
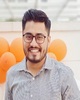
- Open Access
- Authors : Jaideep Saraswat , Akanksha Golchha , Nikhil Mall
- Paper ID : IJERTV11IS030032
- Volume & Issue : Volume 11, Issue 03 (March 2022)
- Published (First Online): 17-03-2022
- ISSN (Online) : 2278-0181
- Publisher Name : IJERT
- License:
This work is licensed under a Creative Commons Attribution 4.0 International License
Using Multi-Server Single Line Queuing Analysis to Design Electric Vehicle Charging Bays Optimally
Jaideep Saraswat, Akanksha Golchha, Nikhil Mall
Abstract.:- The world is facing a perilous challenge in climate change caused by anthropogenic emissions that are making the earth increasingly fragile. The transport sector is one of the leading sources of global emissions. Thus, the electrification of the transport sector is manifested as a vital intervention to decarbonize this sector. In India, though the penetration of electric vehicles is increasing
rapidly, they still account for a minuscule proportion in comparison to internal combustion engine vehicles. The range anxiety
characterized by the prevalence of fear that the vehicle will discharge before reaching the destination, associated with electric vehicles is the biggest deterrent. Addressing range anxiety is a catch-22 situation where the deployment of electric chargers is subdued due to the low presence of electric vehicles on the road and vice versa. This paper develops a multi-server single line queueing model that will assist in identifying the number of chargers, their capacity, and spare parking spaces needed to have a feasible business model at play. We have also carried out sensitivity analysis for several parameters in the model to gauge the impact on finances for the charge point operators.
Keywords: Electric Vehicles; Charging Point Operators; Queuing Analysis; Siting Framework
INTRODUCTION
Anthropogenic climate change has posed itself as the biggest existential challenge for humanity. The secretary-general of the United Nations (UN) has termed the report released by the Inter-governmental Panel on Climate Change (IPCC) in 2021 as the code red for humanity [1]. As per the report, limiting the global warming target below 1.5°C implies that a carbon budget of 400-800 Gt-CO2 is remaining and the world must fall to net-zero by 2050 [2]. This implies that from 2050 any additional release of Greenhouse Gas (GHG) emissions must be balanced by sinks. Moreover, for this decade, as per United Nations Environment Program (UNEP) Emissions Gap Report 2021, the world must halve its GHG emissions to meet the said target [3].
India has been showing exhilarating leadership to address climate change at the global level. In 2021, it became one of the few countries meeting its commitments under the Paris Accord almost 9 years in advance [4]. Moreover, the recently concluded Conference of Parties (COP) 26 saw, India announcing its commitment to be net-zero by 2070 [5]. For India to transition towards net-zero, it must decarbonize its major GHG emitting sectors.
The transportation sector is the third-largest GHG emitting sector in India, behind the power and industry sectors, accounting for 13.5% of Indias GHG emissions as seen in Figure 1. Of this, around 90% of total energy consumption is attributed to road transportation [6].
Others 5.6%
Residential 3.9%
Transport 13.5%
Industry 26.1%
Power Generation 50.9%
0.0% 10.0% 20.0% 30.0% 40.0% 50.0% 60.0%
% CO2 Emissions
Figure 1: Sector-wise CO2 Emissions in India [7]
Electrification of transport systems presents itself as the most promising solution to decarbonize this sector. Moreover, there is a strong push from the Government of India (GOI) to facilitate Electric Vehicles (EV) adoption. The GOI has released phase-II of the Faster Adoption and Manufacturing of Electric and Hybrid Vehicles (FAME-II) scheme with an outlay of INR 10,000 crore [8]. Also, it has set specific EV sales penetration targets for various vehicle segments as mentioned in Figure 2. Moreover, inspired
by the proactive steps of the central government, more than 25 State of Union Territory (UT) governments have released their EV policies [9].
90%
80%
70%
70%
80% 80%
60%
% EV SALES
% EV SALES
50%
40%
30%
30%
40%
20%
10%
0%
Private Cars Commercial
Cars
Buses Two-Wheelers Three-Wheelers
Figure 2: EV Sales Penetration Target- 2030 [10]
These interventions have certainly led to an increase in EV sales in India. The EV sales have risen at a CAGR of 39% from FY12 to FY22 [11]. In December 2021, a new feat was achieved when the EV registrations crossed the 50,000 mark for the first time in a month [12]. However, the quantum of EV sales in comparison to total vehicle sales is still minuscule. Figure 3 showcases that the EV sales accounted for a meager 2.16% of overall vehicle sales in India.
Figure 3: Year-on-Year EV sales in India [13]
The low uptake of EVs in India can be attributed to several reasons as seen in Figure 4. As per a global survey conducted by Deloitte in 2022, lack of public charging infrastructure tops as the biggest deterrent for consumers purchasing Electric Vehicles [14]. Specifically in India, this reason persists as 67.9% of respondents in the survey were not satisfied with the lower number of charging stations [15].
Figure 4: Reasons for Low Uptake of EVs in India [15] [16] [17] [18]
STATE OF ELECTRIC VEHICLE CHARGING INFRASTRUCTURE IN INDIA
As per the E-Amrit portal launched by the GOI, there are 1800 public chargers installed in India by February 2022. [19] Of these chargers, most of them are owned by state actors while some prominent industry actors like Tata Group are also foraying into the EV charging business. The world average for chargers per million people is around 190. As seen in Figure 5, the Netherlands has 20 times more chargers than the world average. On the flip side, India has a minuscule 1.5 chargers per million people. Several research studies have validated that the availability of public charging infrastructure soars EV adoption [20]. As per the study by Grant Thornton and FICCI, India requires around 400,000 EV chargers by 2026 to meet the requirements of 2 million EVs [21]. Also, as per NITI Aayog, around 13.5 Million Square Feet (MSF) of real estate will be needed for charging stations around the same time frame [22].
4000
3500
NO. OF CHARGERS
NO. OF CHARGERS
3000
3800
3200
2500
2000
1500
1000
500
0
1300
1100
400
190
1.5
Figure 5: No. of Public Chargers per Million People [23]
Clearly, a robust network of EV chargers is needed for the large-scale adoption of EVs in India. For this to happen, it is vital to attract private sector investments in the sector to ratchet up the charging infrastructure. To enable this, the GOI has already stripped off policy and regulatory roadblocks that will increase investor confidence. In January 2022, it released the consolidated guidelines & standards for charging infrastructure for EVs [24]. As per these guidelines, setting up of charging station is a de-licensed activity and can be carried out by any individual/organization while adhering to all the necessary technical and safety guidelines issued in this regard. Also, the distribution utilities have been provided with strict timelines to issue connections to public charging stations. This will expedite the commissioning of projects in the future. Along with this, it will entice utilities to gravitate towards transport
sector electrification. As per the Boston Consulting Group, EV can create an additional USD 3-10 Billion value for mid-sized utilities having 2-3 Million customers [25]. Moreover, the public charging stations are free to procure power from any generator in the country via open access afte paying the necessary grid charges, the provision of which must be made within 15 days. The government has also come up with a target for charger density. In cities, the charging stations must be available in a grid of 3 km X 3 km while for the highways the grid size is 25 km X 25 km for electric 2-Wheelers, electric 3-Wheelers, and electric 4- Wheelers. However, for long-range heavy-duty vehicles like trucks and buses, the grid size is kept at 100 km X 100 km. An additional requirement of heavy-duty vehicles will be addressed through separate provisions at Transport Nagar and depots in the cities. More conducive is the fact that these guidelines support the installation of chargers beyond the aforementioned limits. Finally, these guidelines articulate the vision of the government for the installation of chargers. The government has come up with 2 phases to install charging stations. Phase-1, lasting for 2-3 years, will focus on installing chargers in all megacities with a population greater than 4 million as per the 2011 census. This phase will also cater to important highways catering to these megacities. In phase -2, lasting for 3-5 years, big cities, state capitals, etc., will be targeted along with the connecting highways. With these standards and guidelines, transparency will prevail in the EV ecosystem. However, there is a significant difference in charging an EV in comparison to fuelling an Internal Combustion Engine (ICE) vehicle. With the ease and possibility of charging EVs at home, maximum charging will take place at residential premises. [26] Furthermore, as ICE vehicles can be fuelled completely in less than 5 minutes at oil retail outlets, charging EVs is a different paradigm where even the fastest chargers will charge your vehicle in 40 minutes (Assuming 30kWh battery size getting charged with 50 kW DC charger) [27]. Although the trend of EV charging is inclined towards residential charging, the installation of chargers by EV owners is constrained by the inherent design of our urban areas and the lack of sufficient distribution infrastructure in the rural areas. There is a need to examine this divergence and innovate.
What is needed at this point is a model and associated financial analysis that can entice investors, in setting up EV charging bays (a large number of EV chargers and appropriate parking spaces). This paper proposes a queuing model as a solution as it can help integrate multiple decision parameters for setting up charging bays and arrive at the final decision, after detailed financial analysis.
QUEUING MODEL FOR AN EV CHARGING BAY
The charging bay design is based on several critical elements- the number of chargers, wait time of the EVs, the power capacity of the chargers, the availability of land parcels, etc. Figure 6 is a representative model of a charging bay. Various behavioral studies have indicated that the quality of service is usually validated through the rapidity of service a.k.a minimal time spent in the waiting lines [28] [29]. In this scenario, waiting lines occur due to the inconsistent rate of EVs arrival and the inconsistent time taken to charge them. However, in the longer run, both these facets reach an equilibrium identified with the average over time. Reducing time spent in the waiting lines can be realized by increasing service capacity through the concomitant increase in the number of chargers or increase in the power capacity of chargers. However, both these methods entail higher costs, both upfront and recurring. There is a need to analyze the trade-off between the cost of improved service and the cost of making customers wait. Clearly, the investment decision will revolve around multi-dimensional techno-economic factors basis which the optimum number of chargers and their capacity, spare parking spaces, and other amenities must be designed. The charging bays need to create a semblance between the investment required on creating the entire infrastructure and the return over their lifetime.
Figure 6: A representative schematic of charging bay
The queuing theory as a solution was first used by A.K. Erlang in 1917 to address the telephone network congestion problem.
- Since then, it has been utilized in several applications like bank tellers, shopping markets, and more [31] [32]. Also, as each application is different, based on its inputs and environmental parameters, there is a need to conduct certain modifications to accustom the model based on the application. Here, the multi-server single line queuing model is considered for the analysis as this closely resembles the charging bay orientation. This type of model is most prevalent in airline ticket and check-in counter applications and this paper builds on that understanding. [33]The multi-server single line queuing model will entail certain key parameters mentioned below:
- Queue discipline (Q) is the order in which the EVs are served/charged. For our model, it will be a first-come, first- served basis.
- Calling population (C) is the source of the EVs in the region where the charging bay will be located. This can be deduced by forecasting the penetration of EVs in the region based on current and past EV sales. The data on current and past EVsales in the region can be obtained through primary or secondary research. Moreover, it is important to understand that the calling population will be infinite in the long run based on the ambitious climate targets set by India.
- Arrival rate (A), is the rate at which the EVs arrive at the charging bay during a specified period of time. This data can be obtained by studying the existing charging bays [34]. Also, several studies have demonstrated that the arrival rate at a service facility can frequently be defined by a Poisson Distribution [35] [36].
- Service rate (S), is the rate at which the average number of EVs can be charged during a specified period of time. The EV charging case is a peculiar case where service rate cannot be defined by a probability distribution. Studies on caseswith undefined services like EV charging times can be represented as a mean and standard deviation obtained via Monte- Carlo Simulation [37].
- Number of Charging Stations in the bay (c).
- Mean effective service rate (c X S).Moreover, the charging bays represent a finite queuing model where the length of the queue is limited by the parking space availability. Charging bays will be able to allow only a limited number of EVs to enter the waiting area termed as the queue. And if the queue is full the prospective EVs will drive on.
The operating characteristics of this model are defined by the following formulae: P0, the probability that there are no customers in the system,
=1
1 A n 1 A c cS
0 = 1/([ ! (S) ] + c! (S)
=0
( ))
cS A
L, Average number of EVs entering the charging bay,
()
=
+
( 1)! ( )2 0
W, Average time an EV spends in the charging bay (waiting and being charged),
=
Lq, Average number of EVs waiting to be charged in the specified time units,
=
Wq, Average time an EV spends in the waiting line to be charged,
1
= =
Pw, Probability that the EV entering the bay will have to wait before a charger is free,
1
= ! ()
0
Using these formulae, an excel model was developed that is capable of carrying multiple simulations. Table 1 represents the assumptions considered in the model development phase.
Table 1: Key Assumptions
Parameters Consideration Values Arrival Rate (A) Poisson Distribution Mean = Standard Deviation = 20 minutes No. of Parking Spots for EVs to wait Constant 1 /td>
Service Rate Random Type of Charger DC Fast 50 kW Total Operational Hours in a Day Constant 20 Hours - Mean = 30 minutes
- Standard Deviation = 15 minutes
Based on the above assumptions, the model was allowed to run for 100 simulations. Table 2 captures the sensitivity analysis on the number of chargers in the bay. It can be observed that the values for the 4 and 5 number of chargers in the bay were the same. This is because even with 4 chargers, the charging bay was able to meet the demand for EV charging in the region. And with similar demand, an additional charger will remain idle, if installed. Moreover, the fractional values for U and Lq are attributed to the mean taken across 100 simulations. Here, another important observation is the difference between the average number of EVs entering the bay and the average number of EVs served. This is for the reason that the charging bay is considered to be operational for 20 hours a day. Finally, the arrival and service rate can be easily catered to by 3 DC fast chargers. The service level with this configuration is nearing 100%.
Table 2: Sensitivity Analysis on No. of Chargers in the Bay
Sr. No.
Parameters Number of Chargers in the Bay 1 2 3 4 5 1 Average number of EVs entering the charging bay (L) 37 55 58 59 59 2 Average number of EVs unable to enter the bay (U) 21.35 3.43 0.45 0 0 3 Service Level of the bay (L/(U+L)) 64% 94% 99% 100% 100% 4 Average number of EVs waiting to be charged (Lq) 0.54 0.12 0.02 0 0 5 Average time an EV spends in the waiting line to be charged (Wq, minutes) 17.62 2.6 0.32 0.03 0 6 Average number of EVs served 35 53 57 57 57 However, to examine the plausibility of using 2 chargers to cater to the assumed arrival and service rate, sensitivity analysis was conducted on the number of parking spots available for EVs to wait. Here, we observed that even two DC fast chargers can optimally address the requirement of EVs when there are two spare parking spots available for EVs to enter the bay and wait.
Table 3: Sensitivity Analysis on No. of Parking Spots available for EVs to wait
Sr.No. Parameters No. of Parking Spots for EVs to wait 1 2 1 Average number of EVs entering the charging bay (L) 55 58 2 Average number of EVs unable to enter the bay (U) 3.43 0.93 3 Service Level of the bay (L/(U+L)) 94% 98% 4 Average number of EVs waiting to be charged (Lq) 0.12 0.22 5 Average time an EV spends in the waiting line to be charged (Wq, minutes) 2.6 4.48 6 Average number of EVs served 53 56 FINANCIAL ANALYSIS
To examine the financial feasibility of the charging bay, an excel model was developed to assess key financial parameters such as net present value (NPV), Project and Equity Internal Rate of Return (IRR), payback period, debt service coverage ratio, and more. The financial model was designed to assess the optimum scenario identified in the previous section for the charging bay i.e., 2 chargers; catering to 53 cars per day having 2 spare parking spots.
The financial feasibility assessment was based on the following key assumptions:
- The financial projection is computed for a period of 10 years, equivalent to the life of chargers [38].
- The debt-equity ratio is 70:30, the cost of debt is assumed 10% per annum and the cost of equity is assumed at 15.50% per annum [39]. The weighted Average Cost of Capital (WACC) is 9.83% per annum.
- The land is on a long-term fixed annual lease and other assets are depreciated using the written down value method at INR 10,00,000 per annum.
- Capital cost includes cost of one 50 kW DC City Charger (INR 13,00,000), Ancillary Equipment (INR 5,00,000), other capital expenditure (INR 4,00,000), CCTV Camera set up (INR 30,000), Active Harmonic filter (INR 76,500), and Fire Protection Equipment & Facilities (INR 30,000).
- There are no subsidies or tax incentives accounted for in the model [40].
- Taxes have been computed as applicable under the Income Tax Act, 1961 with no tax holidays [41].
- Revenue has been computed assuming 100% Capacity Utilization Factor (CUF) for 20 hours of charging operations 365 days a year.
- The electricity tariff for the charging bay owner is INR 5.8/kWh and is sold at a margin of INR 1.5/kWh to the EV [19]. The electricity tariffs increase by INR 1/kWh every three years. A service charge of INR 100 per visit is also levied onthe EVs.
- Other costs are incurred towards human resources, advertisement, information technology infrastructure, and network service provider, escalating at 5% per annum.
Table 4 provides a detailed financial assessment. Furthermore, the sensitivity analysis was carried out to see the impact on key financial parameters for the scenario when the number of EVs served per day decreases to 45.
Table 4: Financial Ratios and Sensitivity Analysis
Sr. No. | Particulars | Units | Average number of EVs served | |
56 | 45 | |||
1 | Weighted Average Cost of Capital | % | 9.83% | 9.83% |
2 | Land Lease | INR (in lacs) | 10 | 10 |
3 | Total Capital Expenditure | INR (in lacs) | 23.36 | 23.36 |
4 | Electricity sold to EVs | kWh | 4,70,120 | 3,77,775 |
5 | Equity IRR | % | 56.49% | – | |
6 | Project IRR | % | 24.81% | -1.05% | |
7 | Net Present Value | INR | 14,52,729 | (7,99,373) | |
8 | Payback Period of the Project | Years | 3.46 | – | |
9 | Minimum DSCR | Ratio | 2.17 | 0.67 | |
10 | Average DSCR | Ratio | 2.34 | 0.83 |
The financial feasibility is determined based on the following parameters:
- Internal Rate of Return (IRR) should be more than the Weighted Average Cost of Capital (WACC) also known as the hurdle rate [42].
- Net Present Value (NPV) should be positive [42].
- Payback period should not be very high as it renders investment unattractive [43].
- Minimum Debt Service Coverage Ratio (DSCR) should not be less than 1 and the average DSCR should be 1.2 or above [44].
Considering the financial feasibility criteria specified above, it is established that setting up an EV charging bay is a viable proposition with 56 EVs charged in a day. In this scenario, the project meets all the critical ratios used to measure the profitability of a business case such as IRR and NPV. The DSCR is in the specified range, establishing the credit worthiness of the business. In addition, the time required to recoup the initial investment is also less than four years, providing an option to reinvest the funds in business expansion.
On the flip side, the financial feasibility of the business is not attractive in case the daily number of EVs served is around 45. Through iteration, it is identified that the minimum no. of EVs that needs to be catered to is 49. In this case, the project IRR becomes 10.06%, more than the WACC of 9.83%.
CONCLUSION
As addressing the issue of the lower number of chargers is critical to mainstream EVs in India, this research paper propounds an approach to increase private sector investments in this regard. The paper establishes that the multi-server single line queuing system can assist in the optimal designing EV charging bay. Additionally, it showcases that setting up an EV charging bay is a feasible proposition with a payback period of fewer than 4 years. However, one must be mindful that the first movers will be able to reap the maximum benefit from the value created by transport electrification.
Future work will include a better approximation of the arrival rate of EVs based on real-life datasets and transfer learning tacks. This is because the mainstreaming of EVs in India will lead to an arrival rate of less than a minute. Also, there is a need to better assess the depreciation of EV chargers and incorporate the cost in tandem in the financial analysis. As seen above, the estimate does not include incentives from the government as that abstains EV charging bay owners to levy surcharges beyond certain limits. And with a miniscule surcharge, the viability of this business hangs in the lurch. Going forward, the government must allow a free hand to EV charging bays in setting up service charges as these will be kept in check via market forces. With the inclusion of government subsidies and incentives, the business model may become even more lucrative. All these aspects have not been explored fully and therefore serve as a rich research agenda across disciplines like energy, electronics, economics, and more.
REFERENCES
[1] | U. Nations, “Guterres: The IPCC Report is a code red for humanity,” 09 08 2021. [Online]. Available: https://unric.org/en/guterres-the-ipcc-report-is-a- code-red-for- humanity/#:~:text=UN%20Secretary%2DGeneral%20Ant%C3%B3nio%20Guterres,%E2%80%9Ccode%20red%20for%20humanity.%E2%80%9D& text=However%2C%20strong%20and%20sustained%20reductions,gases%20would%20lim. |
[2] | S. Fankhauser, S. M. Smith, M. Allen, K. Axelsson, T. Hale, C. Hepburn, J. M. Kendall, R. Khosla, J. Lezaun, E. Mitchell-Larson, M. Obersteiner, L. Rajamani, R. Rickaby, N. Seddon and T. Wetzer, “The meaning of net zero and how to get it right,” Nature Climate Change, vol. 12, pp. 15-21, 2022. |
[3] | U. D. P. UNEP, “Emissions Gap Report 2021,” United National Environment Program , 2021. |
[4] | M. o. N. a. R. Energy, “India achieves target of 40 % installed electricity capacity from non- fossil fuel sources,” Press Information Bureau, 02 12 2021. [Online]. Available: https://pib.gov.in/PressReleasePage.aspx?PRID=1777364. |
[5] | M. o. N. a. R. Energy, “National Statement by Prime Minister Shri Narendra Modi at COP26 Summit in Glasgow,” Press Information Bureau, 01 11 2021. [Online]. Available: https://pib.gov.in/PressReleasePage.aspx?PRID=1768712. |
[6] | IEA, “Data and statistics,” International Energy Agency, 2021. [Online]. Available: https://www.iea.org/data-and- statistics?country=INDIA&fuel=CO2%20emissions&indicator=CO2BySector. |
[7] | C. A. Tracker, “Decarbonising the Indian transport sector pathways and policies,” 2020. |
[8] | M. o. H. Industries, “About FAME-II,” Government of India, 2019. [Online]. Available: https://fame2.heavyindustries.gov.in/content/english/1_1_AboutUs.aspx. |
[9] | N. Aayog, “State Level Policies,” Government of India, 2021. [Online]. Available: https://e-amrit.niti.gov.in/state-level-policies. |
[10] | N. A. &. R. M. Institute, “India’s Electric Mobility Transformation,” New Delhi, 2019. |
[11] | V. Dashboard, “Vehicle Registrations,” Ministry of Road Transport and Highways, 2022. [Online]. Available: https://vahan.parivahan.gov.in/vahan4dashboard/. |
[12] | J. R. &. Analytics, “EV Monthly Updates,” 2021. [Online]. [Accessed 2022]. |
[13] | C. f. E. Finance, “Electric Mobility Dashboard,” CEEW, 2022. [Online]. Available: https://cef.ceew.in/intelligence/tool/electric-mobility. |
[14] | Deloitte, “Global Automotive Consumer Study,” Deloitte, 2022. [Online]. Available: https://www2.deloitte.com/content/dam/Deloitte/in/Documents/consumer-business/in-cb-2022-gacs-noexp.pdf. |
[15] | O. Tupe, S. Kishore and A. Johnvieira, “Consumer Perception of Electric Vehicles in India,” European Journal of Molecular & Clinical Medicine, vol. 7, no. 8, pp. 4861-4869, 2020. |
[16] | P. Singh, “What would make Indians buy EVs?Our Survey Has Some Answers,” The Energy And Resources Institute, 11 09 2020. [Online]. Available: https://www.teriin.org/blog/what-would-make-indians-buy-evs-our-survey-has-some-answers. |
[17] | E. Pigge, “Many People Still Doubtful About Electric Cars Environmental Friendliness,” Continental, January 2020. [Online]. Available: https://www.continental.com/en/press/press-releases/mobility-study-electric-mobility/. |
[18] | P. Release, “Battleground for Electric Vehicle Purchase Consideration is Wide Open, J.D. Power Finds,” J.D. Power, 2021. [Online]. Available: https://www.jdpower.com/business/press-releases/2021-us-electric-vehicle-consideration-evc-study. |
[19] | N. Aayog, “E-Amrit,” 2022. [Online]. Available: https://e-amrit.niti.gov.in/home. |
[20] | D. Hall and N. Lutsey, “Electric vehicle charging guide for cities,” International Council on Clean Transportation, Washington DC, 2020. |
[21] | G. Thornton and FICCI, “India-Germany:Building an integrated and robust e-mobility ecosystem,” April 2021. [Online]. Available: https://www.grantthornton.in/insights/articles/india-germany-building-an-integrated-and-robust-e-mobility-system/. |
[22] | V. Bala, P. Bhargava and S. Goel, “Electric Mobility in Full Gear,&qot; Colliers, 2021. |
[23] | D. Hall, Y. Xie, R. Minjares, N. Lutsey and D. Kodjak, “Decarbonizing road transport by 2050: Effective policies to accelerate the transition to zero- emission vehicles,” ZEV Transition Council, pp. 1-41, December 2021. |
[24] | M. o. Power, Charging Infrastructure for Electric Vehicles (EV)-the revised consolidated Guidelines and Standards-reg, New Delhi: Government of India, 2022. |
[25] | T. Baker, S. Aibino, E. Belsito and A. Sahoo, “Electric Vehicles Are a Multibillion-Dollar Opportunity for Utilities,” Boston Consulting Group, 2019. |
[26] | S. Das, C. Sasidharan and A. Ray, “Charging India’s Four-Wheeler Transport,” Alliance for an Energy Efficient Economy, New Delhi, 2020. |
[27] | M. Stone, “Will charging electric cars ever be as fast as pumping gas?,” National Geographic, 2020. [Online]. Available: https://www.nationalgeographic.com/environment/article/will-charging-electric-cars-ever-be-as-fast-as-pumping-gas. |
[28] | V. A. Zeithaml, “Service quality, profitability, and the economic worth of customers: What we know and what we need to learn,” Journal of the Academy of Marketing Science, no. 28, pp. 191-201, 2000. |
[29] | B. Schneider and D. E. Bowen, “Employee and customer perceptions of service in banks: Replication and extension,” Journal of Applied Psychology,
vol. 70, no. 3, pp. 423-433, 1985. |
[30] | A. Erlang, “Solution of Some Problems in the Theory of Probabilities of Significance in Automatic Telephone Exchanges,” Post Office Electrical Engineers Journal, vol. 10, pp. 189-197, 1917. |
[31] | P. S., S. A. G and S. M., “Crowd Tendency in Retail Shops and Machine Learning Algorithm for Reducing Queuing Time in Billing Counters,” Ilkogretim Online, vol. 20, no. 1, pp. 2349-2354, 2021. |
[32] | W. Ngamsirijit, “Using queuing theory for evaluating flexibility performance in banking services,” International Journal of Services and Operations Management , vol. 12, no. 4, pp. 387-404, 2012. |
[33] | B. W. Taylor, Introduction to Management Science, Pearson, 2019. |
[34] | D. Rolnick, P. L.Donti and et.al., “Tackling Climate Change with Machine Learning,” ACM Computing Surveys, vol. 55, no. 2, pp. 1-96, 2022. |
[35] | R. Bekker, S. Borst, O. Boxma and O. Kella, “Queues with Workload-Dependent Arrival and Service Rates,” Queueing Systems, vol. 46, pp. 537-556, 2004. |
[36] | E. Modiano, Introduction to Queueing Theory, Massachusetts Institute of Technology, 2020. |
[37] | M. Vorobjovs, K. Berzma and A. Zirovecka, “Electric Vehicle Charging Station Load Analyzing Based on Monte-Carlo Method,” in European Conference on Power Electronics and Applications, 2018. |
[38] | G. Insurance, “Electric Vehicle Charging Stations,” [Online]. Available: https://www.gny.com/products/electric-vehicle-charging-stations. [Accessed 2022]. |
[39] | Forum of Regulators, “Study on Impact of Electric Vehicles on the Grid,” Forum of Regulators, Delhi, 2017. |
[40] | S.Majumdar, Charging Infrastructure for Electric Vehicles (EVs)- the revised consolidated guidelines and standards, New Delhi: Ministry of Power, 2022. |
[41] | The Income Tax Act, 1961, Delhi, 1962. |
[42] | D. Chang, E. Daniel, E. Lin, A. F. Rice, C. T. Goh, A.-A. Tsao and D. J. Synder, “Financial Viability of Non-Residential Electric Vehicle Charging Stations,” UCLA Anderson School of Management, 2012. |
[43] | J. Kagan, “Investopedia,” 07 February 2022. [Online]. Available: www.investopedia.com. [Accessed 03 March 2022]. |
[44] | Reserve Bank of India, Resolution Framework for COVID-19-related Stress Financial Parameters, Reserve Bank of India, 2020. |