
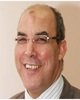
- Open Access
- Authors : M. H. El-Axir , Y. A. Mashal , N. K. Taha , Asmaa A. Rashed
- Paper ID : IJERTV10IS100188
- Volume & Issue : Volume 10, Issue 10 (October 2021)
- Published (First Online): 08-11-2021
- ISSN (Online) : 2278-0181
- Publisher Name : IJERT
- License:
This work is licensed under a Creative Commons Attribution 4.0 International License
Single and Multi-Optimization of Roller Burnishing Process Parameters for AL-Alloy 7075
M. H. El-Axir
Department of Production Engineering and Mechanical Design, Faculty of Engineering,
Shebin El-kom, Egypt
Y. A. Mashal
Department of Production Engineering and Mechanical Design, Faculty of Engineering,
Shebin El-kom, Egypt
Abstract In the present experimental investigation, Single and multi-optimization of three methodologies (Artificial Neural Network (ANN), Response surface methodology (RSM) and Taguchi method) for prediction of the results of roller burnishing process parameters on surface roughness, surface micro hardness, surface out of roundness, and change in diameter for AL-alloy 7075 have been studied. Its known that the part's performance is strongly influenced by the surface quality and burnishing process is one of the most significant surface finishing processes. It has been increasingly applied in manufacturing due to its several advantages that are lacked in other finishing processes. Roller Burnishing is a cold working process which produces a fine surface finish by the planetary rotation of hardened rolls over a bored or turned metal surface. This work studies the effect of burnishing speed, number of passes, depth of penetration and burnishing feed on surface roughness, surface microhardness, change in diameter, and surface out of roundness of AL-alloy 7075. The experiments are designed based on Taguchi experimental design technique.
The results obtained have shown that the best network is 4- 50-1 for both average surface roughness (Ra) and microhardness (HV), 4-30-1 for surface out of roundness (OR), and 4-40-1 for change in diameter (D). In the case of multi-response optimization, the ANN architectures 4-40-4 was found as the best networks in this investigation.
Keywords(Single and multi-optimization; Roller burnishing; Surface roughness; Taguchi technique; ANN; RSM;)
-
INTRODUCTION
In todays world, the manufacturing of machines and other components with the highly finished surfaces are becoming more and more important. Drastic attention is being given on the quality of the surfaces. The quality of the surface is very important for a large number of components which utilized in aerospace, chemical and nuclear industries, that spend their life working under critical conditions such as high temperatures, cyclical loading etc. [1,2,3]. Burnishing process have several advantages that are lacked in the other finishing processes. One of the most important advantages of burnishing process that it is more efficient when compared to lapping, grinding, and polishing techniques. It can be used to create a mirror-like surface finish on nonferrous and ferrous materials [4,5].
N. K. Taha
Department of Production Engineering and Mechanical Design, Faculty of Engineering,
Shebin El-kom, Egypt
Asmaa A. Rashed
Department of Production Engineering and Mechanical Design, Faculty of Engineering,
Shebin El-kom, Egypt
Also, it can be said that the prime advantage of burnishing process is its ability to minimize surface roughness, change in diameter, surface out of roundness and maximize the surface microhardness of the workpiece [6,7,8]. Surface finish is one of the most important quality parameters for ensuring that manufactured components conform to specified standards [2,9,10]. The most important parameters that have an effect on burnishing process are burnishing speed, burnishing feed, number of tool passes, and depth of penetration [11,12,13]. The surface roughness decreases with the increase of burnishing speed firstly, but as the burnishing speed is increased, its increases. [14,15]. An increase in feed considerably reduces the surface roughness [14,16]. When number of passes increases the surface roughness decreases first and then starts to increase with number of passes [17]. But with the increase of number of passes, the surface roughness increases [15]. The surface roughness firstly decreases by increasing the burnishing depth, then the surface roughness increases as the burnishing depth is increased [18]. On the other hand, according to [19,20], higher burnishing depth results in more plastic deformation, leading to lower roughness.
When the burnishing speed and burnishing feed are increased, the material's surface hardness gradually decreases. [14,15]. The increase in pass number and burnishing depth significantly increases the surface hardness, however, a further burnishing depth leads to decrease of surface hardness [6,7,19].
The increase in burnishing speed decreases out-of- roundness error [21], conversely, the increase in the burnishing feed causes high out-of-roundness error [6]. The out-of-roundness error increases as the pass number increases until it reaches to highest value, by more increasing in pass number, the error of roundness decreases [6]. The out-of- roundness decreases first as the depth of penetration increases, until it reaches to lowest value. Then the out-of-roundness starts to increase gradually with more increasing in depth of penetration [22].
The increase in burnishing speed and burnishing feed result in decreasing change in diameter and increasing in depth of penetration which lead to reduction in the change in diameter [23,8].
-
EXPERIMENTAL WORK
-
Workpiece Material
The workpiece material utilized in this research was 7075 aluminum alloy. Table1 and Table2 show the chemical composition in weight percent and the significant mechanical properties, respectively.
TABLE 1. CHEMICAL PROPERTIES OF 7075 ALUMINUM ALLOY
Chemical Composition
Alloy
Cu
Fe
Mn
Mg
Si
Zn
Cr
Ti
Ni
Pb
Bi
Al
Al- 7075
1.2-
2%
0.5
0.3
2.1-
2.9
0.4
5.1-
6.1
0.18-
0.28
0.2
–
–
Ti+Zr 0.25
Balance
TABLE 2. MECHANICAL PROPERTIES OF 7075 ALUMINUM ALLOY
Mechanical Properties
Tensile Strength
Shear Strength
Elongation A5 (%)
HV
Fatigue limit
Al-7075
580 MPa
331 MPa
10
157
160 MPa
The choosing of this material is due to their importance in manufacturing and their susceptibility to degradation during the machining process, through surface and subsurface damage. Alloy 7075 is one of the highest strength aluminum alloys with zinc as the principal alloying element. 7075 has excellent fatigue resistance. It has an excellent strength-to weight ratio than that of steel, and it is ideally used for highly stressed aircraft parts [24].
-
Workpiece Preperation
The as-received materials were first machined into short bars workpieces, external diameter of 50 mm. Workpieces were machined with the desired dimensions as illustrated in Figure 1. The workpieces were then machined with five recesses, enabling each specimen to be utilized in 2 conditions at parts A and B, Section A was burnished but section B was remained unburnished to comparison with section A. Initial turning conditions wee unified for all workpieces as rotational speed 1200 rpm, depth of cut 0.05 mm and feed = 0.1 mm/rev.
Figure 1. Workpiece geometry (Dims. in mm)
Turning and burnishing processes are carried out on Computer numerical control (Z-MaT STAR SL6 CNC) lathe. The tool is changed with a single roller carbide burnishing tool after turning process.
The experimental work was carried out with a single roller burnishing tool, as illustrated in Figure 2. It can be used on lathes or similar machines to roll uniform and complete inner and outer cylindrical and conical surfaces of carbon steel, alloy steel, non-ferrous metal cast iron and other parts with hardness HV <335.
Figure 2. Single roller burnishing tool
-
Experimental Design
The Taguchi philosophy is a useful technique for creating production system with high-quality. Taguchi philosophy is a strategy constructed according to orthogonal array (OA) experiments, that produce substantially lower variance for obtaining an optimum experiment setting with controlled process parameters. Burnishing speed (N), number of passes (np), depth of penetration (d), and burnishing feed (f) were chosen at five levels in this investigation as the four control parameters. The burnishing parameters are given in Table 3. So, the total number of degrees of freedom of the control variables is equivalent to 25. Taguchis (L25 OA) orthogonal array (Table .3) was utilized in current study.
TABLE 3. L25 ORTHOGONAL ARRAY
Exp. No.
N (speed, rpm)
np, (No. of passes)
d, (depth of penetration,
)
f, (feed,
/rev)
Code
Actual
Code
Actual
Code
Actual
Code
Actual
1
1
200
1
1
1
25
1
25
2
1
200
2
2
2
55
2
50
3
1
200
3
3
3
85
3
75
4
1
200
4
4
4
115
4
100
5
1
200
5
5
5
145
5
125
6
2
400
1
1
2
55
3
75
7
2
400
2
2
3
85
4
100
8
2
400
3
3
4
115
5
125
9
2
400
4
4
5
145
1
25
10
2
400
5
5
1
25
2
50
11
3
600
1
1
3
85
5
125
12
3
600
2
2
4
115
1
25
13
3
600
3
3
5
145
2
50
14
3
600
4
4
1
25
3
75
15
3
600
5
5
2
55
4
100
16
4
800
1
1
4
115
2
50
17
4
800
2
2
5
145
3
75
18
4
800
3
3
1
25
4
100
19
4
800
4
4
2
55
5
125
20
4
800
5
5
3
85
1
25
21
5
1000
1
1
5
145
4
100
22
5
1000
2
2
1
25
5
125
23
5
1000
3
3
2
55
1
25
24
5
1000
4
4
3
85
2
50
25
5
1000
5
5
4
115
3
75
-
-
MEASUREMENTS
-
Surface Roughness
The surface that results from turning operation contains irregularities. One of causes of this irregularities is the surface roughness that result from the mark left by the tool itself, due to the tearing of the material during turning operation, as a result of small irregularities in the tool tip and debris of a built up edge. So that, burnishing process was applied to reduce the irregularities of such a surface. The surface roughness factors (arithmetic average Ra, root-mean-square Rq, the maximum peak to valley height Rt and Ten points height Rz) values were measured by using (Mitutoyo surftest.301) shown in Figure 3 and the cut-off length was taken as 0.75mm. It should be pointed out here that for each test surface roughness was measured at 3 different positions around the workpiece. The average of the three measurents was reported as the final results.
Figure 3. Mitutoyo surftest-301
-
Surface Microhardness
a microhardness tester (MITUTOYO) was employed to measure the microhardness (Figure 4). The component is securely mounted on the V Block, and a 10 N force was applied to the workpiece using a diamond indenter. The load was applied for 15 seconds. when the load had been lifted, the workpiece was examined under a microscope (40X magnification). The surface indentation's 2 diagonals were measured. The Vickers Pyramid Number (HV) is the measure of hardness which was determined by the test. The arithmetic average of three readings was obtained for better results.
Figure 4. Vickers micro-indentation hardness
-
Surface Out-of-Roundness
The roundness of the surface was measured before and after burnishing process. Taylor Hobson precision – TALYROND 73 which is shown in Figure 5. was used for these measurements. An arithmetic average of three readings was obtained for better results.
Figure 5. Taylor Hobson precision – TALYROND 73
Change in the Workpiece Diameter
The diameter of each sample was measured by using (Axiom Too) Coordinate measuring machine (CMM) as shown in Figure 6. About 150 points were taken for each specimen. By using Microsoft excel the change in diameter has been calculated by Subtraction the value of the diameter of specimen after burnishing process from the value of diameter of specimen after turning.
Figure 6. Coordinate measuring machine (CMM)
-
-
EXPERIMENTAL RESULTS
Table 4. illustrates the arrangement and the output results of the twenty-five experiments carried in current study using the Taguchi design matrix in the case of four roller burnishing parameters and five levels of each one. These results are used to deduce the single and multi- optimization using different methodologies which is one of the main aims of this investigation. Also, these results are utilized to develop mathematical models that relate each
response to the burnishing input parameters through response surface methodology (RSM).
TABLE 4. EXPERIMENTAL DESIGN MATRIX AND RESULTS FOR ROLLER BURNISHING RESPONSES FOR AL-7075 ALLOY
Exp. No.
Burnishing process parameters
Surface roughness
Surface micro- hardness
Surface out of roundness
Change in diameter
N,
rpm
np
d,
m
f,
m/rev
Ra, m
HV
OR, m
D, m
1
200
1
25
25
0.260
180
27.76
34
2
200
2
55
50
0.230
227
20.07
180
3
200
3
85
75
0.246
246
18.77
270
4
200
4
115
100
0.333
229
16.78
316
5
200
5
145
125
0.428
202
19.12
320
6
400
1
55
75
0.175
230
15.57
120
7
400
2
85
100
0.185
238
11.39
150
8
400
3
115
125
0.238
227
7.30
190
9
400
4
145
25
0.460
228
10.49
255
10
400
5
25
50
0.396
220
10.56
205
11
600
1
85
125
0.269
219
4.96
95
12
600
2
115
25
0.312
240
8.57
170
13
600
3
145
50
0.247
244
4.51
296
14
600
4
25
75
0.237
239
9.75
175
15
600
5
55
100
0.219
241
7.10
214
16
800
1
115
50
0.242
235
4.41
220
17
800
2
145
75
0.158
242
6.66
285
18
800
3
25
100
0.185
235
17.04
110
19
800
4
55
125
0.205
235
14.35
105
20
800
5
85
25
0.303
245
12.16
190
21
1000
1
145
100
0.270
221
7.74
329
22
1000
2
25
125
0.296
200
25.82
112
23
1000
3
55
25
0.269
232
22.55
138
24
1000
4
85
50
0.136
248
16.89
228
25
1000
5
115
75
0.059
245
16.93
320
-
COMPARISON OF THREE METHODOLOGIES PREDICTED RESULTS AND ERRORS
In todays complex and competitive economy there is a need to improve the efficiencies of roller burnishing processes. Such efficiencies may be attained by understanding the relationship between the outputs of burnishing processes and burnishing variables through several methodologies and to determine the best methodology that has the minimum deviation between the experimental results and the predicted one. This will be a good tool for production engineers to use the best methodology for predicting the result according to the combination levels of input burnishing parameters before carrying out the experiment depending on the desired result.
Before presenting the comparison between the experimental and the predicted results of the three methodologies used in this investigation, a brief discerption of each methodology is presented in the following paragraphs.
At this investigation, the Taguchi technique utilized for determining the optimal burnishing parameters and predicted results for decreasing the surface roughness to the minimum value, maximum micro-hardness, minimum surface out of roundness, and minimum change in diameter in burnishing operation. Corresponding to Taguchi technique, the loss function was utilized for computing the deviation among the desired value and actual value. The loss function is converted to a signal-to-noise ratio. There are three S/N ratios existing based on the kind of characteristics of the output parameters; lower is better (LB), nominal is better (NB) and higher is better (HB). For HB and LB, the explanations of the loss function for burnishing performance produces yi of n repeated number are:
-
Smaller is the Better
LB = (S/N) LB = (1)
-
larger is the Better
HB = (S/N) HB = (2)
Where: yi in the experimentally observed result of the experiments
A low surface roughness, low change in diameter, and low out of roundness are alway desirable for better product performance. As a result, the surface response parameter such as surface roughness, change in diameter, and surface out of roundness have been classified such as lower is better type problem and the S/N ratio in this condition has been computed as (Ra), (OR) and (D). In addition, a high micro- hardness is desirable. As a result, this output parameters have been classified as higher is better. It should be mentioned that in the burnishing process, larger value of (Ra), (OR), (D) and (HV) is desired. A greater value of is corresponded to a better performance, whatever the category of the performance characteristics is. So that, the optimum level of burnishing factors is the level which the value of is the greatest. mathematical models that relate burnishing response (surface roughness factors, surface microhardness, surface out of roundness, and change in diameter of the workpiece) to burnishing factors have been proposed using the Response Surface Methodology (RSM) and the observed values of the responses can be seen in Table 5. The experimental data is evaluated and analyzed by using a polynomial response surface of second order in terms of process parameters by determining their interactions. To obtain the regression for every model, the system of equations was solved, the student's t-test was used to eliminate the less- significant coefficients from any more analysis. The predicted results of each response have been obtained though the deduced mathematical models. Table 5 shows the response surface for surface roughness factors, surface microhardness, change in diameter, and surface out of roundness as a function of the four parameters utilized in current study for Al-7075.
TABLE 5. DEDUCED MATHEMATICAL MODELS FOR DIFFERENT RESPONSES ACCORDING TO RSM METHODOLOGY
Mathematical Models
1
Ra=
0.2654 + 0.000293 N + 0.0785 np + 0.000657 d –
0.006199 f + 0.00764 np*np + 0.000002 d *d +
0.000034 f *f – 0.000151 N *np – 0.000001 N *d +
0.000002 N *f – 0.000292 np*f
2
HV=
102.06 + 0.1206 N + 22.05 np + 0.9305 d + 1.043 f –
0.000086 N*N – 3.114 np*np – 0.004937 d*d –
0.007451 f*f
3
OR=
47.98 – 0.09255 N – 4.330 np – 0.0977 d + 0.000073 N
*N + 0.000982 d *d + 0.00642 N *np – 0.000203 N*d
4
D=
-114.5 – 0.1815 N + 50.79 np + 1.2763 d + 4.717 f+ 0.000273 N *N – 0.03242 f *f – 0.04852 N *np
Taking into account the needs of a fast-growing and rapidly increasing manufacturing industry, researchers developed new alternative techniques in order to prevent or avoid deviation in prediction, a technique that operates extremely different from traditional simulation techniques. This technique is known as Artificial Neural Network (ANN). It is a novel technology. It works according to the philosophy of biological neural systems. This technique immediately became well-known, and it is now widely used in the manufacturing to solve real-world problems. Artificial Neural Network (ANN) is a type of computational modelling that is inspired by the human brain's neural structure. The Artificial Intelligence (A.I) is one of the most widely used nonlinear mapping systems. This technique is essentially utilized for 2 applications: classification and prediction. Training determines the pattern between input and output data for the neural network.
It should be pointed out here that before applying Artificial Neural Network (ANN), the artificial neural network structure, different input parameters, and output parameters must initially be defined. So that, optimizing the ANN model and its different parameters is essential. Finding the optimal structure for ANN is considered a major investigation topic. It contains the following information: (1) number of hidden layers, (2) number of hidden neurons at every hidden layer, and (3) number of connections and activation function for each neuron. The choosing of these attributes is commonly done utilizing trial-and-error process.
Artificial Neural Network (ANN) uses data obtained from experimentation in order to predict the outputs. In the present investigation, Neural Network Analysis, data gathered from burnishing experiments were separated into 3 groups, with 70% of datasets chosen at random for neural network training, 15% for testing, and the remaining 15% for validation. Aim of training the neural network is to make it more evolved and have strong relation with Inputs and outputs. The network construction contains 1 input layer, 1 hidden layer, and 1 output layer. the Artificial Neural Network modeling, which carried out in this study, done using multi-layered perception (MLP) by MATLAB software. After many attempts, changing
number of neurons from 5 to 50 in the hidden layer and through each attempt some of training is carried out, various ANN models are chosen for comparison by detecting the best ANN performance and the correlation coefficient. It should be mentioned here that the changing in the number of neurons and number of training were carried out for obtaining the best network for each response studied in this investigation. The Artificial Neural Network models are labeled with the neurons number in every layer, and the neurons number in the hidden layer is optimized. For illustration, model MLP 4- 10-1 has 1, 4, and 10 neurons in the output, input, and hidden layers, correspondingly.
The statistical values for the Artificial Neural Network model, Correlation Coefficient (R), and Mean Square Error (MSE) are utilized as the foundation for detecting the performance of the neural network. The R value shows the degree of agreement among measured values and predicted values. Figures 7-10. represent the comparisons of actual results and predicted results of the different responses for all data and the mean absolute percentage error of each methodology.
It can be seen from these figures that the mean percentage errors of ANN are the minimum value for the whole responses, which indicate that the Neural network models produce high agreement of the prediction results with a correlation coefficient.
Figures 7. Experimental and predicted results and MPE of three methodologies for surface roughness
Figures 8. Experimental and predicted results and MPE of three methodologies for surface microhardness
Figures 9. Experimental and predicted results and MPE of three methodologies for surface out of roundness
Figures 10. Experimental and predicted results and MPE of three methodologies for change in diameter
-
-
SINGLE AND MULTI-OPTIMIZATION OF ROLLER BURNISHING PROCESS
-
Taguchi Method
– Single Optimization Results
To determine the best roller burnishing process settings, single response optimization is used to decrease surface roughness factors, change in diameter, and surface out of roundness while maximizing surface microhardness individually. Surface roughness factors, out of roundness, surface microhardness, and change in diameter main effect plots (MEP) are plotted in Minitab 19 software to determine the optimum influence of roller burnishing process input parameters as burnishing speed, number of passes, depth of penetration, and burnishing feed. For determining the optimum condition of roller burnishing process for each response, the experimental output results are converted to a signal-to-noise (S/N) ratio with the category of the-smaller- the-better which utilized to compute the S/N ratio quality characteristics of surface roughness factors, change in diameter, and surface out of roundness diameter. However, the S/N ratio quality characteristics of hardness are calculated using the larger-is-better category. The results of signal to noise ratio and the main effect plot of each response studied in this investigation arepresented in Table 6 and Figure 11.
At this research, according to the Taguchi method, the S/N ratio should have a maximum value to achieve optimal cutting condition. Table 6 shows the optimum combination of the input burnishing factors that lead to the best (optimal) result of each response. For example, the lowest value of surface roughness can be achieved at burnishing speed of 1000 rpm, number of passes of 2, depth of penetration of 115 m and feed of 75 m/rev.
TABLE 6. SINGLE RESPONSE OPTIMIZATION OF DIFFERENT RESPONSES ACCORDING TO TAGUCHI METHODOLOGY
Response
Roller burnishing parameter
Optimum Value
N, rpm
np
d, m
f, m
Ra
1000
2
115
75
0.091
Hv
800
3
85
75
265.04
OR
600
1
145
50
0.412
D
800
1
25
25
22.96
– Multi-Response Optimization
Single response optimization is not ideal for industrial applications. The burnishing process must be optimized in the light of the priorities given to various responses depending on the application. Optimization for a single response may lead to results that have a variety of impacts on other responses. As a result, multi-response optimization is used in this study to minimize surface roughness factors, out of roundness, and diameter change while maximizing surface micro-hardness based on the importance given to various responses. Response surface methodology, Grey relational Taguchi technique, response surface methodology and artificial neural network are used to perform multi response optimization.
Surface roughness, Ra
Surface hardness, HV
Surface out of roundness, OR
Change in diameter, D
Figure 11. Main effect plot for S/N ratio of studied responses
– Optimization of Process Parameters Using GRA Based on Taguchi Method.
Table 4. shows the results of Ra, OR, HV, and D according to the experimental conditions. These response values are transformed to grey scale coefficient and GRG. Normalized values, Grey Relation coefficient, the values of GRGs and ranks are shown in Table 7. A higher GRG introduces a better response in the process parameters. Among all the experiments, the best multiple performance characteristics is the 13th experiment in Table 7 due to the fact that it has the highest grey relational grade (GRG). By another phrase, optimization of the complicated multiple performance parameters can be transformed to the optimization of a single grey relational grade.
N3np3d5f2 are the optimum parameters for multi response optimization that simultaneously minimize surface roughness, maximize surface microhardness, minimize surface out of roundness, and minimize change in workpiece diameter. For Al-7075, the GRG produced is 0.691. the optimum processing parameters in roller burnishing are burnishing speed of 600 rpm, number of passes of 3, depth of penetration of 145 m and burnishing feed of 50 m/rev which would lead to minimum surface roughness, maximum surface microhardness, minimum change in workpiece diameter, and minimum surface out of roundness.
-
Response Surface Methodology
– Single Optimization Results
Response Surface Methodology is a sequential technique which facilitates approaching optimal region and illustrates the response efficiently. Response Surface Methodology has been proven its efficiency in optimizing the burnishing process parameters for surface roughness, change in diameter, surface microhardness, and surface out-of-roundness. Single response optimization determines how input parameters influence individual response desirability. The numerical optimization identifies a position where the desirability function is maximized.
Table 8. shows the optimum combination of the input burnishing parameters that lead to the best (optimal) result of each response. For example, minimum surface roughness can be obtained at burnishing speed of 1000 rpm, number of passes of 5, depth of penetration of 145 m and feed of 90.6566 m/rev.
TABLE 8. SINGLE RESPONSE OPTIMIZATION OF DIFFERENT RESPONSES ACCORDING TO RSM
Al-7075 alloy
Response
Roller burnishing parameter
Optimum Value
N, rpm
np
d, m
f, m
Ra, m
1000
5
145
90.6566
0.0295
HV
701.010
3.545
94.090
70.4545
263.620
OR, m
765.657
1
129.242
–
4.393
D,m
418.182
1
25
125
2.785
– Multi Optimization Results
The burnishing factors were optimized using Minitab's response surface optimization software. Letters N, np, d and f represent coded values of burnishing rotational speed, number of passes, depth of penetration, burnishing feed, respectively.
TABLE 7. EVALUATED GREY RELATIONAL COEFFICIENTS AND GRADES AND RANKS FOR 25
Input Parameters
Output Parameters
The Normalized
Gray Relation Coefficient
GRG
Rank
N,
rpm
np
d,
m
f,
m
Ra,
m
HV
OR,
m
D,
m
Ra
HV
Or
D
Ra
HV
OR
D
1
200
1
25
25
0.260
180
27.76
34
0.499
0.000
0.000
1.000
0.500
0.333
0.333
1.000
0.542
17
2
200
2
55
50
0.230
227
20.07
180
0.574
0.691
0.329
0.505
0.540
0.618
0.427
0.503
0.522
20
3
200
3
85
75
0.246
246
18.77
270
0.534
0.971
0.385
0.200
0.518
0.944
0.448
0.385
0.574
16
4
200
4
115
100
0.333
229
16.78
316
0.317
0.721
0.470
0.044
0.423
0.642
0.486
0.343
0.473
23
5
200
5
145
125
0.42
202
19.12
320
0.079
0.324
0.370
0.031
0.352
0.425
0.442
0.340
0.390
25
6
400
1
55
75
0.176
230
15.57
120
0.710
0.735
0.522
0.708
0.633
0.654
0.511
0.632
0.607
15
7
400
2
85
100
0.185
238
11.39
150
0.686
0.853
0.701
0.607
0.614
0.773
0.626
0.560
0.643
8
8
400
3
115
125
0.238
227
7.3
190
0.554
0.691
0.876
0.471
0.529
0.618
0.802
0.486
0.609
14
9
400
4
145
25
0.460
228
10.49
255
0.000
0.706
0.740
0.251
0.333
0.630
0.658
0.400
0.505
22
10
400
5
25
50
0.397
220
10.56
205
0.158
0.588
0.737
0.420
0.373
0.548
0.655
0.463
0.510
21
11
600
1
85
125
0.270
219
4.96
95
0.475
0.574
0.976
0.793
0.488
0.540
0.955
0.707
0.672
4
12
600
2
115
25
0.312
240
8.57
170
0.369
0.882
0.822
0.539
0.442
0.810
0.737
0.520
0.627
11
13
600
3
145
50
0.248
244
4.51
296
0.530
0.941
0.996
0.112
0.516
0.895
0.992
0.360
0.691
1
14
600
4
25
75
0.238
239
9.75
175
0.555
0.868
0.771
0.522
0.529
0.791
0.686
0.511
0.629
9
15
600
5
55
100
0.219
241
7.1
214
0.602
0.897
0.885
0.390
0.556
0.829
0.813
0.450
0.662
6
16
800
1
115
50
0.243
235
4.41
220
0.542
0.809
1.000
0.369
0.522
0.723
1.000
0.442
0.672
5
17
800
2
145
75
0.159
242
6.66
285
0.752
0.912
0.904
0.149
0.668
0.850
0.838
0.370
0.682
3
18
800
3
25
100
0.185
235
17.04
110
0.686
0.809
0.459
0.742
0.614
0.723
0.480
0.660
0.619
12
19
800
4
55
125
0.206
235
14.35
105
0.635
0.809
0.574
0.759
0.578
0.723
0.540
0.675
0.629
10
20
800
5
85
25
0.303
245
12.16
190
0.392
0.956
0.668
0.471
0.451
0.919
0.601
0.486
0.614
13
21
1000
1
145
100
0.270
221
7.74
329
0.474
0.603
0.857
0.000
0.488
0.557
0.778
0.333
0.539
18
22
1000
2
25
125
0.296
200
25.82
112
0.409
0.294
0.083
0.736
0.458
0.415
0.353
0.654
0.470
24
23
1000
3
55
25
0.270
232
22.55
138
0.475
0.765
0.223
0.647
0.488
0.680
0.392
0.586
0.536
19
24
1000
4
85
50
0.136
248
16.89
228
0.808
1.000
0.466
0.342
0.722
1.000
0.483
0.432
0.659
7
25
1000
5
115
75
0.059
245
16.93
320
1.000
0.956
0.464
0.031
1.000
0.919
0.483
0.340
0.685
2
Figure 12. shows the response surface optimization plot for optimum burnishing parameters and output responses. It is illustrated in this figure that the optimized average surface roughness Ra is 0.195 m, surface microhardness is 247.596 HV, surface out of roundness OR is 6.178 m and change on diameter D is 155.49 m with desirability factors of 0.659, 0.994, 0.924 and 0.588, respectively. The optimized values of the roller burnishing process are burnishing speed of 620.202 rpm, number of passes of 3, depth of penetration of 71.6 m, and burnishing feed of 107.828 m/rev
Figure 12. Response surface optimization plot for optimum burnishing parameters and output responses.
C.Artificial Neural Network
– Single Optimization Results
Any multilayer Artificial Neural Network model consists of input layer, hidden layer, and output layers. In the present investigation, the ANN architecture containing an input layer with 4 neurons as the first layer at network where each neuron represented one input parameter. A hidden layer varying the number of neurons and an output layer with 1 neuron having purelin processing function was employed in the current study. The model was trained utilizing 17 experimental train set. During training, 4 experiments were utilized to test the ANN model. and four experiments were utilized for validating the ANN model. MATLAB version R2015a (8.5.) was used in writing the source code.
The input layer contains four neurons represented the control parameters as number of passes, burnishing speed, depth of penetration and burnishing feed. The output layer contains 1 neuron which is having purelin processing function representing the output response. For achieving the most excellent structure for a given ANN with regard to number of neurons in the hidden (the second layer at the network used in this investigation) layer, a training technique simulations models with numbers of hidden neurons in the range from 5 to 50 was carried out. The comparison of different training algorithms and variations of neurons is determined for all the networks 4-5-1, 4-10-1, 4-20-1, 4-30-1, 4-40-1 and 4-
50-1. The best network is 4-50-1 for average surface roughness (Ra), 4-50-1 for surface microhardness (HV), 4-30-1 for surface out of roundness (OR), and 4-40-1 for change in diameter (D). The Performance curve and regression graphs for optimum model of average surface roughness are presented in Figure 13.
training, validation and test phases and its values were 0.9979, 1, 0.9996; respectively. As a result, there is a close fit between experimental and predicted results. Figure 15. determine the generated regression plots for the testing.
4 4
5-50 4
Figure 13. The performance curve and regression graphs for optimum model of average surface roughness
– Multi Optimization Results
The architecture proposed to simultaneously predict average surface roughness, surface microhardness, surface out of roundness and change in diameters determined by 25 experimental trials using burnishing speed, number of burnishing passes, depth of penetration and the burnishing feed prior to roller burnishing as input. Figure 14 illustrates the architecture of the ANN with three layers (first layer is the input layer, second layer is one hidden layers and the last one is the output layer), a multilayer feed forward network with sigmoid activation function for the hidden and purelin for output layers. The optimum number of neurons that produce minimum mean square error found as best network architecture. The ANN architectures 4-40-4 was found optimum in this work. The correlation coefficient was calculated for the
Figure 14. ANN architecture used to predict average surface roughness, surface microhardness, surface out of roundness and change in diameter after roller burnishing
Table 15. Performance curve and regression graphs for multiple optimum models of all responses.
-
-
A COMPARISON BETWEEN THE OUTPUT RESPONSES VALUES
Figure 16 shows a comparison between the experimentally obtained output responses values and
the results from the multiple optimum ANN model (during training, validation and test phases).
Figure 16. Output responses values obtained experimentally and by the multiple optimum ANN for Al-2011 alloy.
-
DISCUSSION
This research's major goal is to investigate in depth the effect of different burnishing parameters (speed, number of passes, depth of penetration, and feed), on some burnishing characteristics (surface roughness, microhardness, change in diameter, and surface out of roundness).
The results are presented in graphs using the final mathematical models that created in the previous section throughout the response surface methodology.
-
Effect of Roller Burnishing Parameters on Average Surface Roughness
The influences of burnishing speeds on arithmetic overage (Ra) of surface roughness at different number of passes, depth of penetrations and feeds are shown in Figure 17. The effect of burnishing speed on surface roughness is not the same as shown in these figures. Figure 17.a represents the relation between burnishing speed and surface roughness for different number of passes. An interaction among burnishing speed and number of passes can be seen in this figure.
(a)
(b)
(c)
Figure 17. The influences of burnishing speeds on arithmetic overage (Ra) of surface roughness at different number of passes, depth of penetrations and feeds
Surface finish was significantly improved by the combination of very low speeds and low number of passes. Therefore, Low speeds are preferable since the burnishing tool's deforming action is larger and metal flow is more regular. A combination of very high speeds with high number of passes improves surface finish. Also, when the high speeds combined with the low number of passes gives a deterioration
in surface finish. because of chatters which usually occurs at this condition. Also, a combination of low speed with high number of passes deteriorates the surface finish. High number of passes deteriorates the surface finish because the overhardening as a result of the many repetitions burnishing process.
-
Effect of Roller Burnishing Parameters on Average Surface Microhardness
Figure 18. shows the variation of surface microhardness as a function of burnishing speed at various number of passes, depth of penetrations and feeds for A-7075 alloys. It can be generally observed from these Figures that the trend of results in both cases are the same, at any value of number of passes,
(a)
(b)
(c)
Figure 18. The influences of burnishing speeds on surface microhardness (HV) at different number of passes, depth of penetrations and feeds
depth of penetration and feed, the increasing in burnishing speed up to the middle value (600 rpm) lead to an increase in surface microhardness, whereas the surface microhardness starts to decrease gradually with more increasing in burnishing speed. This is partially because At high speeds, the burnishing tool's deforming action is limited, and partially because of the chatter that occurs at high speeds. [8].
-
Effect of Roller Burnishing Parameters on Out of Roundness
The influence of burnishing speed on surface out of roundness for AL- 7075 at different values of number of passes, depth of penetration, and feed rate are shown in Figure
19. Generally these figures show that the surface out of roundness reduces to a minimum value when the burnishing speed increases. Then, surface out of roundness gradually increases with more increasing in burnishing speed.
(a)
(b)
(c)
Figure 19. The influences of burnishing speeds on surface out of roundness (OR) at different number of passes, depth of pnetrations and feeds
-
Effect of Roller Burnishing Parameters on Worlpiece Diameter Change
The influence of the burnishing speed on the change in the diameter of the workpiece at different number of passes, depth of penetration and burnishing feed can be assessed from Figure 20. An interaction occurred between the curves of the effects of speed and number of passes on change in diameter as shown in Figure 20.a. Its also illustrates that the increasing in burnishing speed leads to an increase in the change in the diameter of the workpiece at low number of passes. Additionally, the increasing in burnishing speed at high number of passes leads to gradually decrease in change in diameter of the workpiece.
(a)
(b)
(c)
Figure 20. The influences of burnishing speeds on the change in workpiece diameter (D) at different number of passes, depth of penetrations and feeds
In general, it can be noticed that for different depth of penetration and burnishing feed utlized in this investigation that as the burnishing speed is increased, the change in workpiece diameter reduces until it reaches to lowest value, further with the increase in burnishing speed, the change in the diameter of the workpiece increases. The increasing in the change in workpiece diameter at high speed may be due the deterioration in surface layers which occurs because of the presence of chatter.
-
-
CONCLUSION
From the results and discussion presented earlier the following conclusion are drawn
-
This study presents a development of single and multi- optimizations for obtaining the best combinations of roller burnishing parameters that leads to good results of different responses through three methodologies (Taguchi method, ANN, and RSM). The analysis show that ANN is the most accurate methodology for obtaining the best combinations of roller burnishing parameters that lead to good results of different responses. Although ANN model provided better prediction accuracy than Taguchi and RSM, both methods showed relatively high prediction accuracy and can be used for the same purpose.
-
The results obtained have shown that the best network is 4-50-1 for average surface roughness (Ra), 4-50-1 for surface microhardness (HV), 4-30-1 for surface out of roundness (OR), and 4-40-1 for change in diameter (D).
-
The optimum number of neurons that produces minimum mean square error found as the best network architecture. In the case of multi-response optimization, the ANN architectures 4-40-4 was found as the best networks in this investigation.
-
GRA in the Taguchi technique for multi-response problem optimization is a very valuable tool for predicting various burnishing surface characteristics. The optimum combination of parameters for better combination of surface roughness, microhardness, change in diameter, and surface out of roundness are burnishing speed of 600 rpm, number of passes of 3, depth of penetration of 145 µm and burnishing feed of 50 µm/rev because of highest grey relational grade.
-
RSM enables the quadratic model to be fitted to a variety of responses. The optimized average surface roughness is
0.195 m, surface microhardness is 247.596 HV, surface out of roundness is 6.178 m and change on diameter is
155.49 m with desirability factors of 0.7728. The optimized values of the roller burnishing process are burnishing speed of 620.202 rpm, number of passes of 3, depth of penetration of 71.6 µm, and burnishing feed of 107.828 µm/rev.
The presented results are useful for production and design engineers to allocate and select the roller burnishing
conditions to produce a surface with high performance characteristics.
REFERENCES
-
Sergio Rinaldia, Giovanna Rotellab, Domenico Umbrelloa, Experimental and numerical analysis of roller burnishing of Waspaloy
, Procedia Manufacturing, Vol.34, pp. 6572, 2019.
-
Partchapol Sartkulvanich, Taylan Altan, Francisco Jasso, Ciro Rodriguez, Finite Element Modeling of Hard Roller Burnishing: An Analysis on the Effects of Process Parameters Upon Surface Finish and Residual Stresses, Journal of Manufacturing Science and Engineering, Vol.129, pp. 705-716, 2007.
-
Xiaoliang Liang, Zhanqiang Liu, Bing Wang, State-of-the-art of surface integrity induced by tool wear effects in machining process of titanium and nickel alloys: A review, Measurements, Vol. 132, pp. 150- 181, 2019.
-
Trung-Thanh Nguyen, Xuan-Ba Le, Optimization of roller burnishing process using Kriging model to improve surface properties, Journal of Engineering Manufacture, Vol. 233(12), pp. 22642282, 2020.
-
C. S. Jawalkar, Development and Analysis of Sustainable and Innovative Surface Finishing Process Through Combined Effects of Ball and Roller Burnishing, ICEM 2020, pp. 175-187, 2020
-
Mehrzad Boozarpoor, Majid Elyasi, Morteza Hosseinzadeh, An Investigation of The Surface Quality of Burnished AISI 4340 Steel, Journal of Engineering Manufacture, Vol. 232, issue 3, pp. 299-313, 2018.
-
Trung-Thanh Nguyen, Minh-Thai Le, Optimization of the Internal Roller Burnishing Process for Energy Reduction and Surface Properties, Journal of Mechanical Engineering, Vol. 67, issue 4, pp. 167-179, 2021.
-
M.H. El-Axir, An Investigation into The Ball Burnishing of Aluminum Alloy 6061-T6, J. Engineering Manufacture, Vol. 221, pp. 1733-1741, 2007.
-
Yungchang Yen, Partchapol Sartkulvanich, Taylan Altan, Finite element modeling of roller burnishing process, CIRP Annals 54, Vol. 54, Issue 1, pp. 237-240, 2005.
-
Giovanna Rotella, Serafino Caruso, Antonio Del Prete, Luigino Filice, Prediction of surface integrity parameters in roller burnishing of Ti6Al4V, Metals, Vol.10(1671), pp. 117, 2020.
-
Deepak Mahajan, Ravindra Tajane, A Review on Ball Burnishing Process, International Journal of Scientific and Research Publications,
Volume 3, Issue 4, pp. 1-8, 2013
-
Prabhu, P. R., S. M. Kulkarni, and S. S. Sharma, Influence of deep cold rolling and low plasticity burnishing on surface hardness and surface roughness of AISI 4140 steel, World Academy of Science, Engineering and Technology, Vol.48, pp. 619-624, 2010.
-
Ch.Priyadarsinia, V.S.N. Venkata Ramanab, K.Aruna Prabhac, S.Swethad, A Review on Ball, Roller, Low Plasticity Burnishing Process, Materials Today: Proceedings, Vol. 18, pp. 5087-5099, 2019.
-
B. Sachin, S. Narendranath, D. Chakradhar, Effect of working parameters on the surface integrity in cryogenic diamond burnishing of 17-4 PH stainless steel with a novel diamond burnishing tool, Journal of Manufacturing Processes, vol.38, pp.564-571, 2019.
-
M. Fattouh, M. H. El-Axir, S. M. Serage, Investigations Into The Burnishing Of External Cylindrical Surfaces Of 70/30 Cu-Zn Alloy, Wear, Vol. 127, pp. 123 -131, 1988.
-
Vijay Kurkute, Sandeep T. Chavan, Modeling and Optimization of Surface Roughness And Microhardness for Roller Burnishing Process Using Response Surface Methodology for Aluminum 63400 Alloy, Procedia Manufacturing, vol.20, pp. 542-547, 2018.
-
Sandeep Kumar, Bedasruti Mitra, Naresh Kumar, Application of GRA Method for Multi-Objective Optimization of Roller Burnishing Process Parameters Using A Carbide Tool on High Carbon Steel (AISI-1040), Grey Systems: Theory and Application, Vol. 9, Iss. 4, pp. 449-463, 2019.
-
Reza Teimouri, Saeid Amini, A comprehensive optimization of ultrasonic burnishing process regarding energy efficiency and workpiece quality, Surface and Coatings Technology, Vol. 375, pp. 229-242, 2019.
-
Trung-Thanh Nguyen, Le-Hai Cao, Xuan-Phuong Dang, Truong-An Nguyen, Quang-Hung Trinh, Multi-objective optimization of the flat burnishing process for energy efficiency and surface characteristics, Materials and Manufacturing Processes, vol. 34, issue 16, pp. 1888- 1901, 209.
-
M.H. El-Axir, A.A. Ibrahim, Some Surface Characteristics Due to Center Rest Ball Burnishing, Journal of Materials Processing Technology, Vol. 167, pp. 47-53, 2005.
-
M.R. Stalin John, Welsoon Wilson, Prasad Bhardwaj, Avinav Abraham, B.K.Vinayagam, An Investigation of Ball Burnishing Process on CNC Lathe Using Finite Element Analysis, Simulation Modelling Practice And Theory, Vol.62, pp. 88101, 2016.
-
M.H. El-Axir, O.M. Othman, A.M. Abodiena, Improvements in Out-of- Roundness and Micro Hardness of Inner Surfaces by Internal Ball Burnishing Process, Journal of materials processing technology, Vol. 196, pp. 120-128, 2008.
-
Naresh Kumar, Sachdeva Anish, Lakh winder pal Singh and Himanshu Tripathi, Experimental Investigation of Effect of Roller Burnishing Process Parameters on Surface Roughness and Surface Hardness of C40E Steel, Int. J. Machining and Machinability of Materials, Vol. 18, pp. 185-199, 2016.
-
J.R. Davis, Aluminum and aluminum alloys, ASM Specialty handbook, Menlo Park, ASM International, pp. 351-416, 1996.