
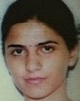
- Open Access
- Authors : Gurpreet Kaur
- Paper ID : IJERTV13IS010085
- Volume & Issue : Volume 13, Issue 01 (January 2024)
- Published (First Online): 02-02-2024
- ISSN (Online) : 2278-0181
- Publisher Name : IJERT
- License:
This work is licensed under a Creative Commons Attribution 4.0 International License
The Diagnostic Imaging Techniques in Medical Field
Published by : http://www.ijert.org
International Journal of Engineering Research & Technology (IJERT)
ISSN: 2278-0181
Volume 13, Issue 01 January 2024
Author Gurpreet Kaur
Department of Computer Science, SGGS College, affiliated to Punjab University, Chandigarh, India.
Abstract: The medical study is predominantly being governed by the advancements in imaging technologies. Superior diagnosis, treatment proposals, treatment monitoring and assistive procedures easily govern the medic to finer cure, refined therapies and better patient outcomes. Medical imaging continues to evolve providing a more promising diagnostic mechanism. This visual representation of the organs can differentiate the normal from the abnormal. The medical imaging techniques can primarily be categorized to study both the physiology and anatomy of the diseased. There are various imaging technologies as x- ray, computed tomography, Magnetic Resonance Imaging (MRI) etc. These advancements have many applications in diagnosis. Image fusion collaborates the images from multiple modalities to include details from all. This paper focuses to collaborate the study of the advancements in multiple medical modalities and how these innovations promise to improve patient outcomes.
Keywords : Medical Imaging, Anatomy, Physiology, Multimodal, Image fusion.
-
INTRODUCTION
Medical imaging is the field of medicine also known as radiology. It is a non invasive technique to capture the underlying organ, bone, tissue and blood vessels in order to assess, diagnose and cure the patient. It plays a major role in modern imaging to show the interior of the body for analysis and treatment. The progression in imaging technology, revolutionaries all healthcare. The improved technologies provide high quality images with no interventions and reduced radiation. These modalities help accurately differentiate between the healthy and the diseased tissue. The innovation and accomplishment in medical image fusion research has enhanced due to the advancements in medical imaging equipments. The medical images are confined from different modalities as from anatomical, functional, tomography and projection. Each medical imaging modality aims to deliberate and extract the precise and detailed clinical features from the domain. With limited convincing matter from one medical image, it is difficult to reveal structural tissue differences [1]. Image fusion is a technique to combine various acquired medical images from more than one modality to attain additional clinical content for diagnosis. Medical imaging has become an inherent part of diagnostic mechanism. In dealing with medical images, complexities and computations in image processing increase as large volumes of unprocessed data is produced [2]. Image Fusion is a mechanism to reduce this data bulk and also reveal additional information from the fused image which was lacking in original inputs. The advent and progression in imaging techniques is radically controlling treatment planning. Image fusion is spreading itself in other application areas.
The sections are divided as Section II details the Medical Image Processing, section III list the Modality aspects in Image Fusion, Section IV includes discussion with respect to Techniques used and Modality selected , section V gives the Conclusion of the Paper..
-
MEDICAL IMAGE PROCESSING
-
Medical Imaging:
These provides visual representation of the image. It forms the center of modern medicine. With the x-rays radiation medicine came into form [3]. Since various machines have been produces and modalities devised. Ionizing radiation can lead to mutation and cancers. Nuclear resonance imaging possess less risk. Ultrasonic are the safest amongst the medical imaging modalities. Medical imaging make possible image led surgeries. Surgeries, tumor localizations, blood clots, blockage, stent placements are all when governed by image processing, better captivate the procedure. Medical images perform accuracy in diagnosis, this is limited to the acquisition of high quality images which seems a vital requirement [4]. Clinical visibility is achievable from multiple modalities and methods [5]. For enhanced image analysis deep learning and artificial intelligence provide deeper understanding [6].
-
Medical Image Fusion Applications:
Image fusion applications are dominating various medical domains with the aid of images and videos. Fusing images reduces redundancy by assimilating information from multiple source image inputs. Image fusion in medical domain captures human body images confining the organs, tissues, physiological functions and cellular compositions. It provides disease analysis with increased accuracy supporting better decision making. The original images are noisy. Blurring and inconsistencies due to incomplete image capture or limitations of the acquiring devises are overcome due to image fusion. Image fusion is making its presence in image processing [7], Computer assisted diagnoses [8], dosage measurement, and identification of lesions. Benign, pre-malignant, and malignant tumors localization. It plays a vital role in extracting physiological data from anatomical structures[9]. Radiotherapy guidance in treatments, scheduling and delineating, Imaging helps capture structures, bone diversities, tissue thickness, shape and location. These areas are further expanding with the preface of new acquisition procedures[10] Image fusion is expanding to direct superior diagnosis, playing a vital role in medical analysis, where image inconsistencies can have serious consequences.
C. Medical Diagnostic Approach:
Medical images are used for diagnostic analysis. Fractures, tumors, structures, bones are captured using images, various angles and directions help in superior physiology analysis. Imaging promotes immediate diagnosis and subsequent treatment. During treatment track of planning and recovery monitoring is accomplished using imaging modalities. The stage of disease can be devised. Images acquired from CT, MRI, PET, SPECT can detect the meta-stages and provide progression to base it channelized treatment planning. This incite helps in understanding the lesion growth , the malignant organs and direct surgical decisions. Once the treatment is chalked, its response varies with every case. Monitoring and assessment is studied to estimate the orbital reactions and fixations.
C. Medical Fusion Categories
(a) |
(b) |
(c) |
To formulate and incorporate all complementary data, fusion is categorized into different types [11]-[13] as depicted in Fig 1.
(d) |
Fig . (a) Fusion Categories (a) Multiview (b) Multi Temporal (c) Multi focus (d) Multimodal fusion
-
Multi-view fusion
Images are acquired from different viewpoints. This capture from multi dimensional angle these include pixel wise weighted average, object level fusion.
-
Multi-view fusion
Multi-temporal fusion: These images are governed by time. the same organ under study is captured at different time intervals, indicative of the progression These multiple images, acquired at different times, reinforce the pragmatic concepts and perceive alterations.
-
Multi-focus fusion
The source images acquired by varied focal length, when fused expand the details. It involves compression in the input images. The focus depths of the inputs are different which fuse to preserve additional information.
-
Multi-modal fusion
Sensors of different acquisition models capture the image sets. Due to the principle complementary details are captured in different input sets. When fused the multimodal images acquire additional feature information.
-
MODALITY ASPECTS IN IMAGE FUSION
There are various imaging modalities playing a crucial role in medical domain. modularity has a relevance in clinical domain and required medical expertise. These work on different physical principals [14-17]. Image fusion helps preside over the nature of modalities [18]. Modalities are functional metabolic modality and anatomical morphological modalities [1]. The anatomical modalities as an external source in Magnetic Resonance Imaging (MRI), Computed Tomography (CT) give the position, structure and relations. Functional modalities emit from within the body as in Ultrasound (US), Positron Emission Tomography (PET), Single Photon Emission Computed Tomography (SPECT) provide tissue illustrations in pseudo color [19].
Mammography and x-ray produce images to evaluate breast cancer. Hand held transducer in Ultrasound transmits and receives acoustic waves. CT provides several slices of the
underlying tissue. These are captured in various directions. MRI analyze features non invasively in a radiation free manner. PET display abnormal metabolic features with malignancy.
-
DISCUSSION
The organ , tissue, modalities and processing formulate the fusion mechanism with certain limitations and future aspects put forth.
-
Technique Based Analysis
Various models and in medical image fusion are existing. Pixel level and transform domain fusion techniques are used for fusion. Pixel level the information remains intact while transform domain the salient features are better depicted [20]. There are various techniques defined in literature for fusion, . The color space techniques, multiscale transforms, pyramids and advanced wavelets. Color space models encompass physiological details. In multiscale the images are decomposes in various scales where information may coexist at different levels [21]. The pyramid transforms lack directional information and structures are not well represented[22]. The wavelets, have missing edges and segment information [23]. Complex wavelets as Curvelet, Contourlet and NSCT. NSCT comprises of two components, the multiscale non subsampled pyramid transform and non subsampled directional filter bank. Fourier transforms do not undergo the problems of wavelets or complex transforms. The fractional wavelets have global localized transform in time-fractional-frequency domain.
The literature has moved from single transform to hybrid techniques to exploit variation amongst modalities. In addition to the technique to implement image fusion, there are various other factors affecting fusion results [24].
-
Modality Based Analysis
The fusion of images is governed by medical organs and modalities. The medical images are obtained from one or more modalities [24]. The modality captures underlying structures n anatomy. Fusion of same modality images work under controlled environments and limited complexity. image fusion captures and reveal the spectral content. But during the process, spatial information is misrepresented with loss of spatial features.
Fusion of multiple modality images enhances fusion results but complexity structures increase due to different principle, varied acquisition mechanism and interdependencies. Higher contrast and better color perception is reported in work when dealing with anatomical and functional modality.
-
-
CONCLUSION
-
With extensive work done in literature medical image fusion has reinforced diagnosis. The features from medical images provide intricate information. The heterogeneity in anatomical and functional modalities, add diagnostic value. It is not easy to recognize and extract the most relevant clinical information. This multi disciplinary research area has various aspects to be further explored. In this paper the aspects pertaining to the medical images and its clinical relevance is discussed. Images, processing and modalities have limitations. Various modalities
and their specific medical value is exemplified. It is challenging to summarise all information. These existing techniques and affecting attributes, in neuro imaging have a greater potential of progression.
REFERENCES
-
Hasegawa, B.H., Zaidi, H.: Dual-Modality Imaging: More Than the Sum of its ComponentsQuantitative Analysis in Nuclear Medicine Imaging, 2006, pp. 3581.
-
Kaur Gurpreet; Singh Sukhwinder; Vig Renu: 'Medicalfusion framework using discrete fractional wavelets and non-subsampled directional filter banks', IET Image Processing, 2020, 14, (4), p. 658-667, DOI: 10.1049/iet-ipr.2019.0948 IET Digital Library, https://digital- library.theiet.org/content/journals /10.1049/iet- ipr.2019.0948
-
3. Amy B. Hershey Pa. The history of radiation use in medicine. Journal of Vascular Surgery. Published 27 September 2010;53(1):3S-5S. DOI: 10.1016/j.jvs.2010.07.024.https://doi.org/10.1016/j.jvs.2010.07.024
-
web 13. 4. Perry S. Effective physics knowledge for diagnostic radiologists. The universal image characteristics that affect visibility. Medical Physics International Journal. 2019;7(3):261. http://www.mpijournal.org/pdf/2019-03/MPI-2019-03-p257.pdf
-
14. 5. Shaik N. A review on image processing applications in medical field. Research Journal of Pharmacy and Technology. 2017;10(10):3456- 3460. DOI: 10.5958/0974-360X.2017.00644.8
-
15. 6. Muralikrishna P. Medical image analysis based on deep learning approach. Multimedia Tools and Applications. 2021;80:24365-24398. DOI: 10.1007/s11042-021-10707-4. https://doi.org/10.1007/s11042-021- 10707-4
-
Z. Zhang, R. S. Blum, "A categorization of multiscale-decomposition- based image fusion schemes with a performance study for a digital camera application," Proceedings of the IEEE, vol. 87, no. 8, 1999, pp. 1315-1326.
-
M. Manchanda and R. Sharma, "An improved multimodal medical image fusion algorithm based on fuzzy transform," Journal of Visual Communication and Image Representation, vol. 51, 2018, pp.7694.
-
N. Lelenards, "Role of image fusion in medicaldosimetry," Eradimaging,2009.[Online].Available: http://www.eradimaging.com/site/article.cfm? ID=745.
-
Gurpreet Kaur, Sukhwinder Singh, Renu Vig,Medical image fusion framework for neuro brain analysis, Advances in Computational Techniques for Biomedical Image Analysis, Academic Press, 2020, Pages 57-69, ISBN 9780128200247, https://doi.org/10.1016/B978-0-12- 820024-7.00003-7.
-
J. Flusser, F. Sroubek and B. Zitova, "Image fusion: principles, methods and applications, in 15th European Signal Processing Conference (EUSIPCO), Poznan, Poland, 2007, pp. 1-60.
-
A. Goshtasby and S. Nikolov, Guest editorial, "Image fusion: Advances in the state of the art," The International Journal on Multi Sensor, Multi Source Information Fusion, An Elsevier Publication, vol. 8, no.2, pp. 114-118, 2007
-
Z. Wang, D. Ziou, C. Armenakis, D. Li and Q. Li, "A comparative analysis of image fusion methods," IEEE Transactions on Geoscience and Remote Sensing, vol. 43, no. 6, pp. 1391-1402, 2005.
-
I. De and B. Chanda, "A simple and efficient algorithm for multifocus image fusion using morphological wavelets," Signal Processing, vol. 86, no. 5, 2006 pp. 924-936.
-
J. Teng, X. Wang, J. Zhang and S. Wang, "Wavelet-based texture fusion of CT/MRI images," in 3rd International Congress on Image and Signal Processing (CISP), vol. 6, 2010, pp. 2709-2713.
-
V. D. Calhoun,Feature-Based Fusion of Medical Imaging Data," IEEE Transactions on Information Technology in Biomedicine, vol. 13, no. 5, 2009, pp. 711-720.
-
Z. Jingzhou, Z. Zhao, T. Jionghua, L. Ting and M. Zhiping. "Fusion algorithm of functional images and anatomical images based on wavelet transform." in BMEI09. 2nd International Conference on Biomedical Engineering and Informatics, IEEE, 2009, pp. 1-5.
-
Kaur, G., Singh, S., & Vig, R. (2019). Multimodal Image Fusion in Clinical Research. In International Journal of Recent Technology and Engineering (IJRTE) (Vol. 8, Issue 3, pp. 52025211). Blue Eyes Intelligence Engineering and Sciences Engineering and Sciences Publication – BEIESP. https://doi.org/10.35940/ijrte.c5820.098319
-
S. Angenent, E. Pichon and A. Tannenbaum, "Mathematical methods in medical image processing," Bulletin of the American Mathematical Society, vol. 43, no. 3, 2006, pp. 365-396.
-
T. Li, Y. Wang, C. Chang, N. Hu, Y. Zheng, "Color-appearance-model based fusion of gray and pseudo-color images for medical applications," Information Fusion, vol. 19, no. 1, 2014, pp. 103114.
-
Gurpreet Kaur, Sukhwinder Singh, and Renu Vig, Medical Fusion of CLAHE Images using SWT and PCA for Brain Disease Analysis Springer LNNS Series 2nd International Conference on Communication, Computing and Networking (ICCCN2018), NITTTR, Chandigarh, Springer , International, 29-30 March 2018
-
D. L. G. Hill, and D. J. Hawkes, "Medical image registration using voxel similarity measures in applications of computer vision in medical image processing," AAAI spring symposium series, 1994, pp. 3437.
-
M. Beaulieu, S. Foucher, L. Gagnon, "Multispectral image resolution refinement using stationary wavelet transform", in Proceedings of 3rd IEEE International Geoscience and Remote Sensing Symposium, 2003, pp. 4032-4034.
-
Kaur G, Vig R and Singh S 2017 A review on medical image fusion aspects and techniques, in Proceedings of 1st International Conference on Communication, Computing and NetworkingICCCN (NITTTR) Chandigarh, India.
AUTHORS PROFILE
Author-1
Photo
Gurpreet Kaur is working as a Associate professor at Post Graduate Department of Computer Scienec at Sri Guru Gobind Singh College (Co-ed), affiliated to Panjab University. She obtained her Masters in Engineering in Computer Science & Engineering from Thapar Institute of Engineering &Technology, Patiala. Her research interests are lie in the areas of Artificial Intelligence, Biomedical Processing, Medical Image Processing, Signal Processing and Machine Leaning.