
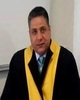
- Open Access
- Authors : Hayder Kareem Hammood , Bushra Kadhum Awaad , Oday Hatem Jalil
- Paper ID : IJERTV12IS010091
- Volume & Issue : Volume 12, Issue 01 (January 2023)
- Published (First Online): 01-02-2023
- ISSN (Online) : 2278-0181
- Publisher Name : IJERT
- License:
This work is licensed under a Creative Commons Attribution 4.0 International License
Truncated Rayleigh Lomax Distribution
Hayder Kareem Hammood 1, Bushra Kadhum Awaad 2, Oday Hatem Jalil 3
1 Babylon Education Directorate, Babylon, Iraq.
2 College of Education of Pure Sciences, University of Kerbala, Kerbala, Iraq.
3 Assistant teacher – General Directorate of Education, Babylon Governorate.
Abstract:- The Truncated Rayleigh – Lomax distribution is introduced in this research. To do this, we interrupt the period of Rayleigh-Lomax to create a distribution with more flexibility and efficiency. Then we extracted the statistical and mathematical characters of the distribution, like, cumulative distribution, survival, density, Hazard, and cumulative Hazard functions. We also found the arithmetic mean, median, mode, order statistics, and moment-generating function extracted the Kurtosis and Skewedness, Variation Coefficients, and presented some analysis methods. Finally, we compared the new truncated Rayleigh-Lomax distribution with the original Rayleigh-Lomax distributions and some other distributions. The results showed that the truncated Rayleigh -Lomax distributions was better than the Rayleigh -Lomax distribution.
Keywords: Cumulative function, density, Reliability, moment generating function, reverse hazard, hazard, cumulative hazard function, mean and variance, mode, median, quintile, order statistics, moments, truncated Rayleigh Lomax distribution.
INTRODUCTION
We are sometimes required by design that is forced to truncate some of the distributions observations or delete them to take advantage of the time. In this case, only the sample taken from the truncated distribution is used for estimation purposes, and removing part of the possible values for this distribution, is done in one or two parts. Generally, any value outside the
period [, ] is ignored.
Since (; ) represents the truncated probability distribution function. That is, this function fulfills the conditions of
the probability density function, i.e. 0 and (; ) = 1 or ( ( = ; ) = 1). It is important to remember that
the truncation process changes the original distributions mean, variance, and other statistical measures.
The goal of discovering or developing the distribution is new because of its importance, usefulness, and practical and scientific reality in several areas of life, such as biostatistics or the analysis of survival function. So in this research, a new distribution was found: the truncate Rayleigh-Lomax distribution. This is done by truncating the period for the distribution of Rayleigh-Lomax.
-
Truncated Rayleigh Lomax distribution.
The distribution in which the period truncated at point a, in which a is a constant. i.e., All of the values chosen at random
are in the range 0 < < .
-
PDF and CDF of TRLD
1 (1+)
( ; , , ) 22 (1 + )1 22
(; , , ) = () () = 1 , 1 22(1+)
(1)
The pdf of the (TRLD) is given by
1 & , , > 0
1
(1+)1 22(1+)
Such as 2 2 = 1
1
1
1 (1+) 22
For the (TRLD), the corresponding plot to pdf is as follows:
1
0.9
0.8
b=0.9
d=0.2,a=0.6
d=0.6,a=1.1
d=1.2,a=1.4
d=1.4,a=1.6
probability density function
0.7
0.6
0.5
0.4
0.3
0.2
0.1
0
0 1 2 3 4 5 6
x-axis
Figure.1: p.d.f plotting of (TRLD), parameters b=0.9, theta=0.6,1.1,1.4,1.6 ; d=0.2,0.6,1.2,1.4
theta=1.9
1
0.9
0.8
b=0.9,d=0.6
b=1.2,d=0.8
b=1.7,d=1.4
b=2.2,d=1.9
probability density function
0.7
0.6
0.5
0.4
0.3
0.2
0.1
0
0 0.5 1 1.5 2 2.5 3 3.5 4
x-axis
Figure.2: p.d.f plotting of (TRLD), parameters theta=1.9, b=0.9,1.2,1.7,2.2 ; d=0.6,0.8,1.4,1.9
beta=1.3
1
0.9
0.8
b=1.4,a=1.9
b=1.1,a=1.6
b=0.9,a=0.7
b=0.6,a=0.4
probability density function
0.7
0.6
0.5
0.4
0.3
0.2
0.1
0
0 1 2 3 4 5 6
x-axis
Figure.3: p.d.f plotting of (TRLD), parameters beta=1.3, b=1.4,1.1,0.9,0.6 ; a=1.9,1.6,0.7,0.4
Figures (1), (2) and (3) indicate that the TRPD family generates various shapes such as symmetrical, left skewed, right skewed and reversed- J
1
(1 + )1 22(1+)
(; , , ) = (; , , ) = 22
1
1
1
1 22(1+) 1
= 1 , & , , > 0 1 22(1+)
(2)
1
1 22(1+)
theta=4.7
1
0.9
0.8
cumulative distribution function
0.7
0.6
0.5
0.4
0.3
0.2
0.1
0
b=0.4,d=0.3
b=0.6,d=0.5
b=0.8,d=0.7
b=0.9,d=1.1
0 0.5 1 1.5 2 2.5 3
x-axis
Figure.4: c.d.f plotting of (TRLD), parameters theta=4.7, b=0.4,0.6,0.8,0.9 ;d =0.3,0.5,0.7,1.1
b=0.4
1
0.9
0.8
cumulative distribution function
0.7
0.6
0.5
0.4
0.3
0.2
0.1
0
a=2.9,d=2.3
a=2.1,d=1.9
a=1.8,d=1.7
a=1.6,d=1.5
0 0.5 1 1.5 2 2.5 3 3.5 4 4.5 5
x-axis
Figure.5: c.d.f plotting of (TRLD), parameters b=0.4, a=2.9,2.1,1.8,1.6 ; d =2.3,1.9,1.7,1.5
beta=1.7
1
0.9
cumulative distribution function
0.8
0.7
0.6
0.5
a=1.4,b=1.3
0.4 a=1.1,b=0.9
a=0.8,b=0.7
a=0.3,b=0.5
0 0.5 1 1.5 2 2.5 3 3.5 4
x-axis
Figure.6: c.d.f plotting of (TRLD), parameters beta=1.7, a=1.4,1.1,0.8,0.3 ; b=1.3,0.9,0.7,0.5
Figures (4), (5), and (6) show that when x and the parameters , , are increased, the cdf of the TRPD does not decrease. Figures (4), (5), and (6) demonstrate that the cdf of the TRPD does not decrease when and the parameters, , , are increased.
-
Limitation of c.d.f and p.d.f
lim (; , , ) = 0
1
(3)
The distributions limitations are determined via:
1 (1+)
(1 + )1 22
lim 22 = 0
1
1 (1+) 1 (1+)
(1+)1 22 (1+)1 22
lim (; , , ) = lim 2 2 = 2 2 > 0
1 (1+) 1 (1+)
1 22 1 22
(4)
Also,
1 (1+)
1 22
1
Because 1 22(1+)
> 0 and
1
2
1 (1+)
22 (1 + )
2 > 0
1
1 22(1+)
lim (; , , ) = lim = 0
1 1 1 (1+)
1 22
(5)
As a result, this distributions c.d.f. is as follows:
1
1 22(1+)
lim (; , , ) = lim 1 = 1
1 22(1+)
(6)
Also,
i.e 0
(; , , ) 1
-
Some Survival Functions
In this section, some survival functons for the TRLD will be introduced.
-
Survival Function
1 1 1
1 22(1+) 22(1+) 22(1+)
() = 1 () = 1 1 = 1
1 22(1+) 1 22(1+)
(7)
The survival function of TRLD is given by:
Such as,
1
1
lim () = lim
22(1+)
22(1+)
= 1
1
1
1 (1+)
1 22
12(1+)
lim () = lim 2
1 (1+)
2
2 = 0
1 (1+)
1 22
That is 1) () 0, () 1 1
2) () 1, () 0 0
The plot of the () and the () for the(TRLD) as follows.
0.9
0.8
0.7
theta=3.9
b=0.5,d=0.7
b=0.6,d=0.8
b=0.7,d=0.9
b=0.9,d=1
reliability function
0.6
0.5
0.4
0.3
0.2
0.1
0
0 0.5 1 1.5 2 2.5 3
x-axis
Figure.7: Plotting of () for (TRLD), parameters theta=3.9, b=0.5,0.6,0.7,0.9 ; d=0.7,0.8,0.9,1
1
0.9
0.8
b=0.4
a=2.8,d=2.6
a=1.9,d=1.7
a=1.6,d=0.9
a=0.9,d=0.8
0.7
reliability function
0.6
0.5
0.4
0.3
0.2
0.1
0
0 0.5 1 1.5 2 2.5 3 3.5 4
x-axis
Figure.8: Plotting of () for (TRLD), parameters b=3.9, a=2.8,1.9,1.6,0.9 ; d=2.6,1.7,0.9,0.8
beta=3.8
0.9
0.8
0.7
a=0.4,b=0.3
a=0.6,b=0.5
a=0.8,b=0.7
a=0.9,b=0.9
reliability function
0.6
0.5
0.4
0.3
0.2
0.1
0
0 1 2 3 4 5 6 7 8 9 10
x-axis
Figure .9: Plotting of () for (TRLD), parameters beta=3.8, a=0.4,0.6,0.8,0.9 ; b=0.3,0.5,0.7,0.9
The () of the TRPD is shown in Figures (7), (8), and (9) to be a decreasing function.
-
Hazard Function
1 (1+)
( ; , , ) 22 (1 + )1 22
() = () = 1 1
22(1+) 22(1+)
(8)
The hazard function for TRLD is given by:
Figure.10: Plotting of () for (TRLD), parameter theta=3.2, b=3,4,5,6 ; d=0.5,1,1.5,2
3.5
a=1.6,d=1.5
a=1.7,d=1.6
b=2.6
3 a=1.8,d=1.7
a=1.9,d=1.8
2.5
hazard rate function
2
1.5
1
0.5
0
0 0.5 1 1.5 2 2.5 3 3.5 4
x-axis
Figure.11: Plotting of ()of the (TRLD) for parameter b=2.6, a=1.6,1.7,1.8,1.9 ; d=1.5,1.6,1.7,1.8
beta=2.8
30
a=1.1,b=1.4
a=1.4,b=1.6 25 a=1.5,b=1.7
a=1.6,b=1.8
hazard rate function
20
15
10
5
0
0 0.5 1 1.5 2 2.5 3 3.5 4
x-axis
Figure.12: Plotting of () for (TRLD), parameter beta=2.8, a=1.1,1.4,1.5,1.6 ; b=1.4,1.6,1.7,1.8.
-
Reverse Hazard Function
1 (1+)
( ; , , ) 22 (1 + )1 22
() = (; , , ) = 1
1 22(1+)
(9)
The reverse hazard function for TRLD is:
-
The Cumulative Hazard Function
1 1
22(1+) 22(1+)
() = () = 1
1 22(1+)
(10)
The formulation for the TRLD cumulative hazard function is:
Such as, , , , > 0
-
-
Some properties of the TRLD :
-
mode: Proposition1:
2 1
(2 ( 1)) 1
=
(11)
The mode of the TRLD is.
(, , , )
= = 0
(12)
Proof:
1
2
1 (1+)
(, , , )
=
[22 (1 + ) 2
1
1 22(1+)
] = 0
[ + 22 + ( 1)(1 + ) 1 (1 + ) ( 1 22(1+) )]=0
1
22
( 1) (1 +
) 22 (1 + )
= 0
2 1
(2 ( 1)) 1
=
(13)
( 1) (1 +
) = 22 (1 + )
1
-
Quintile and Median:
Proposition 2
For the TRLD, the formula for the quintile and the median is as follows:
1
1
[ 22 ( 1 [ 1 22(1+) ]] 1(14)
=
1 1
[ 22 ( 1 1 + 22(1+)2 [ ]] 1
=
(15)
Proof:
From equation (3), obtain.
1
1 22(1+)
1
1 22(1+)
= 1
22
1 (1+)
= [ 1
1 (1+) 22 ]
1
1
22(1+)
= 1 [ 1 22(1+) ]
Take Ln to the two parties
1
1 (1+)
22
(1 + ) = (1 [ 1
22 ])
Then,
1
1
=
[ 22 ( 1 [ 1 22(1+) ])] 1(16)
Changing = 1 in equation (16) for TRLDs median yields:
2
1
1
[ 22 ( 1 1 1 22(1+) ])] 1 [=
1 1
[ 22 ( 1 1 + 22(1+)2 [ ]] 1
=
(17)
2
5.3.Moment Generation Function:
Proposition3
() = () 1) ()(+1)+
(
=0 =0 =0 =0
(1)[()(+1)+ 1 (+1)+
( ) ]
× 1
(!)(!)(22)+1(1 22(1+))(( + 1) + )
(18)
The TRLD moment generation function is the formula:
Proof:
1
2 (1 + )
1 (1+) 22
() = ( ) = 2
1
1
1 22(1+)
=0
By using series expansion of get =
So,
!
() = ( ) (
1
) ()
(+1) ( 1)
1
=0 =0 =0 =0
(!)(!)(22)+1(1 22(1+) )
× (+1)+1
1
() = ) 1) ()(+1)+
( (
=0 =0 =0 =0
(1)[()(+1)+ ( 1 )(+1)+]
×
1
(!)(!)(22)+1(1 22(1+) )(( + 1) + )
(19)
Then,
-
-
Moments Theorem1:
1
() = ( ) ( ) ()(+1)
=0 =0 =0
(1)[()(+1)++1 (1)( + 1)(+1)+]
×
1
! (22)+1[1 22(1+)] (( + 1) + )
(20)
The TRLDs moment with respect to the origin and moment with respect to the mean are as follows:
1
( ) = ( ) ( ) ( ) () ()(+1)
=0 =0 =0 =0
(1)[()(+1)++1 1)(+1)++1
( ]
× 1
! (22)+1[1 22(1+) ] (( + 1) + + 1)
(21)
r=1,2,3,.n
Proof: Regarding the origin, the rth is:
1
22
(1 + )1
(1+)
( ) = (; , , ) = 22
1
1 22(1+)
1
1
1 (1+)
= 1
22[1 22(1+) ]
(1 + )1
22
Then,
1
1
(1)
() = ( ) (
=0 =0 =0
) ()(+1)
! (22)+1[1
1 (1+) 22 ]
× (+1)+1
1
Then,
1
() = ( ) (
=0 =0 =0
) ()(+1)
(1)[()(+1)+ ( 1 )(+1)+]
1
×
! (22)+1[1 22(1+)] (( + 1) + )
(22)
when r = 1,2
1
() = ( ) ( ) ()(+1)
=0 =0 =0
(1)[()(+1)+1 ( 1 )(+1)+1]
×
1
! (22)+1[1 22(1+)] (( + 1) + 1)
(23)
1
(2) = ( ) ( ) ()(+1)
=0 =0 =0
(1)[()(+1)+2 (1)(+1)+2]
×
1
! (22)+1[1 22(1+)] (( + 1) + 2)
(24)
() = (2) [()]2
(25)
The mean's rth moment is provided via:
1
(1 + )1 22(1+)
( ) = ( ) 22
1
1
1 22(1+)
Where
( ) = (
=0
) ()()
1
( ) = ( ) ( ) ( ) () ()(+1)
=0 =0 =0 =0
(1)[()(+1)++1 ( 1 )(+1)+]
×
1
! (22)+1[1 22(1+) ] (( + 1) + )
(26)
Therefore, mean's rth moment is provided via:
-
Order Statistics
Suppose that x1, x2,.,xn denoted a r.s for the size n from a TRLD with (; , , ) and (; , , ) in the equation (1) and (2) . Let 1, 2, . , denote the correlating orders statistical; so, the p.d.f of Xk:n is provided via:
!
1
,(, , , ) = (1)!()! (; , , )[(; , , )] [1 (; , , )]
1 (1+)
! 22 (1 + )1 22
,(, , , ) = ( 1)! ( )! [ 1 ]
1 (1+)
22
1 1 1 1
1 22(1+) 22(1+) 22(1+)
× [ 1 ] [ 1 ] 1 22(1+) 1 22(1+)
(27)
The rth order statistics p.d.f. is derived from the TRLDs p.d.f.
1 1 1
(1+)122(1+) (1+) (1+)
(, , , ) = 2 2 22 22 ]1
1, 1 [ 1
(1+) (1+)
1 22 1 22
(28)
So, we can define the median, maximum, and minimum p.d.f. as follows: 1- p.d.f of minimum if k=1:
1
(1 + )1 22(1+) 1 (1+)
2 2 1 22
,(, , , ) = 1 [ 1 ]1 1 22(1+) 1 22(1+)
(29)
-
p.d.f of maximum if k=n:
1 (1+) 1
! 22 (1 + ) 22 1 22(1+)
+1,(, , , ) = [ ][ ][1
! ( 1)! 1 (1+) 1 (1+)
1 22 1
22
1
1 22(1+)
1 ]1 1 22(1+)
(30)
-
p.d.f of median if k=m+1:
-
-
Skewedness, Kurtosis, and Variation Coefficients
In this section, we introduce and study Skewedness, kurtosis and variation of (TRLD) based on the moment as the following proposition.
Proposition 4
( )3
=
3
(31)
The, variation coefficients, skewedness-kurtosis of the TRLD are presented via:
Let =
By equation ( 26), get
3
3
1
=
( ) ( ) (
) ()3 ()(+1)
=0 =0
=0 =0
(1)[()(+1)++1 (1)(+1)+]
1
×
! (22)+1[1 22(1+) ] (( + 1) + )
2
1
= [
=0 =0
( ) ( ) (
=0 =0
) ()2 ()(+1)
1
(1)[()(+1)++1 ( )(+1)+]
( )4
=
4
1
! (22)+1[1 22(1+)] (( + 1) + )
(32)
×
3
]2
Let =
By equation (26), get
4
4
1
=
=0 =0
( ) ( ) (
=0 =0
) ()4 ()(+1)
1
2
(1)[()(+1)++1 ( )(+1)++1]
× 1
! (22)+1[1 22(1+) ] (( + 1) + + 1)
= [
=0 =0
2
(
=0 =0
) (
1
) (
) ()2 ()(+1)
( ]
(1)[()(+1)++1 1)(+1)++1
=
1
! (22)+1[1 22(1+) ] (( + 1) + + 1)
(33)
× ]2
Let =
2
2
1
= [
=0 =0
( ) ( ) (
=0 =0
) ()2 ()(+1)
1
(1)[()(+1)++1 ( )(+1)++1]
× 1
1
]2
1
! (22)+1[1 22(1+) ] (( + 1) + + 1)
= ( ) (
=0 =0 =0
Proposition 5:
) ()(+1)
(1)[()(+1)+1 (1)(+1)+1]
1
×
! (22)+1[1 22(1+)] (( + 1) + 1)
1 1
= ( ) = ( ) ( )
=0 =0 =0
(+1)(1)[()(+1)1 1 (+1)1
( ) ]
× 1
! (22)+1[1 22(1+)](( + 1) 1)
(34)
The harmonic mean is given by:
Proof:
1
1
1
1 22 (1 + )
1 (1+) 22
= (
) =
(; , , ) =
1
1
1
1 22(1+)
1 (1+)
= 1
22[1
(1+)]
1(1 + )1
22
Thus,
1
22 1
1
(+1)
( ) =
1
22 [1 22(1+) ]
( ) (
=0 =0 =0
) ()
( 1 )
× 22
!
(+1)2
Thus,
1 1
( ) = ( ) ( )
=0 =0 =0
(+1)(1)[()(+1)1 ( 1 )(+1)1]
×
1
! (22)+1[1 22(1+) ](( + 1) 1)
(35)
1
Proposition 6:
1
= () = ( ) ( )
=0 =0 =0
1
(+1)(1)[()(+1)1 ( 1 )(+1)+ ]
2
×
1
! (22)+1[1 22(1+) ](( + 1) + 1 )
2
Proof:
(36)
The formula for the geometrical mean is:
= ( ) = 1 (; , , )
1
(1 + )1
1 (1+)
22
1
1
= 2 22
1
1
1 22(1+)
= 1
22(1+)
2(1 + )1 22(1+)
22[1
] 1
Then,
1
() = ( ) ( )
=0 =0 =0
1
(+1)(1)[()(+1)1 (1)(+1)+ ]
2
×
1
! (22)+1[1 22(1+) ](( + 1) + 1)
2
(37)
() = 1 () , > 0 & 1
1
1
(38)
-
Renyi Entropy:Following is the Renyi entropy notation of the p.d.f. random variable x:
Proposition 7:
() = 1 [ () ) ()(+)
1 (
=0 =0 =0
(1)[()(+)+1 ( 1 )(+)+1]
× ]
1
! (22)+[1 22(1+)] (( + ) + 1)
Proof:
1 (1+)
(39)
The Renyi entropy of x, when x is a random variable has a TRPD.
1
() = 1 log [
22 (1 + )1
1
22
]
(40)
1
1 22(1+)
By integrating equation (40), get equation (39) by using the same steps in E(Xr).
-
Estimation Methods
There will be a discussion of several approaches for estimating TRLD's unknown parameters.
-
Maximum Likelihood Estimation.
Maximum likelihood estimation is employed to estimate the TRLDs unknown parameters. The p.d.f. the likelihood function is defined as follows:
1
(1+)
(, , , ) =
(22) =1(1 + )1
1
22
=1
1 22(1+)
The likelihood function with the Ln of both sides is taken.
1
= + (22) + ( 1) (1 + ) (1 + )
=1 22
=1
1
(1 22(1+) )
(41)
1
= + (1 + ) (1 + ) (1 + )
=1 22
=1
1 1 (1+)
(1 + ) (1 + ) 22
22
1
1 22(1+)
(42)
Now, derive the equation (3.43) with respect to , b and
1 1 (1+)
2 1 3 (1 + ) 22
= + (1 + )
3 1 (1+)
=1 1 22
(43)
1 1 (1+)
( 1) (1 + )1 2 (1 + ) 22
= =1 =1 2
(1 + ) 22 1 (1+)
=1 1 22
(44)
1 1 (1+ )
1 (1 + ) (1 + ) 2 2
+ (1 + ) (1 + ) (1 + ) 22
22 1 (1+ )
=1 =1 1 2 2
= 0
(45)
Lastly, set every one of these equations equal to 0.
=1
( 1)
=1
(1 + )1
1 1 (1+ )
2 1 (1 + ) 2 2
+ (1 + ) 3 = 0
3 1 (1+ )
=1 1 2 2
1
(46)
(1+ )
(1 + )
22
1
2 2
=1
(1 +
)
22
1
1 (1+ ) = 0 (47)
2
2
In order to determine the MLEs of the parameters , b and and, we solve equations (45) through (47) by using numerical
techniques.
-
Least Square Method (LS):
Following is a working definition of the method. It is well known that.
(( )) =
()
+1
Obtain the estimators by minimizing.
2
=1((()) +1)
1
1 22(1+)
[ ( 1 ) ]2 1 22(1+) + 1=1
(48)
SO
The could exist by deriving equation (48) concerning b.
1
1
2 1 2
1 (1+)
(1+) (1+)
(1 2 )( (1+) 2 )
2[ ( 1
2 2
) ] ×[
3
=1
1
1 (1+) 22
+1
(1
1 (1+)
22 )2
(1
1 (1+) 2 2
)(
1
3
(1 + )
1 (1+) 2 2
)
] = 0
1 1
1 (1+) (1 22(1+) )( 1 (1+) (1+) )
22
[ ( 1 2 2 ) ] ×[ 3
=1 1 (1+) +1 1 (1+)
1 22 (1 22 )2
1 (1+) 1 1 (1+)
(1 2 2 )( (1 + ) 2 2 )
3 ] = 0
1 (1+)
(1 2 2 )2
(49)
Then,
(1
1 (1+)
2 2 )2
The
could exist by deriving equation (48) concerning .
1 (1+ )
1 (1+ )
2
2[ ( 1
1 (1+ )
2
(1 22
) ] × [
)( (1+ )1 22 )
2
2
=1
1
1 (1+ ) 22
+1
1
1 (1+ )
(1 22 )2
1
(1
(1+ ))( (1 + )1
(1+ ))
22
22
1
22
] = 0
Then
(1 22(1+ ))2
1 (1+ ) 1 (1+ )
12(1+ ) (1 22 )( (1+ )1 22 )
2[ ( 1 2 ) ] × [ 2 2
=1 1 (1+ ) +1 1 (1+ )
1 22 (1 22 )2
1 1
(1 (1+ ))( (1 + )1 (1+ ))
22 22
22 ] = 0
1
(1 (1+ ))2
22
The can be found by deriving the equation (48) with respect to .
1 1
(50)
12(1+)
2(1+)
1
(1+)
2
2
2[ ( 1
2
) ] × [(1 2
) (
2
2
(1 + ) (1 + ) )
=1
1
1 (1+) 22
1
+1
1 (1+ )
(1 22
)2
1
22
(1
(1+) )( 1
22
2 2
(1 + ) ln (1 + )
1
(1+)
)
] = 0
1 1
12(1+) ( 1 2(1+) ) 1 ( 1 + ) (1 + ) 2(1+) )
[ (1 2 ) ] × [ 2 ( 2
22
=1 1
(1+) +1 1 (1+ )
1 22 (1 22 )2
1 1
(1 (1+) )( 1 (1 + ) l (1 + ) (1+))
22 2 2
22 ] = 0
1
(1 (1+ ))2
22
(51)
Then,
22
(1
(1+ ) )2
Equations (49) through (51) have numerical solutions.
-
-
Application
Data set 1: have data set represents the lifetime of 51 devices [23] the data set is 0.1,0.2,1,1,1,1,1,2,3,6,7,1,1,12,18,18,18,18,18,21,32,36,40,45,46,47,50,55,
60,63,63,67,67,67,67,72,75,75,79,82,82,84,84,84,85,85,85,85,85,86,86.
= 2 + 2 , |
(52) |
Then compares the proposed method to the truncated Rayleigh Lomax distribution, the RLD, the LD, and the RPD, and it applies the MLE method to the dataset (TRPD). These metrics (CAIC, AIC, BIC, and HQIC) are used to make the model selection.
= 2 + (), |
(53) |
= 2 + 2(log()) |
(54) |
= 2 + 2 1 |
(55) |
Table (1) displays the model-level estimates (MLEs) for the data models, while Table (2) provides numerical amount for the
model-chosen statistical (AIC, , CAIC, BIC, and HQIC). Based on Table (2), the (TRLD) model provides the smallest
representation of the data set due to its small amount for the criteria CAIC, BIC, AIC, and HQIC.
Table 1. Data-based estimates of various parameters.
Models |
Parameters Estimation |
||
TRPD(x;,q,w) |
=0.66 |
=20.247 |
=10.959 |
R-LD(x;,,b) |
=0.844 |
=12.7726 |
=9.738 |
LD(x;,) |
=1.08 |
=20.744 |
|
R-PD(x;p,b,) |
=0.172 |
=0.627 |
=0.419 |
TRLD(x;,b) |
= 0.859 |
= 2.987 |
= 5.617 |
Models |
AIC |
BIC |
HQIC |
CAIC |
|
TRPD(x;,b,p) |
-217.7661 |
441.5321 |
447.2682 |
443.7164 |
442.0588 |
R-LD(x;,,b) |
-238.5086 |
495.0171 |
504.7532 |
497.2494 |
495.5388 |
LD(x;,) |
-248.2744 |
502.5487 |
508.2848 |
504.7330 |
503.0704 |
R-PD(x;p,b,) |
-287.7194 |
581.4387 |
587.1748 |
583.6230 |
581.9604 |
TRLD(x;,,b) |
-239.2239 |
484.4478 |
490.1839 |
486.6321 |
484.9695 |
Table 2. , CAIC, BIC, AIC, and HQIC Data Set statistics values.
By comparing the results in Tables (1) and (2), we conclude that the truncated Rayleigh-Pareto distribution is preferable to Rayleigh-Lomax distribution Lomax distributions, truncated Rayleigh-Lomax distributions, and Rayleigh-Pareto distributions. This is because the (TRPD) and (TRLD) distributions have the smallest values for the (BIC), (AIC), (HQIC), and (CAIC) compared to the other distributions.
-
CONCLUSIONS
The current study presents a truncated Rayleigh – Pareto distribution (TRPD) for three parameters. In addition to the stress strength reliability pdf and cdf, distribution pdf and cdf are also located. Mod, the moment generating function, the median, the quintile, the mean and variance, the moments, the geometric mean, the harmonic mean, and the order statistics were among the data we retrieved. Essential estimating techniques were also used. It is observed that the (TRPD) was preferable to other distributions we considered.
-
FUTURE WORK The current research suggests the following future works:
-
Split the amputated period into (n) of the periods.
-
Comparison of estimation methods.
-
Use other new methods to estimate parameters.
-
Find the parameters for the (TRPD) by numerical methods
-
Find new distributions by truction.
REFERENCES
[1]. Wen-liany Hung and ching- yichen. (2004). Approximmate MLE of the scale parameter of the truncated Rayleigh distribution under the first censored data Department of Statistics Tamkang University Tamsui, Taipei Taiwan, 25137 ROC Department of Mathematics Education National Hsinchu Teachers College Hsin-Chu Taiwan ROC. [2]. David R-clark, FCAS. (2013). A note on the upper- truncated Pareto distribution, Presented at the. 2013 Enterprise Risk Management SymposiumApril 22-24, 2013. [3]. Taylor and francis. (2014). Parameter Estimation for the Truncated Pareto Distribution, Informa Ltd Registered in England and Wales Registered Number: 1072954 Registered office: Mortimer House, 37-41 Mortimer Street, London W1T 3JH, UK [4]. Mathias Reachke. (2012). Inference for the truncated exponential distribution, Published online: 5 February 2011. [5].AL_ Yasseri , Ahmad Yassin Taqi. (2014). Estimation of parameter and Reliability function for truncated Logistic distribution , M.CS. Thesis. AL- Mustansiriya University. [6].Dibagay, Dler Mustafa. (2007). Numerical Estimation of the Parameters of the Gamma and Truncated Exponential Distribution, M.Sc. Thesis, University of Mosul. [7].Hormuz. Amir Hanna. (1990). Mathematical Statistics,Directorate of Dar Al-Kutub for Printing and Publishing, University of Mosul. [8] Blumenthal & Goel. (1988). Estimation With Truncated Data OHIO State Univresity For columus VOL.4 [9]Al_ Sultani ,Bnadheer Dheaa Mohmmad. (2017). New Distribution on Proposed Classes M.cs. Thesis. University of Babylon. [10].Hassan, Habe Ali. (2015). Issues related with uniform distribution, M.CS. Thesis. AL-Mustansiriya University. [11]AL_Ghalib ,Sabah Muhammad Khudair. (2019). On some Special oge distributions , M.CS. Thesis. University of Pabylon. [12].AL- Sultani , BD. (2017). New Distributions on proposed classes, M.Sc.Thesis, University of Babylon. [13].Hanaa H. Abu Zinadah. (2014). Six Method of Estimations for the Shape Parameter of Exponentiated Gompertz Distribution Department of Statistics, sciences Faculty for Girls King Abaulaziz University, PO BOX 3269 Jededah 21436, Saudi Arabia