
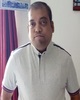
- Open Access
- Authors : Vivek Singh
- Paper ID : IJERTV11IS050153
- Volume & Issue : Volume 11, Issue 05 (May 2022)
- Published (First Online): 19-05-2022
- ISSN (Online) : 2278-0181
- Publisher Name : IJERT
- License:
This work is licensed under a Creative Commons Attribution 4.0 International License
Statistical Process Control (SPC) Guide Line
Vivek Singp
1Manager Quality Assurance Ahmedabad
Abstract – Statistical process control (SPC) is a method of quality control which employs statistical methods to monitor and control a process. This paper helps us to ensure that the process operates efficiently, producing more specification-conforming products with less waste. SPC can be applied to any process where the "conforming product" output can be measured. Key tools used in SPC include run charts, control charts, a focus on continuous improvement. An example of a process where SPC is applied is in manufacturing lines.
Key Words: Statistical process control, control charts, Methodology, rules
1.INTRODUCTION
SPC has been around since the early 1920s. It is involves the study of variation, which simply means difference. Variation is referred to as either common cause, which is inherent variation (often called noise), or special cause, meaning theres an assignable reason for its occurrence. Special cause variation results from circumstances that are not always acting on the process, but when they are, instability will affect the output until they are identified and addressed. The purpose of SPC is to cost effectively ensure that products produced meet the customers expectations. From a system standpoint, it defines the extent of common cause variation and also signals when a special cause has acted on it so that its source can be identified and reacted to before the problems arise.
-
Pre-Requisites
There are three key necessities for the application of SPC: measurement system robustness; a sample data collection scheme; and the ability to react to special cause instability, if encountered, in real time. Each will be covered at an introductory level below. More detailed information can be found in the SPC.
-
Measurement System Robustness
A critical issue in preparing to implement SPC is that no measurement system is without variation. The broad topic is referred to as Measurement System Analysis Measurement system robustness is defined as the systems ability to minimize variation from measurement practices, therefore providing a consistent means of acquiring accurate and precise data for the feature to be checked. In other words, by using the best-suited device, providing details and documented instructions and thoroughly training all those who will collect data.
2. ABBREVIATIONS AND ACRONYMS SPC Statistical Process Control ,CMMs Co-ordinate Measuring Machine, 5M&M Man, Machine, Material,
Method, Movement, Environment, SPM Statistical Process Monitoring, LCL Lower Control Limits, UCL Upper Control Limits, CMM Capability Maturity Model, CMMI Capability
Maturity Model Integration, HML Hypertext Mark-up Language, HCM Hardware Compatibility List.
-
The most common control chart types for variable and attribute data.
There are different kinds of control charts for variable and attribute data. That said, they generally share a common look and features:
-
Each studies a specific characteristic of a part or process (vertical axis) overt time (horizontal axis).
-
Data points are plotted in a graphical framework.
-
Analysis is conducted with respect to an average line and control limits (not specification limits).
Chart -1: Control Charts
Fig -1: Control Charts Characteristics
Control limits are the boundaries of a zone in which almost all the data should fall in order for the process to be in a controlled state. The key point for control charts is the ability to separate special (assignable) cause variation from common (inherent) cause variation, allowing for correction before the production of non-conforming product.
4. TYPES OF CHARTS
There are two types of data are commonly use in SPC.
1) Variable Data
2) Attribute Data Variable Data Charts:- X-Bar and R-Bar Chart
When it comes to variable data, the overwhelmingly preferred control chart type is the x (read as X-bar) and R. (An example is shown below.)
These are often referred to as average & range (or Shewhart) charts. They are favored because:
-
Calculations are relatively easy.
-
Underlying distribution of individuals need not be normal. They are less susceptible to type 1 errors (over adjustment/adjusting when not necessary).
-
Why? Because the averages of the average tend toward normality.
Fig -2: X-Bar and R-Bar Chart
Individuals and Moving Range (I & MR) Charts
Another popular variable data control chart type is the individuals and moving range chart (I and MR). (An example is shown following.)
On the plus side
-
Calculations are very easy with a subgroup size of 1 (none at all on the Individuals chart, and only subtraction of two numbers on the MR charts).
-
If offers a way to statistically control a process where samples are scarce or costly to evaluate, or samples are produced in homogeneous batches where repeated sampling within the batch would not vary (e.g., chemicals etc.)
On the minus side
-
With a sample size of 1, there is increased susceptibility to type 1 errors because, unless the underlaying data are somewhat normally distributed, the Individuals Chart will give more false signals for certain tests of stability (indicate that the process is out of control when it actually is not), and
Because of its subgroups size 1, I and MR control charts are not sensitive to shifts in the means of the process as X-bar and R charts. Generally speaking, the larger the subgroup size, the more sensitive it is to those types of changes.
Fig -3: I and MR Chart
Attribute Data Charts :-
Control charting for attribute data is similar in format to that for variable data; however, there are some structural differences:
-
Remember, attribute data is counting (or discrete) data.
-
Unlike control charting for variable data, there is no R chart for attribute data.
-
Also, there is not one prevalent chart type for attribute data as there is for variable data (X-bar & R Charts).
-
Because attribute data are less powerful than variable data, attribute data require much larger sample size to reap similar benefits.
-
The diagram below shows the four commonly used chart types for attribute data. The proper one to apply in a particular circumstance is based on answering two questions:
-
Are subgroup sizes constant?
-
Is the characteristic being studied described as a defect or a defective?
Defects |
Defectives |
|
Variable Sample Size |
U – CHART |
P – CHART |
Constant Sample Size |
C – CHART |
NP – CHART |
Control Charts for Defectives : P and NP
P and NP charts show how the number of defective units changes over time. Examples are paint aberrations on a completed vehicle and fabric irregularities in a bolt of cloth. These two charts are essentially the same, except that a P chart does not require a constant sample size while an NP chart does.
Fig -4: P Chart
Fig -5: NP Chart
Control Charts for Defectives : C and U
U and C charts show how the number of defects per unit(s) changes over time. Defects can be described as any characteristic that is present but should not be, or nt present but should be. For example, a scratch, contaminant, missing item, and extra item are all defects.
These two charts are essentially the same, except that a U chart does not require a constant sample size per subgroup, whereas the C Chart does
Fig -6: U Chart
Fig -6: C Chart
Rules of abnormal condition of SPC :-
Rule 1:- One point is more than 3 standard deviations from the mean. One point is outside the control limits as shown in fig 7.
Fig -7: SPC Chart for Rule 1
Rule 2:- Nine (or more) points in a row are on the same side of the mean. This represents sudden, large shifts from the average. These are often fleeting a one-time occurrence of a special cause like the flat tire when driving to work as shown in fig 8.
Fig -8: SPC Chart for Rule 2
Rule 3:- Six (or more) points in a row are continually increasing (or decreasing) trend exist either moving to upper limit or lower limit as shown in fig 9
Fig -9: SPC Chart for Rule 3
Rule 4:- Fourteen (or more) points in a row alternate in direction, increasing then decreasing. They represent smaller shifts that are maintained over time. A change in raw material could cause these smaller shifts as shown in fig 10.
Fig -10: SPC Chart for Rule 4
Rule 5:- Two (or three) out of three points in a row are more than 2 standard deviations from the mean in the same direction. This represents a process that is trending in one direction. For example, tool wearing could cause this type of trend. This is shown in fig 11.
Fig -11: SPC Chart for Rule 5
Rule 6:- Four (or five) out of five points in a row are more than1 standard deviation from the mean in the same direction. It occurs when you have more than one process present and are sampling each process by itself. This is shown in fig 12
Fig -12: SPC Chart for Rule 6
Rule 7:- Fifteen points in a row are all within 1 standard deviation of the mean on either side of the mean. Rule 7(stratification) also occurs when you have multiple processes, but you are including all the processes in a subgroup. This can lead to the data hugging the average. This is shown in fig 13.
Fig -13: SPC Chart for Rule 7
Rule 8:- Eight points in a row exist with none within 1 standard deviation of the mean and the points are in both directions from the mean. Rule 8 (over-control) is often due to over adjustment as shown in fig 14.
Fig -14: SPC Chart for Rule 8
Application of SPC:- SPC is used in manufacturing industries to understand the process and the specification limits. Eliminate special cause of variation, so that the process is stable. Monitor the on-going production process, assisted using control charts, to detect significant changes of mean or variation.
Steps of SPC:- There are 07 steps to set up Statistical Process Control (SPC) on Production process. This is shown in Fig 15.
Fig -15: & Steps of SPC
-
Select critical- to- quality (CTQ) products characteristics:- For example, if metal thickness is lower than specification then it might not perform it task. Thickness is CTQ characteristics.
-
Select critical processes: – What qualifies a process as critical? If something goes wrong with that process, it will probably have a sizable impact on at least one CTQ characteristic.
Critical processes are often indicated with a * on the control plan.
-
Determine if machines can calculate SPC by themselves: – Many modern pieces of equipment collect and analyze data, and then issue an alert when they get out of control. However, this is very uncommon in industry, so lets assume this is not the case; it means you will need to carry out steps 4 and 5
-
Gather data and process knowledge of what impacts the output of the process: – For example, the variables that might affect the output of a gluing process are listed below:
-
Process input: glue
-
Process settings: cycle time; fixture
-
Environment: outside temperature, humidity, etc.
As long as the Cpk index is within the control limits, engineers and production leaders are encouraged to test different approaches. A Cpk target of 1.0 is often attainable within a few weeks. 1.33 is more challenging. 1.66 is much, much harder!
If necessary, you can use another statistical technique called Design of Experiments (DoE) to help you get close to the optimal values for variables that impact your process output. But, be prepared there are several available approaches here, and it can get relatively complex.
7) Keep it up in the long term: – You manage to reduce variation. Set a new target and keep controlling the key variables. If your process characteristics drift in one way or another, your statistical process control system will alert you. Otherwise, dont tamper with the process, constant little adjustments will mechanically make the process unstable in statistical terms.
Table Head |
Table Column Head |
||
Table column subhead |
Figs no |
||
EASE OF USE |
Control charts |
Interpreting control chart |
Fig 1 |
EASE OF USE |
Control charts |
X-Bar and R-Bar |
Fig 2 |
EASE OF USE |
Control charts |
I and MR Chart |
Fig 3 |
EASE OF USE |
Control charts |
P Chart |
Fig 4 |
EASE OF USE |
Control charts |
NP Chart |
Fig 5 |
EASE OF USE |
Control charts |
U and C Chart |
Fig 6 |
Problem Definition |
SPC Chart for Rule 1 |
Rules to be followed |
Fig 7 |
Problem Definition |
SPC Chart for Rule 2 |
Rules to be followed |
Fig 8 |
Problem Definition |
SPC Chart for Rule 3 |
Rules to be followed |
Fig 9 |
Problem Definition |
SPC Chart for Rule 4 |
Rules to be followed |
Fig 10 |
Problem Definition |
SPC Chart for Rule 5 |
Rules to be followed |
Fig 11 |
Problem Definition |
SPC Chart for Rule 6 |
Rules to be followed |
Fig 12 |
Problem Definition |
SPC Chart for Rule 7 |
Rules to be followed |
Fig 13 |
Problem Definition |
SPC Chart for Rule 8 |
Rules to be followed |
Fig 14 |
Methodology |
Steps In Statistical Process Control |
Steps in SPC |
Fig 15 |
Based on this, you might conclude that the viscosity of the glue and the ambient humidity are two variables that need controlling.
5) Control independent variables that have a sizeable impact on the process output: – There are 2 ways you can ensure that your production does not deviate too much:
-
Gather data on the variables you identified previously on a regular basis, e.g., 5 random samples every 4 hours.
-
Teach the production operators and leaders necessary calculations and how to plot them on a chart, e.g., on the X bar R chart, the most popular tool. For simplicity, we tend to set a goal for the process capability index (Cpk) and let operators plot the evolution of that index over time.
6) Look for ways to reduce variation: – Now that you have made variations visible, the next step is to find ways to reduce it. If you use statistical process control charts,ideally it looks somewhat like this:
Figs and Tables: –
3. CONCLUSIONS
After verification of Statistical Process Control, following points are observed.
Control Charts that help monitor manufacturing processes can be more helpful digitally as they can be presented by a well- designed system.
Data for monitoring and visualization is better through applications as all data and visualization for it is accessible easily.
Customization to system and any addition to Statistical Process Control is easier as systems can be reprogrammed to add functionalities necessary, without excessive usage and analysis training
Provision of other charts that could be useful such as normality charts and mean charts.
REFERENCES
[1] Barlow, R. E. & Irony, T. Z. (1992) "Foundations of statistical quality control" in Ghosh, M. &Pathak, P.K. (eds.) Current Issues in Statistical Inference: Essays in Honor of D. Basu, Hayward, CA: Institute of Mathematical Statistics, 99-112. [2] Salacinski, T (2015) SPC – Statistical Process Control. The Warsaw University of Technology Publishing House. [3] S. Jagannatha, M. Niranjanamurthy, and P. Dayananda, Algorithm Approach: Modelling and Performance Analysis of Software System , Journal of Computational and Theoretical Nanoscience (American Scientific publishers),December 2018,Volume 15, Issue 15, PP. 33893397
[4] AIAG Manual- Fourth Revision.BIOGRAPHIES
Myself Vivek Singh, I have complete B.Tech in 2004 in mechanical engineering. I have total 17+ years industry experience in Quality Assurance. I am lean six sigma black belt certified. |