
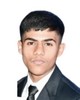
- Open Access
- Authors : Niev Sanghvi, Rahul Shankar Pachpande, Durgesh Hiralal Pawar, Tanishq Gandhi, Tejjas Bhingardevay, Paarth Pandey
- Paper ID : IJERTV14IS030193
- Volume & Issue : Volume 14, Issue 03 (March 2025)
- Published (First Online): 02-04-2025
- ISSN (Online) : 2278-0181
- Publisher Name : IJERT
- License:
This work is licensed under a Creative Commons Attribution 4.0 International License
The Role of Artificial Intelligence and Machine Learning in Analyzing and Predicting Social Media Trends for Executing Effective Marketing Strategies
Niev Sanghvi
The Bishops School Pune, Maharashtra, India
Tanishq Gandhi
Vellore Institute Of Technology, Vellore
Rahul Shankar Pachpande Kavayitri Bahinabai Chaudhari North Maharashtra University, Jalgaon
Tejjas Bhingardevay
Savitribai Phule Pune University
Durgesh Hiralal Pawar
P G College of Pharmaceutical Sciences and Research , Nandurbar
Paarth Pandey
Bhavans b.p Vidya Mandir, Civil Lines
AbstractThis research paper delves into the significance of Artificial Intelligence (AI) and Machine Learning (ML) in scrutinizing and forecasting social media trends to improve marketing strategies. In the ever-changing digital world of today, businesses encounter the task of adjusting to evolving social media trends, which have a substantial impact on consumer behavior and engagement with brands. Traditional marketing methods may struggle to keep up with these trends, potentially leading to missed opportunities.This research paper delves into important inquiries about the optimal utilization of AI and ML algorithms for analyzing and predicting social media trends. It also explores the impact of AI and ML-driven insights on marketing strategies, especially in audience targeting and content enhancement. Python solutions are seamlessly integrated, incorporating clustering for user segmentation and recommendation systems for tailored marketing campaigns. Automated A/B testing and regression models bolster real-time campaign adjustments and predictive ROI analysis. Graphical analyses illustrate the future implications of AI and ML in marketing, showcasing their potential to enhance audience targeting and engagement. This study showcases the transformative influence of AI and ML on marketing, providing businesses with advanced insights to stay competitive in a rapidly evolving digital market.
-
INTRODUCTION
The utilization of predictive analytics is a significant representation of how AI has influenced business paradigms. [1,2,3]. By delving into past data, AI algorithms demonstrate their ability to predict future trends, customer behaviors, and market dynamics.
Graph 1. Artificial Intelligence (AI) and Machine Learning (ML) in social media trends and marketing strategies.
[4-5] This expertise enhances businesses' ability to make data- informed decisions, reducing uncertainty and enhancing strategic vision. [6-7] In the realm of retail, predictive analytics has the ability to enhance inventory management by predicting demand in advance. This leads to improved cost savings and operational efficiency. In the realm of finance, AI-assisted predictive models have the ability to forecast market fluctuations, aiding in the development of strategic investment approaches and risk management initiatives. The effectiveness of predictive analytics is highlighted by its ability to quickly and accurately process large datasets[2-8].On the other hand, conventional statistical approaches frequently struggle with the complexity and scale of modern data collections.
In contrast, AI excels at discovering hidden patterns and correlations that human analysts may overlook, thus improving predictive accuracy and revealing new business opportunities, ultimately fueling innovation and growth.
-
Artificial Intelligence in Social Media Platforms : The development of Artificial Intelligence (AI) in social media platforms has revolutionized our interactions with these networks and how we access information. Initially, social media was primarily designed for connecting individuals and sharing content. However, with the surge in popularity of these platforms, they transitioned into collecting extensive data from users.This marks the point where AI began to play a major role.During the early 2010s, AI was predominantly utilized for simple tasks such as content recommendations and spam filtering.As technology progressed, AI evolved into a more sophisticated system.
Social media platforms have started utilizing machine learning algorithms to study user behavior, guiding businesses in comprehending user preferences and content engagement.This analysis allowed for the development of personalized experiences, crafted to suit individual preferences.Today, a variety of AI technologies, such as natural language processing and computer vision, are extensively utilized within the realm of social media.They support sentiment analysis, assessing public opinion on various subjects, and identifying trends nearly instantaneously.Moreover, AI tools support marketers in developing tailored advertising campaigns through the use of predictive analytics.
AI has transformed social media by enhancing its intelligence, increasing personalization, and improving efficiency for both users and businesses.
-
Significance of Machine Learning in Social Media Platforms :
Machine learning, a branch of Artificial Intelligence (AI), plays a crucial role in shaping the operations of modern social media platforms.It entails leveraging algorithms to examine data, glean insights, and forecast outcomes without the need for direct programming. On social media, machine learning enhances user experience, content delivery, and marketing strategies.One significant application of machine learning in social media involves tailored content recommendations. Through analyzing user behavior, including likes, shares, and comments, platforms can gain insights into users' preferences. This data enables algorithms to recommend posts, videos, or friends tailored to users' interests, thereby enhancing user engagement. Another crucial application that should not be overlooked is sentiment analysis. Machine learning algorithms possess the ability to analyze extensive amounts of social media posts, revealing overall sentiments or viewpoints on various subjects, companies, or occasions.Businesses can
utilize this data to adapt their marketing strategies in accordance with public sentiment. Machine learning also assists in recognizing patterns and forecasting user behavior. This enables marketers to develop tailored campaigns that effectively target specific audiences.Through the use of machine learning, social media platforms are able to enhance users' experiences by delivering more relevant and enjoyable content. This also empowers businesses to make informed decisions based on data, leading to better marketing results.
-
Artificial Intelligence and Machine Learning in shaping Market Strategies and analyzing trends : Artificial Intelligence (AI) and Machine Learning (ML) are pivotal in molding marketing strategies through trend analysis and real-time data utilization. Understanding trends is pivotal for businesses, as they shed light on consumer interests at any given moment.AI and ML technologies empower companies to identify these trends by analyzing large volumes of data gathered from social media, websites, and user engagements. One way artificial intelligence and machine learning assist in identifying trends is by analyzing user behavior on social media platforms.These advanced technologies have the capability to monitor user interaction with content, which includes activities like liking, sharing, and commenting. By analyzing patterns in this data, companies can determine which topics or products are becoming more popular.This understanding empowers marketers to adapt their approaches in orderto leverage these trends, showcasing products or content that truly connect with consumers. Furthermore, the effect of real-time data on decision-making is significant.By utilizing AI and ML, businesses can effortlessly access and analyze incoming data, enabling them to promptly adapt to evolving market trends.For instance, when a specific social media campaign gains traction, companies can promptly enhance their marketing strategies to capitalize on the increased attention. This agility is crucial in the fast-paced digital landscape of today, where consumer preferences can quickly change. Moreover, real-time data enables marketers to assess the impact of their campaigns.By monitoring responses and engagement levels in real time, companies can gather valuable insights to make informed decisions on what is effective and where adjustments are needed.This results in the development of more effective marketing strategies that closely match the interests and behaviors of consumers, leading to increased satisfaction levels and improved sales results. In general, the combination of AI and ML enables marketers to remain ahead of trends and make data-driven decisions swiftly and efficiently.
-
-
PROBLEM STATEMENT
In the current digital environment, businesses encounter the task of keeping up with swiftly changing social media trends that have a considerable impact on consumer behavior and brand interaction. Traditional marketing strategies can sometimes find it challenging to keep up with these changes, causing missed opportunities and ineffective campaigns. The merging of Artificial Intelligence (AI) and Machine Learning (ML) technologies is a promising solution for analyzing vast amounts of social media data. This integration enables businesses to identify and predict trends more accurately.
This research paper aims to address the following critical questions :
-
How can Artificial Intelligence and Machine Learning algorithms be best leveraged to analyze and forecast emerging social media trends? What specific methodologies yield the most precise results?
-
How can AI and ML-powered insights influence the development and implementation of marketing strategies, especially regarding audience targeting, content enhancement, and campaign performance assessment?
-
-
THE ROLE OF ARTIFICIAL INTELLIGENCE IN ANALYZING AND PREDICTING MARKET TRENDS
-
Data Collection techniques used by Artificial Intelligence Artificial Intelligence, commonly known as AI, fulfills a crucial role in collecting and analyzing social media data. This, in turn, assists businesses in comprehending trends and consumer behavior. Various data collection techniques are used to gather the copious information present on different social media platforms. One primary method involves manual monitoring, with marketers personally tracking posts and interactions. This method, unfortunately, is rather time- consuming and susceptible to human errors. Most businesses usually rely on automated data collection techniques supported by AI tools.
These tools are capable of effortlessly sifting through numerous posts, comments, and shares in a fraction of the time it would typically require a human. For instance, AI algorithms possess the ability to promptly detect keywords, hashtags, or phrases indicative of new trends. Another effective technique for delving into people's emotions towards particular subjects is sentiment analysis. AI can analyze the language used in social media posts to ascertain whether the sentiment is positive, negative, or neutral.
Graph 2. AI in Predicting Social Media Trends
Employing Natural Language Processing (NLP), an AI technology, allows companies to collect data and understand the emotions conveyed in user interactions. This capability allows businesses to enhance their marketing strategies by taking into account how consumers perceive their brand or products.
Overall, the integration of AI in data collection methods guarantees that marketers obtain valuable insights from social media trends, enabling them to remain in tune with consumer preferences and make well-informed decisions.
-
Role of Artificial Intelligence in Web Scraping and Application Programming Interface:
Web scraping and API usage are crucial techniques for data collection in social media analysis. These methods enable businesses to gather information efficiently. Web scraping is the procedure of extracting data from websites automatically. AI tools are capable of being programmed to browse through various social media platforms and gather pertinent information, for example, user posts, comments, and engagement metrics.
This technique enables marketers to gather extensive datasets that uncover valuable insights concerning consumer behavior, preferences, and trending topics. For example, a company might employ web scraping to examine numerous tweets concerning a particular product or event. By doing so, they can identify patterns in consumer sentiment and adapt their marketing strategies accordingly. On the flip side, Application Programming Interfaces (APIs) offer users a structured method for obtaining data from social media platforms. Numerous social media networks like Twitter and Facebook provide APIs enabling developers to access targeted data in a standardized format.
Graph 3. AI sentiments in predicting trends
Companies have the ability to utilize these APIs for accessing up- to-the-minute information on user engagement, including metrics like likes, shares, and comments. This capability allows businesses to promptly access the necessary information without facing the potential challenges associated with web scraping.
Both web scraping and API usage emphasize the effectiveness of AI in collecting social media data. This ensures that marketers can access the insights needed to identify trends and shape marketing strategies.
-
User-Generated Content and Engagement Metrics : User-generated content (UGC) significantly enhances the analysis of social media trends, particularly when coupled with powerful AI technologies.
User-generated content (UGC) encompasses all content generated by social media users such as posts, photos, videos, reviews, and comments. This content offers valuable insights into consumer opinions, experiences, and preferences. AI tools assist marketers in analyzing user-generated content to identify the topics or products that attract audiences most. By gathering and analyzing substantial amounts of user-generated content, companies can discover new trends and gain insight into consumer perceptions of their brand. Engagement metrics are a critical aspect that AI assists in analyzing. These metrics encompass likes, shares, comments, and various other forms of interaction that demonstrate people's engagement with content on social media. By analyzing engagement data, AI algorithms can uncover the most effective types of content and the factors that stimulate interaction between users.
For example, should a particular type of post receive significant engagement, companies can tailor their content to appeal to that audience, capturing their interest. The capacity of AI to analyze both user-generated content and engagement metrics empowers marketers to create impactful strategies that match consumer preferences.
Ultimately, this results in more powerful marketing campaigns that successfully engage target audiences and adjust to current trends.
-
-
THE ROLE OF MACHINE LEARNING IN ANALYZING AND PREDICTING MARKET TRENDS
Machine Learning has become a crucial element in the advancement of intricate business strategies, enabling the enhancement of operational processes, expansion of decision-making frameworks, and improvement of customer interaction approaches.
[9-10] By employing sophisticated algorithms to analyze and interpret vast datasets, ML provides crucial insights that support strategic initiatives, encourage innovation, and enhance overall effectiveness[11-13].Machine learning serves as a critical tool in enabling data- driven decision-making processes by deriving actionable insights from complex datasets.
[14-15] Business leaders have the ability to utilize ML models to analyze market dynamics, competitive strategies, and internal performance benchmarks. This enables them to develop more informed strategic plans[16-17]. ML methodologies are beneficial in refining pricing strategies by balancing competitiveness with profitability, and conducting scenario assessments to enhance organizational preparedness for various market contingencies and uncertainties[18].-
Supervised Learning Techniques in Machine Learning : Supervised learning is a form of machine learning in which algorithms are trained on labeled data. In this method, the input is paired with the corresponding output. This particular method is essential when it comes to forecasting trends on social media.Smooth version:This particular method is vital for predicting trends on social media. For example, classification algorithms like Decision Trees and Support Vector Machines (SVM) can aid in organizing social media posts into various trend categories.Decision Trees operate by developing a model that forecasts the value of a target variable through grasping uncomplicated decision rules derived from the characteristics of the data.This method is clear and easy to understand, which is why it is popular for analyzing patterns in social media. Regression analysis holds a crucial role in forecasting trends, especially when predicting future values using past data. When examining social media trends, regression analysis can delve into the impact of factors such as user engagement and post frequency on the popularity of a trend over time. When using regression techniques, marketers can gain valuable insights into the factors driving upward trends, helping them refine their marketing strategies to great effect.
Graph 4. Machine Learning in predicting trends
-
Unsupervised Learning Techniques in Machine Learning : Unsupervised learning refers to the training of algorithms on data without labeled responses. A prominent technique in this category is clustering, which groups similar data points based on features. Algorithms such as K-means and Hierarchical Clustering are widely favored.
K-means operates by categorizing data into 'k' unique clusters, facilitating the recognition of trends in social media conduct. This technique aids in pinpointing groups of users with comparable interests or engagement patterns. Topic modeling is an essential technique within unsupervised learning.
It entails examining the text data from social media posts in order to categorize and summarize content without predefined labels. Employing techniques such as Latent Dirichlet Allocation (LDA), researchers can unveil concealed thematic structures within vast datasets. This helps reveal the primary topics influencing discussions on social media, allowing marketers to customize their messages and campaigns to coincide with current trends.
Graph 5. Machine Learning in analyzing user data from feedback, growth and predicting future growth.
-
Deep Learning Approaches:
Deep learning is an advanced form of machine learning that employs neural networks with multiple layers to scrutinize vast quantities of data. This method is highly successful in forecasting social media trends because it adeptly navigates the intricacies and subtleties of data.
Neural networks, inspired by the human brain, have the ability to grasp and depict complex patterns in social media interactions, thus helping in precise trend prediction. Natural Language Processing (NLP) is an essential component of deep learning that is applied in analyzing social media. NLP allows machines to comprehend human language, empowering them to analyze text from posts, comments, and hashtags. By utilizing NLP techniques, researchers can effortlessly evaluate public sentiment, detect emerging trends,and analyze the popularity of specific topics. For example, sentiment analysis can uncover users' real-time feelings towards specific brands or products, granting marketers crucial insights to quickly adjust their strategies.
-
-
CASE STUDIES AND APPLICATIONS
-
Successful Implementations of AI and ML in Marketing: Artificial Intelligence (AI) and Machine Learning (ML) have completely revolutionized the marketing industry, empowering companies to leverage extensive social media data for predicting trends and customizing their strategies. Numerous companies have effectively utilized AI and ML to boost their marketing endeavors, achieving remarkable outcomes.
One notable example is Coca-Cola, a global beverage leader known for its innovative marketing campaigns. Coca-Cola employs artificial intelligence to examine consumer interaction on social media platforms.By utilizing Natural Language Processing (NLP), Coca-Cola is able to extract valuable insights from user-generated content, like tweets and comments discussing their products.The company's "Freestyle" vending machines, offering customers the option to customize their beverages, are backed by AI-powered analytics that analyze preferences and trends.This data has a significant impact on product development and plays a key role in crafting tailored marketing campaigns that align with the preferences of consumers.
Another instance involves Netflix, the major streaming service that has transformed content marketing by employing AI algorithms.Netflix applies machine learning to examine viewer preferences and behavior on its platform. This involves monitoring users' viewing habits and interactions with various genres.This analysis extends to social media, where Netflix monitors the buzz and sentiment around various shows and movies.By anticipating trends, Netflix is able to customize its marketing strategies, emphasizing the promotion of
appropriate content at the opportune moment. Before the launch of a new season of a popular show, Netflix increases its social media marketing efforts. They interact with fan communities and develop shareable content that captures the audience's excitement.
Nike exemplifies the effective utilization of AI and ML to improve marketing results.Nike utilizes AI algorithms to analyze purchase data and keep up with social media trends.By keeping an eye on platforms like Instagram and Twitter, Nike is able to spot new sneaker trends and gauge consumer feedback on particular products. This proactive approach allows Nike to effectively tailor its marketing campaigns, ensuring they align smoothly with current trends. Nike frequently partners with influencers and organizes sneaker launch campaigns based on insights derived from AI analytics, resulting in heightened engagement and sales.
-
Analysis of Outcomes :
Coca-Cola's marketing campaigns powered by AI have not only resulted in higher engagement rates but have also allowed the company to introduce products that closely match current consumer preferences.For example, after conducting an analysis on social media, Coca-Cola launched special edition flavors inspired by popular social media discussions.Metrics indicate that these campaigns led to a notable rise in social media engagement, with certain campaigns garnering more than 500,000 mentions on Twitter shortly after being launched. Netflix has achieved extraordinary results by employing machine learning for content prediction and marketing. By incorporating data-driven insights into their marketing strategies, Netflix has effectively maintained high viewer retention rates, averaging around 93% globally. The company's knack for foreseeing shows that will captivate viewers leads to successful promotional efforts, frequently causing a surge in viewership of up to 60% for shows marketed in line with social media trends. Furthermore, Netflix revealed that more than 80% of its new viewers discover content via tailored recommendations. This highlights the success of their AI- powered tactics in boosting user engagement. Similarly, Nike has witnessed impressive advancements in sales and customer engagement by utilizing its data-driven marketing strategy.With the use of AI analytics, Nike discovered that the demand for personalized products was increasing.Their marketing strategies incorporated this insight, leading to the successful launch of the Nike By You program (formerly known as NIKEiD). This unique program allows customers to customize their footwear. In the year following the program's launch, Nike experienced a notable 30% surge in online sales. Their targeted social media campaigns, fueled by AI insights into consumer preferences, not only doubled engagement rates compared to past efforts but also proved to be highly successful. Overall, by utilizing AI and ML to forecast social media trends, companies have been able to not only improve their marketing strategies but also achieve tangible success.Metrics such as
ISSN: 2278-0181
Vol. 14 Issue 03, March-2025
engagement rates, viewer retention percentages, sales growth,
and social media mentions offer compelling proof of the favorable results arising from AI-powered initiatives.By leveraging these technologies, businesses can respond more rapidly to market changes and consumer preferences, ensuring that their marketing efforts remain relevant and effective.
-
-
ARTIFICIAL INTELLIGENCE AND MACHINE LEARNING IN EXECUTING EFFECTIVE MARKETING
-
Personalization of Marketing Campaigns:
The most impactful aspects of AI and ML in marketing is their capacity to tailor campaigns according to individual user behavior and preferences.
Graph 6. AI in Executing Effective Marketing
Graph 7. ML in Executing Effective Marketing
Personalization entails customizing marketing messages and product recommendations for individual users, enhancing the chances of engagement and conversion. AI technologies analyze immense amounts of data gathered from customer interactions, including browsing history, purchasing habits, and social media engagement.
Through the utilization of advanced algorithms, companies have the ability to categorize their audience into specific groups. This facilitates the creation of tailored advertisements that align with the unique interests of each group. An online bookstore has the ability to utilize Artificial Intelligence to examine a customer's past purchases and browsing habits. This allows them to suggest books that align with the customer's interests. This enhances not only the customer's shopping experience but also boosts the effectiveness of the marketing campaign. Recommendation algorithms, an essential component
of AI and ML, are extensively utilized to enrich user experience.
These algorithms forecast which products or content a user will probably prefer, taking into account their previous actions and those of comparable users.
Popular platforms such as Netflix and Amazon heavily depend on recommendation systems to maintain user engagement and drive further sales. Amazon's "Customers who bought this also bought" feature utilizes machine learning to recommend complementary products, tapping into the buying patterns of other customers to boost sales. The capacity to customize marketing campaigns brings about a fundamental change in how businesses engage with consumers.
-
Real-time Campaign Adjustments
Another notable benefit of artificial intelligence and machine learning in marketing is the capacity to implement immediate changes to campaigns by leveraging continuous data analysis.Traditional marketing strategies used to depend heavily on historical data and pre-planned adjustments. This approach could result in missed opportunities or ineffective tactics if market conditions shifted unexpectedly. Automated systems driven by AI have the ability to analyze real-time data and promptly adapt marketing strategies to enhance their effectiveness. For instance, in the course of a digital advertising campaign, AI tools have the ability to monitor metrics related to user engagement including click-through rates and social media interactions.If a specific ad is underperforming, the AI system can automatically adjust its content or target audience to enhance its performance.This high level of agility enables businesses to quickly adapt to trends or shifts in consumer behavior, enhancing the chances of campaign success.A/B testing is another field where the use of AI and ML significantly boosts marketing efficiency.A/B testing includes the comparison of two versions of a marketing asset – like an email, webpage, or ad – to determine the one that achieves superior performance. AI-driven optimization can efficiently analyze data from these tests, allowing marketers to quickly identify the most effective variations. By engaging in continuous learning, AI systems can adapt upcoming campaigns by leveraging insights obtained from A/B testing, thereby enhancing marketing strategies as time progresses.
Graph 8. Outcome of AI in Effective Marketing
-
ROI Analysis and Measurement The fusion of AI and ML leads to progress in assessing the return on investment (ROI) of marketing campaigns.It is essential to comprehend the impact of different marketing initiatives in order to enhance budget utilization and strategic planning.AI-powered tools provide marketers with sophisticated metrics and analytics to accurately assess campaign performance.
For instance, AI offers assistance in examining customer acquisition expenses, conversion rates, and customer lifetime value, offering businesses valuable insights on the effectiveness of various marketing channels. These metrics can guide decisions on budget allocation, allowing companies to increase investment in the most productive strategies and gradually phase out less effective approaches.Furthermore, AI tools have the capability to analyze extensive datasets in order to uncover trends and correlations that might not be readily apparent through conventional methods of analysis.Businesses may find that specific demographics respond better to certain marketing messages, which can result in more targeted and successful campaigns.
The integration of AI in marketing has a substantial long- term impact.Businesses that skillfully harness the power of AI and ML experience enhanced campaign results, and gain a more profound insight into their customer demographics.This understanding cultivates loyalty and boosts brand reputation, setting businesses up for long-term success in a highly competitive market.
Graph 9. Outcome of Machine Learning in Effective Marketing
-
-
CHALLENGES AND LIMITATIONS
-
Data Privacy Concerns :
One of the most critical challenges in applying AI and ML to analyze social media trends is the matter of data privacy. As the collection of user data continues to grow, there arises ethical considerations regarding the utilization, storage, and sharing of such information.
Regulations like the General Data Protection Regulation (GDPR) in the European Union have established stringent rules on data privacy. This mandates that businesses must secure explicit consent from users prior to gathering their personal information. Companies need to make sure that they adhere to these laws, a situation that can make the deployment of AI technologies more complex.
For example, companies must apply strong data security measures to safeguard consumer information from security breaches. This involves encrypting data, ensuring secure storage solutions, and conducting regular audits to evaluate vulnerabilities. Non-compliance with data privacy regulations does not only lead to significant fines but also harms a company's reputation. Consumers are becoming more conscious of their privacy rights, and they anticipate companies to handle their data with responsibility. Organizations must find a way to balance harnessing data for AI-driven insights while also honoring consumer privacy.
-
Accuracy and Reliability of Predictions :
One more challenge that companies encounter when utilizing AI and ML in their marketing endeavors lies in the precision and dependability of their forecasts. Although AI algorithms are capable of analyzing large volumes of data and recognizing patterns, they are not perfect.
One of the significant risks is algorithm biaswhen algorithms produce results that are skewed or biased based on the data used to train them. For instance, when an AI model is trained on historical data that contains racial or gender biases, it might perpetuate these biases in its predictions, resulting in unjust treatment of specific consumer groups.
IJERTV14IS030193
Graph 10. Challenges and limitations faced by AI and ML
Furthermore, erroneous forecasts may arise as a result of obsolete or insignificant data. Social media trends have the tendency to evolve quickly, and failing to consistently update the AI model with the most recent data could result in the development of inaccurate marketing strategies. Companies might allocate resources to campaigns that are built on inaccurate predictions, ultimately leading to squandered funds and overlooked chances.
Hence, it is crucial for businesses to consistently assess and enhance their AI models, ensuring they operate with up-to- date and relevant data.
-
Technology Adoption Barriers :
Businesses encounter multiple obstacles when implementing AI and ML technologies.
The most significant challenge is cost. Implementing advanced AI systems may necessitate a significant investment in technology and infrastructure. Furthermore, it is crucial for companies to factor in the continuous expenses associated with the upkeep and modernization of these systems.
For small to mid-sized companies, these expenses can be prohibitive, leading them to hesitate in adopting AI solutions. Another obstacle arises from a deficiency in knowledge and skills. Successful implementation of AI and ML technologies often demands specialized knowledge and skills.Numerous enterprises may lack in-house data scientists or engineers proficient in handling and deciphering AI-generated insights. This talent gap can impede companies from fully realizing the advantages of AI technology. Investing in training or hiring experts can be time-consuming and expensive, potentially deterring some organizations from embarking on AI-driven initiatives.
In conclusion, the realms of AI and ML present thrilling opportunities in forecasting social media trends and amplifying marketing approaches. However, companies need to cautiously tackle the accompanying obstacles. Data privacy concerns require strict compliance with regulations and ethical standards. Accuracy and reliability concerns underscore the importance of regularly updating and assessing AI models.
Moreover, obstacles such as expenses and a shortage of knowledge can hinder the adoption of technology. Addressing these challenges will be crucial for businesses seeking to effectively harness the power of AI and ML in their marketing efforts while maintaining trust and integrity with their consumer base.
-
FUTURE IMPLICATION
-
Emerging Trends in AI and ML for Marketing : The future of AI and ML in marketing is full of promise, with numerous innovative technologies on the horizon.A notable trend in the field involves the evolution of sophisticated algorithms that can effectively analyze extensive data sets. The algorithms are getting more advanced day by day, enabling marketers to delve deeper into understanding consumer behavior and preferences. Predictive analytics is set to see a significant enhancement. This advancement will empower companies to predict customer needs in advance, thus enhancing the effectiveness of their marketing strategies. Another intriguing development is the potential influence of quantum computing on artificial intelligence and machine learning. Quantum computing holds the potential to handle information at incredible speeds, potentially transforming the way data is analyzed.By utilizing the potential of quantum algorithms,marketers have the ability to perform intricate simulations and analyses instantly. This allows for campaigns to be optimized on-the-fly, resulting in enhanced personalized experiences for consumers. This level of computational power could bring about significant transformations in the realm of data- driven marketing.Moreover, the emergence of natural language processing (NLP) is anticipated to elevate customer engagements by refining chatbots and virtual assistants. These AI-driven tools will continue to enhance their ability to comprehend and address customer inquiries in a more human-like manner, thereby enhancing user experience and promoting engagement.
-
Predictions for Future Use in Social Media Marketing : AI tools can already create articles, social media posts,and marketing copy. As these tools advance, companies will be able to generate top-notch content efficiently, customized for distinct audience groups, all the while upholding brand messaging consistency. Another important progress will involve the enhancement of sentiment analysis.AI systems are improving in interpreting not only words but also context, tone, and emotions.
-
-
Graph 11. Future implications of AI and ML in predicting trends for effective marketing.
This advancement enables brands to promptly grasp consumers' sentiments towards their products or campaigns. This feature offers the possibility for more vibrant engagement tactics, empowering brands to tailor their messages according to public sentiment.Social media platforms will use AI and ML to enhance audience targeting with increased precision.As algorithms improve in analyzing user behavior and preferences, marketers can craft highly targeted ads tailored to reach the right audience at the perfect moment, thus enhancing engagement and boosting conversion rates.In conclusion,the potential held by AI and ML in marketing's future is truly great. With the progression of new technologies and ongoing developments, businesses can enhance their strategies, engage with consumers more efficiently, and stay ahead in a constantly changing digital environment.
-
PYTHON INTEGRATED SOLUTIONS FOR PROBLEM STATEMENT AND CRITICAL QUESTIONS
In the current digital environment, businesses encounter the task of keeping up with swiftly changing social media trends that have a considerable impact on consumer behavior and brand interaction. Traditional marketing strategies can sometimes find it challenging to keep up with these changes, causing missed opportunities and ineffective campaigns. The merging of Artificial Intelligence (AI) and Machine Learning (ML) technologies is a promising solution for analyzing vast amounts of social media data. This integration enables businesses to identify and predict trends more accurately.
This research paper aims to address the following critical questions :
-
How can Artificial Intelligence and Machine Learning algorithms be best leveraged to analyze and forecast emerging social media trends? What specific methodologies yield the most precise results?
-
How can AI and ML-powered insights influence the develoment and implementation of marketing strategies, especially regarding audience targeting, content enhancement, and campaign performance assessment?
-
Personalization of Marketing Campaigns : User Segmentation with Clustering : Using clustering algorithms to segment the customers based on behavior data.
Fig 1. Python code for personalization of marketing campaigns
Recommendation Systems : Implementing a recommendation engine using collaborative filtering can enhance user experience.
Fig 2. Python code for Recommendation Systems
-
Real-time Campaign Adjustments :
Automated A/B Testing with Real-time Data : Use a library like statsmodels to analyze A/B test results.
Fig 3. Python code for Automated testing with real time data
-
ROI Analysis and Measurement : Predictive Analytics for ROI Tracking :
Use regression models to predict outcomes and measure ROI.
Fig 4. Python code for ROI Analysis and Measurement
These Python-based solutions provide a starting point for addressing common challenges in AI and ML for marketing. By leveraging clustering, recommendation systems, A/B testing, and predictive analytics, you can enhance the effectiveness of your campaigns.
-
-
-
CONCLUSION
In conclusion, this research paper delves into the transformative impact of Artificial Intelligence (AI) and Machine Learning (ML) on analyzing and forecasting social media trends to enhance the implementation of successful marketing strategies. By delving into crucial inquiries such as enhancing AI's ability to collect and analyze extensive information from social media, and finding solutions for obstacles like data privacy, the
research highlights significant issues within contemporary marketing.By incorporating Python-based tools like Natural Language Processing and sentiment analysis, the paper showcases how businesses can tailor their strategies to meet consumer preferences. This is depicted through graphs illustrating trends and engagement metrics. Ultimately, artificial intelligence and machine learning enhance marketers' capacity to analyze present trends and enable them to forecast future developments. This ensures that marketing endeavors stay pertinent and effective.
REFERENCE
-
Loureiro, S. M. C., Guerreiro, J., & Tussyadiah, I. (2021). Artificial intelligence in business: State of the art and future research agenda. Journal of business research, 129, 911-926.
-
Akerkar, R. (2019). Artificial intelligence for business. Springer
-
] Ruiz-Real, J. L., Uribe-Toril, J., Torres, J. A., & De Pablo, J. (2021). Artificial intelligence in business and economics research: Trends and future. Journal of Business Economics and Management, 22(1), 98- 117.
-
, ., & , . (2024). Influence of Artificial Intelligence on Business Decision-Making. Mechanism of an economic regulation, (1 (103)), 17-23.
-
Getchell, K. M., Carradini, S., Cardon, P. W., Fleischmann, C., Ma, H., Aritz, J., & Stapp, J. (2022). Artificial intelligence in business communication: The changing landscape of research and teaching. Business and Professional Communication Quarterly, 85(1), 7-33.
-
Canhoto, A. I., & Clear, F. (2020). Artificial intelligence and machine learning as business tools: A framework for diagnosing value destruction potential. Business Horizons, 63(2), 183-193.
-
Gomes, P., Verçosa, L., Melo, F., Silva, V., Filho, C. B., & Bezerra,
B. (2022). Artificial intelligence-based methods for business processes: A systematic literature review. Applied Sciences, 12(5), 2314.
-
Dirican, C. (2015). The impacts of robotics, artificial intelligence on business and economics. ProcediaSocial and Behavioral Sciences, 195, 564-573.
-
] Reis, C., Ruivo, P., Oliveira, T., & Faroleiro, P. (2020). Assessing the drivers of machine learning business value. Journal of Business Research, 117, 232-243.
-
Bose, I., & Mahapatra, R. K. (2001). Business data mininga machine learning perspective. Information & management, 39(3), 211-225.
-
Pramanik, P., & Jana, R. K. (2023). Identifying research trends of machine learning in business: a topic modeling approach. Measuring Business Excellence, 27(4), 602-633.
-
Bharadiya, J. P. (2023). Machine learning and AI in business intelligence: Trends and opportunities. International Journal of Computer (IJC), 48(1), 123-134.
-
Singh, D. A. A. G., Leavline, E. J., Muthukrishnan, S., & Yuvaraj, R. (2018). Machine learning based business forecasting. International Journal of Information Engineering and Electronic Business, 14(6), 40.
-
Raturi, R. (2018). Machine learning implementation for business development in real time sector.
International Journal of Pure and Applied Mathematics, 119(15), 1289-1300.
-
Finlay, S. (2017). Artificial intelligence and machine learning for business. A no-nonsense guide to data driven technologies, 3.
-
Afolabi, I., Ifunaya, T. C., Ojo, F. G., & Moses, C. (2019, August). A model for business success prediction using machine learning algorithms. In Journal of Physics: Conference Series (Vol. 1299, No. 1, p. 012050). IOP Publishing.
-
Larsen, K. R., & Becker, D. S. (2021). Automated machine learning for business. Oxford University Press.
-
N. L. Rane, M. Paramesha, S. P. Choudhary, and J. Rane, Artificial Intelligence, Machine Learning, and Deep Learning for Advanced Business Strategies: A Review, Partners Universal International Innovation Journal, vol. 2, no. 3, pp. 147171, Jun. 2024, doi: 10.5281/zenodo.12208298.