
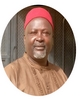
- Open Access
- Authors : M. Laisin, U. O. Chineh
- Paper ID : IJERTV12IS050295
- Volume & Issue : Volume 12, Issue 05 (May 2023)
- Published (First Online): 19-06-2023
- ISSN (Online) : 2278-0181
- Publisher Name : IJERT
- License:
This work is licensed under a Creative Commons Attribution 4.0 International License
Uncertainties of Workforce and End Inventories in Aggregate Production Planning using Imprecise Repair Times
-
Laisin & U. O. Chineh
Department of Mathematics Chukwuemeka Odumegwu Ojukwu University, Uli
Abstract – The focus of Aggregate Production Planning (APP), a type of medium-term planning, is on the most economical way to manage production in order to meet fluctuating demand and other uncertainties like production loss as it affects productivity. The proposed method deals on checking the impact of production loss on inventory and labor levels, overtime, backordering levels, workforce, machine, and warehouse capacity in an effort to reduce total costs and repair cost and maximize income. The study results were obtained using Lingo version 18 software at the very end using data gathered from Rich Pharmaceuticals Limited (RPL). Four different scenarios were thought of for the suggested model, each of which produced a useful compromise solution and varying degrees of DM satisfaction with the various fuzzy objective values. Thanks to the suggested model's systematic structure for facilitating decision-making, a decision maker can interactively change the fuzzy data and associated model parameters until a satisfying answer is attained in the quest to reduce production loss.
Key Words: Production Loss, Workforce, goal programing, Decision maker, linear membership function
-
INTRODUCTION
Aggregate planning strategies assist managers in selecting between production smoothing options (where inventory is produced during low-demand times and/or backlogs are accumulated during high-demand periods) and so-called chasing alternatives (involving matching production output according to demand). Aggregate Production Planning (APP) determines the best way to meet forecasted demand in the intermediate future, typically from 6 to 24 months in advance, by adjusting regular and overtime production rates, inventory levels, labor levels, subcontracting and backordering rates, and other controllable variables (Wang R. et al., 2005). The primary inputs of APP are market demands and the manufacturing plan to meet those expectations. (Leung et al., 2003). Production planning does this in response to changes in demand. The cost and uncertainty of abruptly altering a company's production schedule might be high. Changes in production schedules can be made with minimum effort if demand changes are anticipated months in advance (Hossain M. et al., 2016). Another crucial aspect is to assess the profitability of the plan in order to deliver maximum yield and determine how to address any productivity loss by carefully examining every part of the implemented plan (Piper & Vachon, 2001). The majority of people are not motivated to address the problem of productivity loss, despite the importance of this evaluation step (Filho et. al., 2010).
Many aggregate planning issues do not properly take into account productivity losses brought on by workforce layoffs and hiring. The productivity losses linked to other capacity changes, such backorders, multiple shifts, and overtime, are also largely unmentioned in parts of the research. When productivity losses are taken into account, traditional methodologies impute corresponding costs but do not take lost productivity into account. It has been discovered that when capacity restrictions in linear programming formulations explicitly account for productivity losses, the resultant production plans are better than those that are produced when productivity losses are only treated as costs in the objective function.
This research proposes a novel multi-product, multi-period, multi-objective APP problem. Multi-objective mathematical programming is used to represent the given issue. Three objective functionsminimizing total cost, minimizing repair cost and maximizing sales revenueare taken into account simultaneously. Numerous restrictions are also taken into account, including those relating to the amount of production, the amount of time available, the number of workers, the amount of inventory, the number of backorders, the capacity of the machines, the amount of warehousing space, and the available budget. The proposed model is then solved using fuzzy goal programming (FGP). The outcomes of the suggested strategy are contrasted with those of the company's current experimental method.
-
LITERATURE OF PAST WORKS
-
The literature of earlier studies was reviewed based on two categories. In the first category, the APP and its variants are examined. The second category examines the goal programming approach. Three main categories have been used to assess the APP literature:
1) The conventional APP models, which consider planning horizons; 2) APP models that account for uncertainty; and 3) multi- objective APP models applied to address real-world industrial problems.
The Classic Aggregate Production Planning
Broadly speaking, one of the main categories for production planning is APP (Giannoccaro and Pontrandolfo, 2001; Mula et al., 2006). Since the traditional model of linear decision rule for production and employment scheduling proposed by Holt et al. (1955, 1961), many scholars have thoroughly investigated the APP problem (Leung and Wu, 2004; Wang and Liang, 2004; Jain and Palekar, 2005). APP is one of the most crucial tasks in production and operations management, claim Wang and Liang (2004). In their study of APP models, Nam and Logendran (1992) grouped them into groups of optimal and nearly-optimal models. A review of mathematical optimization models, including the APP, revealed that linear programming is the most extensively used strategy and has become a standard method. The APP approach was defined as medium-term capacity planning with a planning horizon of 218 months by Baykasoglu (2001). APP was defined as a strategy by Fung et al. (2003) to establish the production, inventory, and labor levels necessary to meet all market demands. The works on production planning and management for remanufacturing were reviewed by Junior and Filho (2012). A competitive version of the APP model with capacity restrictions was discussed by Karmarkar and Rajaram in 2012. Systems with several periods, many products, and multiple machines with setup results were the main emphasis of Ramezanian et al. (2012). A mixed integer linear programming model for the APP problem with capacity augmentation in the production system was presented by Zhang et al. (2012).
To model and simulate the APP problem, Jamalnia and Feili (2013) proposed a hybrid system dynamics and discrete event simulation methodology. Their study's main goal was to evaluate the efficiency of APP techniques in terms of Total Profit. In a mixed model production context, Tonelli et al. (2013) developed an optimization strategy to deal with aggregate planning issues. Furthermore, real-world problems can be handled by APP models, and they are frequently resolved by efficient algorithms. The APP cost function has been observed to be convex and piecewise in numerous investigations (Bushuev 2014). Khoshnevis et al, (1982) centered on APP methodologies that mainly focused on the productivity loss caused by APP problem with fixed and variable workforce. Also majority are not inspired to address the problem of productivity loss, despite the importance of this evaluation phase (Filho et. al., 2010).
The Fuzzy Aggregate Production Planning
The Fuzzy Set (FS) theory was first put forth by Zadeh (1965). It is based on an extension of the conventional concept of set A, according to which each element x of a certain uiverse X either belongs to set A or it does not. However, according to the FS theory, an element can only have a specific "degree of membership" in set A. There's no denying that some fuzzy programming problems are outside the capabilities of standard mathematical programming techniques. Zimmermann (1976) was the first to use fuzzy set theory with conventional LP issues.
His research focused on LP problems with fuzzy goal and constraints. With the use of Bellman and Zadeh's (1970) fuzzy decision- making strategy, the same investigation confirmed the existence of a comparable single-goal LP problem. Fuzzy mathematics programming has since evolved into a number of fuzzy optimization techniques for resolving APP problems. At the moment, fuzzy methods are frequently effective for making decisions. Fuzzy techniques have been employed in virtually all decision-making processes, including multi-objective, multi-person, and multi-stage decision-making (Tamiz,1996). Other research on fuzzy decision making also includes applications of fuzzy theory in management, business, and operational research (Zimmermann, 1991).
The fuzzy multi-product aggregate production planning (FMAPP) model was developed by Fung et al. (2003) to address a variety of situations with varying decision-making preferences. It uses integrated parametric programming, best balancing, and interactive techniques. This methodology can also successfully increase an aggregate plan's ability to generate workable disaggregate plans in a variety of situations with fuzzy requests and fuzzy capacities. Wang and Liang (2004a) more recently developed a fuzzy multi- objective linear programming model using the piecewise linear membership function to address multi-product APP choice issues in a fuzzy environment. The model can produce a workable compromise solution and the overall satisfaction levels of the decision maker. Leung and Chan (2009) developed a preemptive goal programming strategy for the APP problem in order to maximize profit, reduce repair expenses, and increase machine utilization.
Multi-Objective Aggregate Production Planning
Wang and Liang (2004) created a Fuzzy Multi-Objective Linear Programming (FMOLP) model to resolve the multi-product APP decision issue in a fuzzy setting. The proposed model attempts to lower total production costs, carrying and backordering costs, and rates of changes in labor levels by accounting for inventory level, labor level, capacity, warehouse space, and the time value of money. And more recently, Boppana and Slomp (2002) used a mathematical programming model with objectives to resolve this problem in a company of machines and tools. Both Wang and Fang (2003) and Dai et al. (2003) present a technique that utilizes fuzzy linear programming. Mirzapour Al-e-Hashem et al. (2011) included the expenses connected to the supply chain and demand as additional unknown elements. Ghasemy Yaghin et al. (2012) proposed a fuzzy multi-objective APP model with both qualitative and quantitative objectives for a two-level supply chain.
Fuzzy Goal Programming
When there are multiple objectives and goals that conflict, decision-makers and production managers must make difficult decisions about which objectives and goals should take precedence. The goal programming (GP) method may be suggested as a workable and useful solution to address this problem. Fuzzy set theory has been introduced to cope with the ambiguous character of real- world situations, which has led to the expansion of several decision-making techniques in fuzzy contexts. The fuzzy goal programming (FGP) technique, in particular, has been used to handle a number of multi-objective production planning problems. Lee et al. (2009) and Kumar et al. (2004) introduced FGP approaches for supplier selection problems with a variety of objectives. According to Liang (2006), a FGP approach may be used to solve integrated production and distribution planning issues with fuzzy multiple objectives in ambiguous situations.
According to the literature cited above, a gap has been found in prior works. An APP problem with multiple objectives, multiple periods, and multiple products is proposed in this paper. A FGP is the recommended fix for the issue. Maximizing sales revenue, minimizing overall production costs, and minimizing repairing cost are all essential components of the situation at hand. Therefore, it makes more sense to categorize them as three separate goals so that the APP model may find a Pareto optimum that achieves a balance between these three aims. The following three-objective, multi-period, multi-product FGP-APP model is developed.
-
Method and Procedure
Majorly there are about Five factors which individually may contribute to Production Loss within a production process; Production Loss Due to Man Power, Breakdowns, Delay in Material Supply, Process Time (the most impactful.) and Buffing Time. A crucial step of this APP involves determining the profitability of the plan in order to deliver maximum yield and determine how to address any productivity loss by carefully examining every component of the implemented plan (Piper & Vachon, 2001). Producers are forced by the intensifying competition on the global market to manage their operations properly and efficiently. The letter is one of the key ingredients for attaining this.
Four possible scenarios are considered, centering on workforce and Production loss with respect to Process Time.
-
Scenario One: Dynamic workforce with backorders not considering Production loss
-
Scenario Two: Dynamic workforce with backorders and considering Production loss
-
Scenario Three: Static workforce without backorders and not considering Production loss
-
Scenario Four: Static workforce without backorders and considering Production loss
-
Assumptions and Problem Definition
Following the findings of a real-world case study, the following presumptions are made for the mathematical model of the suggested APP problem.
-
Production planning is done in a time horizon of T time periods ( = 1,2, , ).
-
There is a Batch production system capable of producing all kinds of types of products.
-
Market demand can be fulfilled or backordered, however no backorder in the last is allowed.
-
There are two working shifts; Regular time production and Over time production
-
A warehouse is allowed for holding final products.
-
In advance, the holding cost of inventories are determined and well known.
-
The workforce accommodates various skill levels ( ).
-
Workers salary is independent of unit production cost.
-
At each period T, Production quantity is considered more of the safety stock for finished products.
-
Hiring and firing of Manpower based on product demand is eligible and there is an allowable limit.
-
In each period T, the shortage of production is recovered by overtime production in each shift.
-
In each period T, the nominal and actual capacity of production machines is not the same due to unforeseen failures. So, the actual capacity of production is usually reduced by a fixed failure percentage.
-
If an unforeseen failure occurs during a shift the repair process is completed in the next. This may stop, reduce, or decrease the production rate during maintenance actions. No repair during overtime.
-
The impreciseness and uncertainty of real-world problem and confliction of different objectives are modeled using fuzzy goals.
-
Linear membership functions are defined for fuzzy goals.
-
FGP used to solve the problem.
-
-
Parameters, Indices, Decision Variables and Notations
They are as stated in Tables 1 to 3
Table 1: Set of indices
Number of periods in the planning horizn; = 1,2, ,
Number of product types; = 1,2, ,
Raw material type; = 1,2, ,
Types of shifts; 1,2
Types of warehouse; = 1,2, ,
Skill levels of workers; = 1,2, ,
Number of objective Functions; = 1,2,3
Table 2: Notation for parameters
Parameter
Definition
Cost of Production; for product in shift
Demand of product in period
Cost of Backordering; for product in period
Sales Revenue for product (/unit)
Process time of product in period
The Budget upper limit in period
AsP
Allowable shortage of product in period
Available Maximum workforce in period
Available Minimum workforce in period
workforce that are available for overtime (in percentage)
Cost of workforce of level k in period
Cost of Hiring workforce of level k in period
Cost of firing workforce of level k in period
Holding cost for raw material type in period in warehouse
Holding cost of unit of product in period
Cost of Finished product Defect
fraction of the workforce variation in period
Machine hours needed to produce unit of product in period
Machine capacity that is lost due to interruption in period (in percentage)
Machine capacity that is lost due to repairs in period (in percentage)
The maximum of machine capacity that is available in shift in period
The machine capacity that is available for overtime (in percentage)
Available Regular time in both shifts in period
The units of type raw material required to produce unit of product
product safety stock
Raw material type safety stock
The maximum available space of warehouse w
The capacity of warehouse for storage of raw-material type in period
The capacity of warehouse for storage of finished-product in period
The Due date of product
Batch size of product
Finished product Defect rate
Production Loss
Table 3. Decision variable Notation
Decision variable
Definition
Number of product i produced in shift q of period t
Number batches of product i produced in shift q of period t
Backorder level of product i in period t
Number of available workers of level k in period t
Number of hired workers of level k in period t
Number of fired workers of level k in period t
Inventory level of raw material type m at the end of period t in warehouse w
Inventory level of finished-product i in period t in warehouse w
-
Model Formulation
-
The Dynamic workforce Model
Hiring and firing of workers are allowed and Backorders are also allowed.
1 = + + +
=1 {1,2} =1
=1 =1
=1 =1
=1 =1
()
+ + +
=1 =1 =1
=1 =1 =1
=1 =1
The above Minimization of Total Cost function (TFC) involves the following seven terms; the per unit Production Cost, Cost of salary of the workforce, Cost of hiring, Cost of firing, Cost of holding of products, Cost of holding of raw materials, and Cost of Backordering.
Minimize Repair Cost
2 = ×
=1 {1,2} =1
(2)
The objective function (2) considers the quality of products. Defect rates differ slightly across products. Managements sets
acceptable amount that the company is willing to pay in each period for repair costs.
Maximize Sales Revenue
This last objective function is to realize the highest possible return from the quantities produced by regular production and overtime production including inventories and back orders.
3 = × (1 1 + + )
=1 {1,2} =1
(3)
Constraints
The Labor-force Constraints are considered as follows:
,
=1
(4)
,
=1
(5)
= (1) + ,
, , > 1
(6)
(1) ,
, , > 1
(7)
Constraints (4) attests that the total labor utilized during period t does not exceed the total workforce that is available. In a similar vein, (5) guarantees that in period t, the employed workforce exceeds the available minimum workforce. Set of Constraints (6) is a workforce level balance equation that assures that the workforce with skill level k available during a given period is equal to the workforce with the same skill level k during the previous period plus the change in workforce level during the current period. The change in workforce level in each planning period cannot be greater than a benchmark number of workers in the present period, according to constraint number seven.
Time Constraints
, , = 1 (8)
=1
=1
, , = 2 (9)
=1
=1
The relationships mentioned above make sure that each working shift's necessary production time is less than or equal to the
available regular production time and overtime. Inventory Constraints
= (1) + , , , > 1 (10)
{1,2}
= (1) + (1) , , , > 1 (11)
{1,2}
, , , (12)
Constraints (10) ensures that the amount of finished product type in period in warehouse is equal to the amount of finished product type inperiod 1 in warehouse w plus the quantity of produced finished goods type I in period t in both working shifts, less the amount of product type in period that is on backorder and the quantity of produced finished goods type I in period t in
both working shifts. A set of limitations (11) assures that there is a balance between raw materials, and (12) guarantees that the
safety stock of raw materials in warehouses is satisfied. Production Constraint
, , , (13)
{1,2}
(1
) + (1), , , > 1 (14)
{1,2}
Set of constraints (13), which is written for all product types and all periods of planning, guarantee the satisfaction of safety stock
of finished-products in working shifts. Set of constraints (14) represents the total production of non-defected final products plus the inventory of finished-product in previous period should be greater than or equal to demand of the finished-product in current period.
Machine capacity Constraints
, , = 1 (15)
=1
, , = 2 (16)
=1
Constraints (15) and (16) pledge that in regular time and overtime, the machine capacity is assured.
Warehouse Capacity Constraint
, , , (17)
=1
=1
, , (18)
=1. =1
=1 =1
+ , , , (19)
=1
=1
The first two constraints (17) and (18) gives the restrictions of actual inventories of finished products and raw materials. While (19)
guarantees that each warehouse at each period will not be able to allow storage capacity of products an raw materials beyond its maximum warehouse available space.
Backorder, Budget limit and Non-negativity Constraints There is backorder obeying the following;
, (20)
=1
=1
= 0, (21)
=1
(22)
, , , , 0, , , , , (23)
, , 0, , , (24)
Constraints (20) represent the backorder level at the end of period t cannot exceed the certain percent-age of the demand which
determines the upper limit of shortage. While (21) assure that there is no possibility for backordering at the end of time horizon or last period.
A restriction on the available budget for each planning period is shown using (22), which ensures that the Total Cost (i.e., Eq. (1)) cannot go beyond the predetermined budget for the time horizon.
(23) and (24) both present non-negativity requirements on decision variables.
-
The Static workforce Model
Hiring and firing of workers are not allowed and Backorders are not allowed.
Minimize Total Cost
1 = + +
=1 {1,2} =1
=1 =1
=1 =1 =1
()
+
=1 =1 =1
The above Minimization of Total Cost function (TFC) involves the following seven terms; the per unit Production Cost, Cost of
salary of the workforce, Cost of holding of products, Cost of holding of raw materials.
Minimize Repair Cost
2 = ×
=1 {1,2} =1
(26)
The objective function (2) considers the quality of products. Defect rates differ slightly across products. Managements sets
acceptable amount that the company is willing to pay in each period for repair costs.
Maximize Sales Revenue
This last objective function is to realize the highest possible return from the quantities produced by regular production and overtime production including inventories and back orders.
3 = × (1 1 + + )
=1 {1,2} =1
(27)
Constraints
By eliminating (20) and (21),the current model will have similar constraints as the previous model except changes in Constraint (6) which gives;
= (1) , , > 1 (28)
And then the non-negativity requirements on decision variables.
, , , 0, , , , , (29)
-
The Dynamic and Static workforce Model Considered under Productivity Loss
The two models share similarities but only differ mainly in changes in workforce constraints. One of the major areas of similarity
is on the Process Time constraints on which the parameter of Productivity Loss (1 ) will be imposed. The Time constraints with Production Loss incorporated gives:
( MTTR ) , , = 1 (30)
=1
=1
( MTTR ) , , = 2 (31)
=1
=1
From (30) and (31), MTTR is a statistical measure frequently used to estimate how swiftly a system is fixed and brought back
into production. the MTTR can be determined by:
MTTR = , + , + , (32)
Where , is time to run the diagnosis, , is the time to run the calibration routine and , represents the setup time which also
includes the time to pick up the spare parts.
-
-
Fuzzy Multi-objective Goal Programing Development
In classic models of GP, the decision maker has to specify a precise aspiration level (goal) for each of the objectives. In general, especially in large-scale problems, this is a very difficult task, and the use of the Fuzzy Set theory in GP models can overcome such problem, allowing decision makers to work with imprecise aspiration levels (Yaghoobi and Tamiz, 2007). In multiobjective
programming, In fuzzifying the inequality signs; = and , Zimmermann (1978) used the symbol ~, they are to be
understood as essentially greater than or equal to and essentially less than or equal to. if an imprecise aspiration level is
introduced to each of the objective functions then these fuzzy objectives are termed as fuzzy goals. Let be the aspiration level assigned to the kth objective (). Then the fuzzy goals are:
() [for maximizing ()] and
() [for minimizing ()]
In solving the problem, a general form of FGP model is considered:
find
to satisfy;
subjet to
()
()
(=)
0
= 1
= + 1 (33)
For this paper, a FGP is employed in solving any of the APP models, like (1) (24). Being able to use FGP approach with fuzzy
goals, the aspiration levels should be calculated. Payoff table is used when the decision maker has no enough view point to determine the aspiration levels. Zimmermann (1978) used a Payoff table to develop an upper and lower limit that was used to formulate the membership functions of the fuzzy goals.
In the general form (33), the purpose of FGP is to find compromise solution such that all fuzzy goals are satisfied. is the aspiration level for kth goal, are system constraints in vector notation. () Means that the kth fuzzy goal is approximately less than or equal to the aspiration level , and () Means that the kth fuzzy goal is approximately greater than or equal to the aspiration level (Hannan, 1981).
The fuzzy decision-making concept of Bellman and Zadeh (1970) can be used to solve the planned multi-objective APP problem (1)(24). Linear membership functions as proposed by Zimmermann (1978) are used to represent the fuzzy goals of decision makers.
Now, the membership function for the kth fuzzy goal () can be expressed as follows:
1 ()
(()) =
()
()
(34)
{0 ()
where is the upper tolerance limit for the kth fuzzy goal and is the tolerance which is subjectively chosen and the
function is as depicted in Figure 1a.
Again, the membership function for the kth fuzzy goal () can be expressed as follows:
1 ()
(()) =
()
()
(35)
{ 0 ()
where is the lower tolerance limit for the kth fuzzy goal and is the tolerance which is subjectively chosen and the
function is as depicted in Figure 1b.
(a) (b)
Figure 1: Linear Membership form
Hence, the associated FGP model for the multiobjective APP problem (1)-(24) with (34) and (35) is formulate as follows:
find
to satisfy;
()
(1()) =
()
(2()) =
()
(3()) =
( ()) [0,1], = 1,2,3
(4) (24)
0, = 1 . .
This suggested approach states that goal weights are decided by DM, and goal aspiration levels are derived using a payout table.
The positive ideal solutions (PIS) and negative ideal solutions (NIS) of the objective functions can be respectively specied as follows, (Hwang and Yoon,1981; Lai and Hwang, 1992b);
1
2
3
1
= 1;
2
= 2;
3
= 3;
= {1()}
= {2()}
= {3()}
Where is the positive ideal solution of objective function .
-
-
-
Application
A business case study
Data description
To demonstrate the effectiveness of the recommended technique, the case study of Rich Pharmaceuticals Limited (RPL) was used. RPL is one of Nigeria's top pharmaceutical manufacturers. The Southern and Middle Belts of Nigeria, as well as other regions of West and East Africa, are where RPL's products are mostly sold, and there has recently been increasing demand for them. RPL's business APP approach is to keep a stable labor force level over the planning horizon, allowing for the flexible meeting of demand through the use of inventories, overtime, backorders and determine possible cases of production loss. Due to the shortcomings of the old method, in which evaluation comparisons are only available for specific plans under specified conditions and indication for the optimal plan is ambiguous, RPL has been unable to reach the performance initially predicted.
Alternately, the DM can produce an aggregate production schedule for the RPL factory using a mathematical programming method. The planning horizon covers a period of six months, from May to October, according to business reports. Two different standard product kinds are part of the concept. The average payroll for each time is 64. The daily costs for hiring and firing workers are
30 and 40, respectively. Production expenses for overtime are capped at 30% of production expenses for regular hours. Additionally, it is assumed that each product has no beginning inventory and no backorders at the last period. The inventory's
maximum allowed storage area is 30003. In a day, there are two working shifts. 8 hours are allotted for regular production per
shift, while 3 hours allotted for overtime production. To produce these products, 10 types of raw materials are required. When
demand for a certain period exceeds production capacity during regular hours and inventory levels are likewise insufficient to meet this demand, production is continued during overtime.
The APP decision issue for the industrial example that is presented here focuses on developing several fuzzy goal programming models for determining the optimal approach to adjust output rates, hiring and firing, inventory levels, overtime, backorders and production loss in order to meet predicted demand. Minimizing overall production costs, repair expenses, and maximizing sales are the expected results of this APP choice.
Table 4. Demand and Constraint Parameters
Product |
Period |
Forecasted Demand |
MTTR / |
1 |
1 |
252 |
0 |
2 |
295 |
1.2 |
|
3 |
430 |
0 |
|
4 |
260 |
1.5 |
|
5 |
300 |
0.5 |
|
6 |
270 |
0.2 |
|
2 |
1 |
255 |
1 |
2 |
284 |
0 |
|
3 |
430 |
0 |
|
4 |
260 |
1.2 |
|
5 |
300 |
0 |
|
6 |
270 |
0.3 |
-
Computational Results
The recommended APP models are programmed and executed separately utilizing the LINGO 18 solution. The four possible scenarios are considered, centering on workforce and Production loss with respect to Process Time to check their impacts on the
stated three objectives 1, 2 and 3.
Table 5: Payoff Matrix
()
Objectives
PIS
NIS
Scenario One
Min 1()
1319339
1602043
1683754
1683754
Min 2()
22056.04
21636.11
25428
25428
Max 3()
2141834
1367429
1637639
1367429
Scenario Two
Min 1()
1326511
1609136
1640441
1640441
Min 2()
22056.04
21636.11
25428
25428
Max 3()
1993114
1373746
1993114
1373746
Scenario Three
Min 1()
1315376
1385800
1511459
1511459
Min 2()
21636.04
21636.11
24784.78
24784.78
Max 3()
2074220
1367429
1541053
1367429
Scenario Four
Min 1()
1323137
1407213
1475007
1475007
Min 2()
21636.04
21636.11
25116
25116
Max 3()
1950451
1373746
1580130
1373746
From Table 5, it can easily be seen that there are significant changes on the various results of the objective values () when comparing the various Scenarios. The Total cost of production in Scenario One is 1() = 1319339 but the value increases to
1() = 1326511 in Two due to Production loss, all within a dynamic workforce model. In Scenario Two, there is a decrease on the revenue 3() = 1993114 as against 3() = 2141834 in Scenario One. Same can also be deduced between scenarios three
and four that considers the APP within a static workfoce. A comparative look on the Total cost of production between Scenario
Two and Four shows a higher production cost in Scenario Two 1() = 1326511 as against that of Four 1() = 1323137, both considering Production Loss but with different workforce parameters, which is attributed to the hiring and firing of workers that is
allowed in Scenario Two. As observed from the table also the repair cost 2() =22056.04 in Scenario Two is higher to that of Scenario Four 2() = 21636.04, as a result of no backorders in the later.
As already said, production quantity, inventory, and workforce are the three main considerations in an entire APP program. From Figure 2 below, the MTTR at each period affected the level of production in that particular period, both in its regular and over time.
In period two where the = 1.2, there is a production loss as indicated in Scenario Two and Four. The Overtime production
is to make up any unmet demand based on customer satisfaction. Clearly production loss affects the major outputs of an APP, it
affects the inventory level, the produced quantity at regular and overtime and also the workforce level. As expected, the quantity produced during regular production reduced slightly when compared to other scenarios without production loss, this results in an increase in production during the overtime as indicated in Figure 2. This increase in production during the overtime was also affected because there is no backorder in the scenario being discussed. Production loss also affects the level of inventory in any period,
though there will be reduction on the cost of inventory but it will still call for increase in production in the next period + 1.
450
400
350
300
250
200
150
100
50
0
Regular Time Production
X111 X112 X113 X114
Scenario One Scenario Two Scenario Three
X115
Scenario Four
X116
Demand
Overtime Production
95
85
75
65
55
45
35
25
15
5
-5
X121
X122
X123
X124
X125
X126
36
31
26
21
16
11
6
1
-4
Product Inventory
Scenario One
Scenario Two
Scenario Three
Scenario Four
XP11 XP12 XP13 XP14 XP15 XP16
Figure 2: Impact of Production Loss on the Produced quantity and on Inventory
-
Added Analysis
The PIS and NIS of each objective function are used to establish the linear membership function for each objective function for any particular Scenario, as well as to express the equivalence of these objective values as a membership value in the range [0, 1]. The appropriate fuzzy linear membership functions may be defined as follows using Eqs. 34 and 35 for Scenario One
1 1() 1319339
(1()) =
1683754 1()
1683754 1319339
1319339 1() 1683754
1
(1())
1()
{ 0 1() 1683754
1 2() 22056.04
0 1319339 1683754
1
(2()) =
25428 2()
25428 22056.04
22056.04 2() 25428
(2())
2()
{ 0 2() 25428
1 () 2141834
0 22056.04 25428
1
(3()) =
3() 1367429
2141834 1367429
1638420 () 2141834
(3())
3()
{ 0 () 1367429
0 1367429 2141834
The data in Table 5 may be utilized to come to the conclusion that even in the presence of several conflicting goal functions and
restrictions, the recommended FGP is capable of finding a high-quality compromise solution. It is clear that all objective functions have a high level of satisfaction, and this is seen as a decent Compromising solution to the issue.
Table 6: The fuzzy goal programming
Objective values |
|
1 2 3 |
|
1447327 23240.33 1364486 |
0.6487841 |
1429867 23177.08 1299021 |
0.6675418 |
1480808 25118.88 1446717 |
0.1121807 |
1458873 24746.30 1435014 |
0.1062375 |
The proposed model provides the overall levels of DM satisfaction ( value), taking into account the multiple fuzzy goal values
(1, 2 3). If the response = 1, then every objective is fully met. None of the objectives are achieved if = 0. If 0 <
< 1, all of the objectives are partially met. In Scenario one (1 = 1447327, 2 = 23240.33, and 3 = 1364486), for example, the initial calculation of the total DM satisfaction () given the objective values was 0.6487841. If the DM does not accept the initial overall degree of this satisfaction value, the value might be changed to seek for a set of superior compromise possibilities.
Productivity losses are typically experienced while using the many chasing techniques used in aggregation planning (such as
backorders, working overtime, managing several shifts, and hiring and firing) where they are cited as costs to be considered. This demonstrates that explicit productivity losses should be included in collective planning models with more care- when seen as a positive productivity impact. It has been demonstrated that an explicit productivity-loss strategy results in significantly smoother,
lower cost production plans, which should be of interest to production planners on a practical level as can be observed in 1 = 1480808 of Scenario Three and 1 = 1458873 of Scenario Four.
The hiring process is viewed as a source of productivity losses in some APP models. It is commonly acknowledged that new hires
take some time to adjust and attain the same level of productivity as experienced employees. It is also acknowledged that before being completely prepared for shop-floor work, new employees regularly participate in non-productive but essential tasks like training sessions and medical exams. These productivity loss causes are well known and also reflects on the Production cost as
found between 1 = 1447327 of Scenario One and 1 = 1429867 of Scenario Two.
5. CONCLUSION
The productivity loss that results from various loss of production is difficult to assess, and many of the studies that are frequently used to do so have come under fire from specialists in the field. Low productivity means that resources aren't making the most of their abilities and talents, which drives up the cost of hiring new employees for the organization. The DM can choose a preferred production plan with a common satisfaction level or different combinations of possibility and satisfaction levels using the suggested models and approaches, depending on the market demands and available production capacities that meets their top priorities having production loss in mind.
Virtually all of the mathematical APP models so far employed capacity limitations and assumed the same productivity levels for regular time, overtime, and multiple-shift work, in addition to not necessarily stating the detrimental productivity impact of each of the chasing techniques. The capacity losses brought on by hiring and layoffs were improperly taken into account by the models. It seems that wen productivity losses were taken into account, it was presumed that the objective function was sufficient to account for their economic impact. As long as the productivity losses are minimal or the expenses are high enough to rule out the chase choices, this strategy should provide workable production plans.
There is no dispute that productivity losses exist, but additional study is needed to determine the specific types and magnitude of production losses linked to chasing techniques. To enable production planners to more completely include these losses in their overall plans, procedures for measuring these losses should be developed. It is possible to do more study to identify the causes of these productivity losses.
Future studies will take into account the fuzzification of the MTTR input parameter and also determines the impact of production loss on other parameters like overtime, raw materials etc.
REFERENCES
[1] Baykasoglu, A. (2001), MOAPPS 1.0: aggregate production planning using the multiple-objective tabu search, International Journal of Production Research,39(16), 3685-3702. [2] Bellman, R.E., Zadeh, L.A., 1970. Decision-making in a fuzzy environment. Management Science 17, 141164. [3] Boppana, V.C. and Slomp, J. (2002) Production Planning Under Dynamic Product Environment: A Multi-objective Goal Programming Approach, Research Report, No. 02A12, University of Groningen. Netherlands. [4] Bushuev, M. (2014), Convex optimization for aggregate production planning, International Journal of Pro-duction Research,52(4), 1050-1058. [5] Dai, L., Fan, L. and Sun, L. (2003) Aggregate production planning utilizing a fuzzy linear programming, Journal of Integrated Design and Process Science, Vol. 7, No. 4, pp.8195. [6] Filho S., Cezarino W. and Ratto J. (2010), Aggregate production planning: Modeling and solution via Excel spreadsheet and solver, IFAC Proceedings Volumes, vol. 43, no. 17. [7] Fung, R. Y., Tang, J., and Wang, Q. (2003), Multiproduct aggregate production planning with fuzzy demands and fuzzy capacities, IEEE Transactions on Systems, Man and Cybernetics, Part A: Systems and Humans,33(3), 302-313. [8] Fung, R. Y., Tang, J., and Wang, Q. (2003), Multiproduct aggregate production planning with fuzzy demands and fuzzy capacities, IEEE Transactions on Systems, Man and Cybernetics, Part A: Systems and Humans,33(3), 302-313. [9] Fung, R. Y., Tang, J., and Wang, Q. (2003), Multiproduct aggregate production planning with fuzzy demands and fuzzy capacities, IEEE Transactions on Systems, Man and Cybernetics, Part A: Systems and Humans,33(3), 302-313. [10] Ghasemy Yaghin, R., Torabi, S. A., and Fatemi Ghomi,S. M. T. (2012), Integrated markdown pricing and aggregate production planning in a two echelon sup-ply chain: a hybrid fuzzy multiple objective approach, Applied Mathematical Modelling,36(12),6011-6030. [11] Giannoccaro, I. and Pontrandolfo, P. (2001), Models for supply chain management: a taxonomy, Proceedings of the POM-2001 Conference: POM Mastery in the New Millennium, Orlando, FL, 2001. [12] Hannan, E. L. (1981), Linear programming with multiple fuzzy goals, Fuzzy Sets and Systems,6(3), 235-248. [13] Holt, C. C., Modigliani, F., and Simon, H. A. (1955), A linear decision rule for production and employment scheduling, Management Science,2(1), 1-30. [14] Holt, C.C., Modigliani, F., Simon, H.A., 1955. Linear decision rule for production and employment scheduling. Management Science 2, 130. [15] Hossain, M.M., Nahar, K., Reza, S., & Shaifullah, K.M. (2016). Multi-period, Multi-product, Aggregate Production Planning under demand uncertainty by considering Wastage Cost and Incentives. https://www.semanticscholar.org/paper/Multi-period-%2C-Multi-product-%2C-Aggregate-Production-Hossain-Nahar/3b72a55d180334313ef4681614267751cbdfece0#paper-header
[16] Hwang, C.L., Yoon, K., 1981. Multiple Attribute Decision Making: Methods and Applications. Springer, Berlin. [17] Jain, A. and Palekar, U. S. (2005), Aggregate production planning for a continuous reconfigurable manufacturing process, Computers and Operations Research,32(5), 1213-1236. [18] Jamalnia, A. and Feili, A. (2013), A simulation testing and analysis of aggregate production planning strategies, Production Planning and Control,24(6),423- 448. [19] Karmarkar, U. S. and Rajaram, K. (2012), Aggregate production planning for process industries under oligopolistic competition, European Journal of Operational Research,223(3), 680-689. [20] Khoshnevis B., Wolfe P. and Terrell M. (1982) Aggregate planning models incorporating productivity an overview, International Journal of Production Research, vol. 20, no. 5, pp. 555564. [21] Lai, Y.J., Hwang, C.L., 1992b. Fuzzy Mathematical Programming: Methods and Applications. Springer, Berlin. [22] Leung, S. C. and Chan, S. S. (2009), A goal programming model for aggregate production planning with resource utilization constraint, Computers and Industrial Engineering,56(3), 1053-1064. [23] Leung, S. C. and Wu, Y. (2004), A robust optimization model for stochastic aggregate production planning, Production Planning and Control,15(5), 502- 514. [24] Leung, S. C., Wu, Y., and Lai, K. K. (2003), Multi-site aggregate production planning with multiple objectives: a goal programming approach, Production Planning and Control, 14(5), 425-436. [25] Mirzapour Al-e-Hashem, S.M. J., Aryanezhad, M. B., and Sadjadi, S. J. (2012),An efficient algorithm to solve a multi-objective robust aggregate production planning in an uncertain environment, International Journal of Advanced Manufacturing Technol-ogy,58(5-8), 765-782. [26] Nam, S. J. and Logendran, R. (1992), Aggregate production planning: a survey of models and methodologies, European Journal of Operational Research,61(3), 255-272. [27] Piper C. J. & Vachon S. (2001) Accounting for productivity losses in aggregate planning, International Journal of Production Research, 39:17, 4001-4012,DOI: 10.1080/00207540110073019
[28] Ramezanian, R., Rahmani, D., and Barzinpour, F. (2012), An aggregate production planning model for two phase production systems: solving with genetic algorithm and tabu search, Expert Systems with Ap-plications,39(1), 1256-1263. [29] Tamiz M., (1996) Multi-objective programming and goal programming theories and Applications, Germany: Springer-Verlag. [30] Tonelli, F., Paolucci, M., Anghinolfi, D., and Taticchi, P.(2013), Production planning of mixed-model assembly lines: a heuristic mixed integer programming based approach, Production Planning andControl,24(1), 110-127. [31] Wang, R. C. and Liang, T. F. (2004), Application of fuzzy multi-objective linear programming to aggregate production planning, Computers and Industrial En-gineering,46(1), 17-41. [32] Wang, R.C. and Fang, H.H. (2001) Aggregate production planning with multiple objectives in a fuzzy environment, European Journal of Operational Research, Vol. 133, pp.521536. [33] Wang, R.C., Liang, T.F., 2004a. Application of fuzzy multi objective linear programming to aggregate production planning. Computers and Industrial Engineering 46 (1), 1741. [34] Wang, Reay-Chen & Liang, Tien-Fu. (2005). Applying possibilistic linear programming to aggregate production planning. International Journal of Production Economics. 98. 328-341. 10.1016/j.ijpe.2004.09.011. [35] Yaghoobi, M.A.,Tamiz, M., 2007. A method for solving fuzzy goal programming problems based on MINMAX approach. Eur. J. Oper. Res. 177, 15801590 [36] Zadeh L. A, (1965) Inform. Contr., 8 338-353. [37] Zimmermann, H.J., 1976. Description and optimization of fuzzy systems. International Journal of General Systems 2, 209215. [38] Zimmermann, H.-J., 1978. Fuzzy programming and linear programming with several objective functions. Fuzzy Sets and Systems 1, 4556.