 1: Biobeat's wristwatch, a single-use patch, and Hospital Screen. Source: Source: Biobeat.
Similarly, Swasthya Slate, a portable diagnostic device with the capability of conducting 33 diagnostic tests, it was launched by the Public Health Foundation of India in March 2014. It has been radically transforming India's health care system due to its impact on improving equity, efficiency, easy accessibility, and quality health care services [22]. The device is augmented with a simple box, power source, test sensors, and an Android operating system based smart device that facilitates the sending of patients' diagnostic record to the database, a primary health care worker, or the doctor through simple SMS. Furthermore, the gadget is currently being used
by fieldworkers of eight different countries on a pilot basis. Moreover, emerging and developed countries such as Pakistan, South Africa, Malaysia, and the UK have shown interest in adopting the technology. Finally, this paper's authors believed that Slate is a suitable technology for The Gambia's health care system due to its easy accessibility and time-saving factor. Likewise, it will serve as cost savings for the health care providers and the patients because $1.25 was the cost of 10 standard diagnostic tests during a pilot phase in India, which is quite affordable for almost every Gambia family.
Fig 2: Swasthya Slate Portable Diagnostic Device, Source: Swasthya Slate
across many Chinese cities and provinces due to its efficiency
-
Adoption of Artificial Intelligence-Led Telemedicine Technology:
The aging population and a shortage of qualified medical specialists have been a persistent challenge for global health care systems. According to [23], [24], there will be a whopping 90,000 vacant health care positions by the year 2025 in very critical specialties, with surgical experts leading the shortage list. Additionally, It is estimated that in the rural area of the US, there are only 30 specialists accessible for a massive 10,000 patients [23]. Therefore, the need to embrace the convergence of Health Care, Telemedicine, and Medical Artificial Intelligence to provide a more coherent, easy accessibility, affordability, and practical approach to treatment should be regarded as an urgency, especially for The Gambia with very few available medical specialists.
For that reason, the authors of this paper recommend the adoption of technology such as "Ping An Good Doctor (PAGD)" within the Gambian's health care system. Chinas primary online healthcare services provider is driven by a "One-Minute Clinics" A.I-led Telemedicine technology. Last year has witnessed "Ping An" expand A.I-based telemedicine
and cost reduction, easy accessibility, and convenience. Moreover, the technology has a robust ecosystem supported by a virtual doctor (an AI doctor), 50,000 external doctors, and 1,000 internal doctors who helped train the A.I.-led Telemedicine (One-Minute Clinics). These are sizable cabins where patients can enter to get quality health care services through the AI doctor and human doctors' help using video conferencing [25]. Finally, it has more than 3,000 partnerships with hospitals and clinics and 1,200 pharmacies, which enable patients to purchase medicine at the same place of diagnosis. If the prescribed medication is not available at the kiosk, patients can use the rich "Ping An" online shopping application to buy the medicine. So, such technology will help to alleviate the challenges faced by The Gambia's health care system.
Similarly, SOC Telemed is also a leading company that specialized in telemedicine with 15 years of experience. They provide critical specialties such as teleNeurology, telePsychiatry, and teleICU [23], which are all specialties lacking within The Gambia. Therefore, this technology is
deemed fit for adoption within the country's health care system.
-
-
CHALLENGES
Despite the pressing needs for the adoption of Medical Artificial Intelligence in The Gambia's health care system, there are evident challenges in implementing such systems in the country, and some of these problems are discussed below;
-
Lack of Essential Infrastructure:
The last decade has witnessed the technological infrastructure of The Gambia flourishing. However, compared to the recent technological development trend, the country is still facing numerous challenges, which need to be addressed to facilitate room for adopting MAI systems. Furthermore, the current electricity and internet facilities are expensive and unreliable, which posed a severe threat to adopting such technologies in the country's health care system. Consequently, addressing these challenges should be regarded as a national priority to enable a better infrastructure to implement the proposed technologies.
-
Procedural Knowhow of Health Workers in Using Such MAI Systems:
The majority of the health workers in The Gambia have minimal knowledge of technology and its related domains, which could be a severe threat to adopting such systems in the country's health care system. As discussed in [2], [4], [26], the need to use an automatic and smart method of developing a user-friendly system is necessary for easy adoption. As a result, this paper's authors believed these challenges could be mitigated when user-friendly and smart systems are implemented.
-
The Fear of Been Replaced by the Medical Artificial Intelligence Systems:
It has been a global concern among various professional domains that Artificial Intelligence could replace them. Similarly, the implementation of MAI systems could be perceived in the same way by The Gambian's health workers, which is a considerable challenge in realizing such systems within the country's health care system. However, the authors believed when health care workers are sensitized that MAI systems are not here to replace them; instead, to make them more efficient and productive in their work, this challenge could easily be lessened.
-
Inadequate Financial Support and Lack of Necessary Data for the MAI Systems:
The Gambia has been an underdeveloped country, with only 6% of its GDP spent on the health care system. The dream of implementing such revolutionary and cost demanding technologies can be seen as merely unfeasible. Similarly, the country's health care ecosystem is not adequate to provide the data needed by these revolutionary
technologies, which generally requires a vast amount of quality data to make informed decisions. Therefore, local governments and international organizations' intervention to financially support and provide these MAI technologies with experts to operate them will be of great significance [2]. Such will ensure equity in health care access by citizens and drastically improve the quality and efficiency of the country's primary health care needs. In closing, despite the challenges mentioned above, the authors of this paper believed the motivation outweighs the problems and that the government, citizens, local government, and organizations should all take it as a priority to save The Gambia's health care system.
-
-
METHODOLOGY
First of all, to determine the eligible articles and materials for inclusion in this evaluation, the authors conducted a systematic review of research papers ranging from the year 2003 until 2020 from various digital databases such as IEEE Xplore, ScienceDirect, Pubmed, Web of Science, and other valuable resources using keywords such as health care system, Clinical Decision Support Systems, Medical Artificial Intelligence, Telemedicine, Precision Medicine, and Wearable Devices. Consequently, the authors identified the number of one hundred and seventy-five articles, blogs, and white papers from various sources, as mentioned earlier. However, the authors ended up using only twenty-six articles from the identified sources after a systematic process, which is summarized as follows:
Firstly, the authors discard any of the identified sources that were published before the year 2003. The motive of benchmarking 2003 is because of the limited number of research work on the Gambia or underdeveloped countries because little or no work was done on this research for The Gambia. However, most of the articles included in this work were published during the last four or five years. Consequently, one-hundred articles were left for further review
Secondly, the authors skimmed through these identified materials by reading their titles, abstract, methodology, and the results claimed and then concluded eliminating duplicates and unrelated articles to our review, which left us with the number of fifty articles.
Finally, the contents of the remaining articles were comprehensively studied and evaluated. The authors methodically filtered a final number of twenty-six articles for final inclusion in this work based on meeting our eligibility benchmarks. For instance, the remaining articles provide us with the necessary information to complete this research work, focused on MAI, health care systems, and the paper written in English. Fig 3 is a summarized illustration of the selection process and the benchmarks.
Fig 3: Article Selection and Benchmarks Flowchart.
the Health System in The Gambia:Overview, Medical
-
CONCLUSION
Improving the easy availability, efficiency, affordability, equity, and quality health care within The Gambia can be accomplished using Medical Artificial Intelligence technologies. Consequently, this paper evaluates the adoption of these technologies within The Gambia's health care system by discussing the motivation, use cases, and challenges of implementing such technologies. Additionally, based on the technological and other infrastructural challenges faced by The Gambia, the authors recommend specific feasible MAI technologies for adoption to mitigate these challenges and therefore enhance equity and improved health care services. Also, further research is needed to understand the problems and proposed solutions better. Finally, the author ntends to propose and develop a Medical Artificial Intelligence model for adoption within The Gambia's health care system as future work.
ACKNOWLEDGMENT
Special thanks to the experts who contributed to the te , e e i
Products, Health Financing, and Governance Components. Washington, DC: Palladium, Health Policy Plus. 2019.
-
J. Guo and B. Li, The Application of Medical Artificial Intelligence Technology in Rural Areas of Developing Countries, vol. 2, pp. 174181, 2018, doi: 10.1089/heq.2018.0037.
-
A. N. Ramesh, C. Kambhampati, J. R. T. Monson, and
P. J. Drew, Artificial intelligence in medicine, Ann. R. Coll. Surg. Engl., vol. 86, no. 5, pp. 334338, 2004, doi: 10.1308/147870804290.
-
The Next Generation of Medicine: Artificial Intelligence and Machine Learning TM Capital.
-
W. Diprose and N. Buist, Artificial intelligence in medicine: Humans need not apply?, N. Z. Med. J., vol. 129, no. 1434, pp. 7376, 2016.
-
C. Castaneda et al., Clinical decision support systems
mplate's development and all the r view rs for their t me and
insightful contributions. Prof Wang Xue Ming, thanks for the guidance and supervision. Mr. Loum, thanks for the insightful comments and contributions.
REFERENCES
[1] T. W. Sine, J., P.P. and Saint-Firmin, Assessment offor improving diagnostic accuracy and achieving
precision medicine, J. Clin. Bioinforma., vol. 5, no. 1, Dec. 2015, doi: 10.1186/s13336-015-0019-3.
-
H. Singh, A. N. D. Meyer, and E. J. Thomas, The frequency of diagnostic errors in outpatient care: Estimations from three large observational studies
involving US adult populations, BMJ Qual. Saf., vol. 23, no. 9, pp. 727731, 2014, doi: 10.1136/bmjqs-
2013-002627.
-
M. L. Graber and A. Mathew, Performance of a Web-Based Clinical Diagnosis Support System for Internists, J. Gen. Intern. Med., vol. 23, no. S1, pp. 3740, Jan. 2008, doi: 10.1007/s11606-007-0271-8.
-
R. O. Deliberato, L. A. Celi, and D. J. Stone, Clinical Note Creation, Binning, and Artificial Intelligence., JMIR Med. informatics, vol. 5, no. 3, p. e24, Aug. 2017, doi: 10.2196/medinform.7627.
-
M. Uddin, Y. Wang, and M. Woodbury-Smith, Artificial intelligence for precision medicine in neurodevelopmental disorders, npj Digit. Med., vol. 2, no. 1, 2019, doi: 10.1038/s41746-019-0191-0.
-
M. D. Abrà moff, P. T. Lavin, M. Birch, N. Shah, and
J. C. Folk, Pivotal trial of an autonomous AI-based diagnostic system for detection of diabetic retinopathy in primary care offices, npj Digit. Med., vol. 1, no. 1, 2018, doi: 10.1038/s41746-018-0040-6.
-
F. D. Verbraak et al., Diagnostic accuracy of a device for the automated detection of diabetic retinopathy in a primary care setting, Diabetes Care, vol. 42, no. 4, pp. 651656, 2019, doi: 10.2337/dc18-0148.
-
S. Hägg et al., Incidence of stroke according to presence of diabetic nephropathy and severe diabetic retinopathy in patients with type 1 diabetes, Diabetes Care, vol. 36, no. 12, pp. 41404146, Dec. 2013, doi: 10.2337/dc13-0669.
-
R. R. A. Bourne et al., Causes of vision loss worldwide, 1990-2010: A systematic analysis, Lancet Glob. Heal., vol. 1, no. 6, Dec. 2013, doi: 10.1016/S2214-109X(13)70113-X.
-
B. Chaudhry, Computerized clinical decision support: will it transform healthcare?, J. Gen. Intern. Med., vol. 23 Suppl 1, no. 1 SUPPL., pp. 857, Jan. 2008, doi: 10.1007/s11606-007-0432-9.
-
P. Ramnarayan et al., Measuring the impact of diagnostic decision support on the quality of clinical decision making: development of a reliable and valid composite score., J. Am. Med. Inform. Assoc., vol. 10, no. 6, pp. 56372, 2003, doi: 10.1197/jamia.M1338.
-
P. Ramnarayan et al., Validation of a diagnostic reminder system in emergency medicine: A multi- centre study, Emerg. Med. J., vol. 24, no. 9, pp. 619 624, Sep. 2007, doi: 10.1136/emj.2006.044107.
-
M. L. Graber and A. Mathew, Performance of a Web-Based Clinical Diagnosis Support System for Internists, J. Gen. Intern. Med., vol. 23, no. S1, pp. 3740, 2008, doi: 10.1007/s11606-007-0271-8.
-
H. Yan, E. S. Sullivan, W. Jingyu, and X. Shan, AI in Health Care.
-
M. Salam, First-ever: FDA Clears Biobeats wearable watch and patch for non-invasive cuffless monitoring of blood pressure, FLEPS 2019 – IEEE Int. Conf. Flex. Printable Sensors Syst. Proc., vol. 6, no. 1, pp. 146, 2019, doi: 10.1016/j.surfcoat.2019.125084.
-
Biobeat | Start-Up Nation Finder. https://finder.startupnationcentral.org/company_page/ biobeat (accessed Jun. 08, 2020).
-
Global Healthcare: Indias New Health Tablet Performs 30+ PoC Diagnostic Tests. http://www.globalhealth.care/2014/12/indias-new- health-tablet-performs-30.html (accessed Jun. 08, 2020).
-
telemedicine Paves Way to Future SOC Telemed. https://www.soctelemed.com/content/telemedicine- paves-way-to-future-healthcare/ (accessed Jun. 11, 2020).
-
5 Ways Telemedicine is Transforming Healthcare SOC Telemed.
https://www.soctelemed.com/content/5-ways- telemedicine-transforming-healthcare/ (accessed Jun. 11, 2020).
-
O. Wang, Ping An Good Doctor: the First Entrance of Online Health Services of the Group s " Health Strategy ", 2015.
-
I.-O. O. Adepoju, B. J. A. Albersen, V. De Brouwere,
J. van Roosmalen, and M. Zweekhorst, mHealth for Clinical Decision-Making in Sub-Saharan Africa: A Scoping Review., JMIR mHealth uHealth, vol. 5, no. 3, p. e38, Mar. 2017, doi: 10.2196/mhealth.7185.