
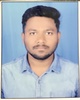
- Open Access
- Authors : Gitesh Kumar Verma , Neha Verma
- Paper ID : IJERTV10IS010179
- Volume & Issue : Volume 10, Issue 01 (January 2021)
- Published (First Online): 30-01-2021
- ISSN (Online) : 2278-0181
- Publisher Name : IJERT
- License:
This work is licensed under a Creative Commons Attribution 4.0 International License
Review Paper on Implementation of Six Sigma and Anylogic Multi-Method Simulation in Manufacturing Industry to Improve Performance and Production Capacity
Gitesh Kumar Verma
Mtech Scholar: Department of Mechanical Engineering SSIPMT, Raipur, Chhattisgarh 492015
Neha Verma
Associate Professor: Department of Mechanical Engineering SSIPMT, Raipur, Chhattisgarh 492015
Abstract Many types of manufacturing industries uses the different types of simulation techniques with different methods either integrated or individual to optimize their performance and increase production capacity. As we know six sigma method have been developed as become improved the capability of process performance and reduce wastage. The idea of this paper is to implementing combination of both six sigma and anylogic simulation in real case manufacturing industry for reduction processing time, improve processes and improve productivity using statistical data analysis and embedding for continues improvement an organization in practice. Here on the six sigma method implemented by DMAIC through over an industry on effectively way however it to identify the problem causing approaches in manufacturing organization alongside anylogic simulation using discrete event simulation (DE) and agent based simulation (AB). This paper also shows the fundamental relationship between six sigma and anylogic simulation in manufacturing line on effectively way.
Keywords Six sigma, Anylogic multi-method simulation, Discrete event simulation, Agent based simulation, DMAIC
-
INTRODUCTION
Manufacturing industries are facing dynamic competitive environment they have to need increase their customers requirement and stand the position in competitive and also they have to do increase their productivity with optimal time and optimized product. Here on implementation of six sigma and anylogic simulation have become helps to meet the reduction process and increase the productivity by statistical data analysis. Simulation technology has progressed continuously in recent times and various new software packages are coming into the market while already existing software packages are being improved and update. Anylogic simulation widely uses in many business or manufacturing industry for effectively progress. The most common part of anylogic simulation in discrete event (DE), agent based (AB), system dynamics (SD) simulation. On our case study we works only with Discrete event simulation and Agent based simulation.
In this paper meet to increase process capabilities and increase the production capacity. If the level of output is
increased faster than that of input, productivity will increase. Conversely, productivity will be increased if the level of input is decreased faster than that of output. Also, an organization may realize a productivity increase from reducing more output with the same level of input. Finally, producing more output with a reduced level of input will result in increased productivity. Productivity is the ratio between output and input. It is quantitative relationship between what we produce and what we have spent to produce. Productivity is nothing but reduction in wastage of resources like men, material, machine, time, space, capital etc.
-
LITERATURE REVIEW
-
Six sigma
S.V. Deshmukh and Ashish Chavan (2017) reviewed of literature is based on evaluation and utilization of six sigma in small medium sized enterprises. The methodology adopted for content analysis and implemented six sigma pertaining in general in industry. The literature reviewed shows the management commitment is most important for success of an organization and advised to specially small medium sized industry to maintain the quality culture management
Suraj Dhondiram, M.M. Ganganallimath, Roopa B Math, Yamanppa Karigar (2015) this literature is based on reduce defects and quality improve by six sigma (DMAIC) method in green sand casting process. On this use design of experiment (DOE) and analysis of variance (ANOVA) techniques combined to analyzed statistical data. In this paper the existing and proposed process has been capered for getting optimal value which needed to get.
Ali Ahmed, J.F. Olsen, and Jhon Page (2017) this research paper worked on combination six sigma and anylogic simulation method. The concept of combining six sigma and anylogic simulation leading the companies for more effectively. Integrating of six sigma and anylogic simulation fundamental is not only for manufacturing industry but also for work it on many business. The objective of this paper is to reduce the defects, improve the quality and increase the
productivity with less time by using decision making by six sigma and statistical data analysis by anylogic.
Fig. 1. Six sigma (DMAIC) process.
-
Discrete event simulation
Pavan Kumar Narayanan, M.S. and Lorena D. Mathien (2016) This literature paper is based discrete event simulation modelling and engineering technique on metal manufacturing mill for solving their shorter product life system, identify bottleneck process and short term budgeting problems and also work on to promotes its use in practice.
Jawad Elomari, Stefan U. Svensson, Kerstin Olsson (2018) this literature has worked for excellency of automation manufacturing assembly line analysis of processing times using the discrete event simulation method by anylogic software. Here on worked in four automation manufacturing industry for strategies and existing state of art technology into new framework.
N. Prajapati, C. Turner, W. Wutabarat (2020) reviewed is based on discrete event simulation for decision making in real time for production line. The frame work of using discrete event simulation for manufacturing optimization process with the objective of improve the quality and cost. The literature has identify key research gaps where the multi objectives optimization method have been applied. The research shows the resource allocation where model provides the accurate prediction data.
Fig. 2. DE simulation flow diagram.
-
Agent based simulation
Gizem Gunes (2014) research paper has worked for enhance the capability of Agent based simulation in anylogic software module. In which described the aspect of agent based simulation where we can use, how we can use, what are criteria of its etc. This paper is full with learning knowledge of agent based simulation in anylogic software.
Luminita Parv, Bogdan Deaky, Marius Daniel Nasulea and Gheorghe Oancea (2019) literature paper is based on value floe to approaches lean strategy, focusing and identify non- value added activity for controlling. In this paper manufacturing system has considered to increase its customer demands. Although the research worked has done in the automotive industry for a medium sized enterprise. Here on lean principle, value stream mapping method and agent based simulation implemented for optimization production process parameter.
Charles Macal, Michael North (2014) this literature is based on approaches to modeling system individual, autonomous. In this to working on predicting the success of market by supply chain modeling through agent based simulation in which the brief tutorial discussed to introduced the agent based simulation.
Fig. 3. AB simulation flow diagram
-
Mult-method simulation
Andrei Borshchev (2014) this paper is based on describing the the main three modeling discrete event simulation (DE), agent based simulation (AB) and system dynamics simulation (SD) of anylogic software. This is defining the dependency for ses in aspects in DE, AB, and SD. The multi method simulation meets the offers the different method with in one methos this works in many field like supply chain, consumer market, manufacturing portfolio etc.
R. Sadeghi, S. Dauzere-Peres, C. Yugma (2016) This paper is based on implementation of multi method simulation in order to validate the approaches to semiconductor manufacturing process. The discrete event and agent based simulation has implemented to extracting the performance of the real case industry process. On the other hand detecting problem related manufacturing process line and applying the strategies and objectives in organization.
Kanse Ashish Balasaheb (2019) review paper is based on for design and developing the different activities and asserting the simulation work in the manufacturing industry. In which used to measure the performance of plant and understand the existing plant behavior. By using simulation method advantages can be achieved such as time, cost, productivity an effectively way.
Onkar Bhandare (2016) literature paper has worked on towards the decreasing the peak load capacity of electricity power station by the considering to developed discrete event simulation at multiple station for production schedule optimization later that implement agent based simulation for considering renewable distribution generation and time of use electricity pricing minimizing. The simulation work has based on to promoting the renewable energy sources with effectively amount.
-
-
FRAMEWORK TO TNTEGRATED SIX SIGMA AND MULTI- METHOD SIMULATION (DE, AB) IN MANUFACTURING
INDUSTRY
There are mainly four approaches of framework for success for research in which Measure phase, Analyze phase, Improve phase and Control phase.
Measure phase focus on understanding root cause problem during implementing input and finalize getting output. On the other hand create interaction in internal variable. In this phase to measure the root causing problem like organization interaction for six, define the manufacturing flowchart for DE modeling and analyze the behavior of element or agent for AB modeling.
Analyze phase is come up potential solution data by processing work. Here on six sigma worked by DMAIC tools in organization, DE and AB modeling go through the Statistical data.
Improve phase will work to put the process or strategies after the analyze phase. In this the changes will work on the DE AB simulation throughout the flowchart. This is an
important phase to enhancing the capabilities of the process flow work.
Finally the Control phase will test the long term process of work flow to validate the output resultant data of simulation work.
-
RESEARCH GAP
There are many research has been done to study on Six sigma and Multi-method simulation for enhancing the process capabilities and productivity improvement for an business and manufacturing industries.
But There is only few research work has been establish in terms only with small medium sized manufacturing industry using six sigma and multi-method (DE and AB) simulation for increase process capabilities, manufacturing process time, and productivity and its analysis. This is for a real case industry to enhance their organizational goal related to high productivity with less time and meet to this integration in practice in manufacturing industry.
-
CONCLUSION
Considering the statistical data of simulation of the manufacturing process related to processing time and product quantity of existing system before and after the implementing six sigma (DMAIC) method. Here on the organizational goal meet by the integration of six sigma and Multi method simulation.
The key improvement in organization are:-
-
To enhance productivity
-
To reduce un-necessary cycle time
-
To reduce the un-necessary processes
-
To optimize the organization management performance (men, machine and power).
REFERENCES
-
Ali Ahmed, J.F. Olsen, and Jhon Page,, Process Improvement Based on an Integrated Approach of DMAIC and Multimethod Simulation, International confernce on Modeling and Simulation, Hobert,Australia, 2017
-
S.V. Deshmukh and Chavan, A. Six Sigma and SMEs: a critical review of literature, International Journal of Lean Six Sigma, 157-167
-
Suraj Dhondiram, M.M. Ganganallimath, Roopa B Math, Yamanppa Karigar, Application of Six Sigma Method to Reduce Defects in Green Sand Casting Process: A case Study, IJRMEE, ISSN 2349- 7947, 2015
-
Ali Ahmed, J.F. and Jhon Page, Adopting Lean Six Sigma to AnyLogic Simulation in a Manufacturing Environment, Conference:
Modelling and Simulation At Gold Coast, Australia, November 2015
-
R. Sadeghi, S. Dauzere-Peres, C. Yugma, A Multi-Method Simulation Modelling for Semiconductor Manufacturing, IFAC Paper, 727-732,
December 2016
-
Dmitry Ivanov, Operation and Supply Chain Simulation with Anylogic, 2017
-
Andrei Borshchev Multi-method modelling: AnyLogic, 248-279,
April 2014
-
Emile Zankoul, Rita Awwad, Hiam Khoury, Evaluation of Agent- Based and Discrete-Event Simulation for Modeling Construction Earthmoving Operations, Conference: Conference: 32nd International Symposium on Automation and Robotics in Construction and Mining At: Oulu, Finland, June 2015
-
Pavan Kumar Narayanan, M.S. and Lorena D. Mathien,, A Metal Manufacturing Mill Uses Discrete-Event Simulation to Optimise Operations, European Journal of Economic and Business, ISSN 2456- 3900, June 2016
-
K. Khedri, H. El Maraghy, M. Hanafy, S.N. Samy, A Framework For Modelling Reconfigurable Manufacturing System Using Hybridized Discrete-Event and Agent based Simulation, IFAC, 2015
-
Jawad Elomari, Stefan U. Svensson, Kerstin Olsson, The Role of Simulation Optimization in Process Automation for Discrete Manufacturing Excellence, Proceedings of the 2018 Winter Simulation Conference, December 2018
-
N. Prajapati, C. Turner, W. Wutabarat, Real-time Discrete Event Simulation: A Framework for an Intelligent Expert System Approach Utilising Decision Trees, The International Journal of Advance Manufacturing Technology, ISSN 2893-2911, 2020
-
Luminita Parv, Bogdan Deaky, Marius Daniel Nasulea and Gheorghe Oancea, Agent-Based Simulation of Value Flow in an Industrial Production Process, Processes 02, 2019
-
Gizem Gunes, Agent Based Simulation and an Example in Anylogic, Yldz Technical University, Industrial Engineering Department,
Istanbul, 2014
-
Charles Macal, Michael North, Introductort tutorial; Agent Based Modeling and Simulation, IEEE, 2014
-
Onkar Bhandare, Simulation Based Electricity Demand Response Considering Product Sequence and Onsite Renewable Energy, University of llinois at Chicago,2016
-
Virginia Fani, Bianca Bindi, Romeo Bandinelli, Balancing Assembly Line with a Simulation Optimizational: A Case Study in the Footwear Industry , Conference Paper at 34th International ECMS, June 2020
-
Khalid Nagadi, Luis Rabelo, Mohammed Basingab, A Hybrid Simulation-based Assessment Framework of Smart Manufacturing System, International Journal of Computer Integrated Manufacturing 1-14, 2017
-
Kanse Ashish Balasaheb, Review Paper on Manufacturing System Performance Improvement by Modeling and Simulation, IRJET, ISSN 2395-0056, December 2019
-
Stefan Treber, Christoph Bubeck, Gisela Lanza, Investigating Casual Relationship between Disruption, Product Quality and Network Configuration in Global Production Network, Procedia CIRP, ISSN 202-207, 2018
-
WoonSeong Jeong, Soowon Chang, JeongWook Son and June Seong Yi, BIM- Integrated Construction Operation Simulation for Just in time Production Management, Sustainability, 2016