
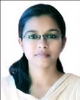
- Open Access
- Authors : Athira K R , Varsha Murali , Aswin C R , Devaprabha B, Aneesh P C
- Paper ID : IJERTV10IS060185
- Volume & Issue : Volume 10, Issue 06 (June 2021)
- Published (First Online): 18-06-2021
- ISSN (Online) : 2278-0181
- Publisher Name : IJERT
- License:
This work is licensed under a Creative Commons Attribution 4.0 International License
Water Quality Modeling and Regression Analysis of Vembanad Lake and its Primary Inflows
Athira K R1, Aswin C R1, Devaprabha B1, Varsha Murali1
1UG Students, Dept. of Civil Engineering,
Adi Shankara Institute of Engineering and Technology, Kalady, India
Aneesh P C2
2Head of the Department, Dept. of Civil Engineering, Adi Shankara Institute of Engineering and Technology, Kalady India
Abstract: Water quality modeling involves the prediction of water pollution using mathematical simulation techniques. A typical water quality model consists of a collection of formulations representing physical mechanisms that determine position and momentum of pollutants in a water body. Modeling can be used to assess and predict future water quality situations resulting from different management strategies. Surface water quality models can be useful tools to simulate and predict the levels, distributions, and risks of chemical pollutants in a given water body.Water quality modeling using Artificial Neural Network (ANN) is a computational method animated by the studies of the brain and nervous system. Our project aims to predict the accuracy of water quality models of Vembanad Lake and its primary inflows by performing regression analysis. And it also aims to develop a relationship between water quality parameter and water quality index of Vembanad Lake and its primary inflows.
Keywords: ANN, Regression, Water quality
-
INTRODUCTION
Water quality study is a diverse area of activity that generally involves developing mathematical and logic- based representations of real world relationships between different variables that is the spatial and temporal relationships between water quality pollutants, stream hydrology, plant life and other chemical components. Surface water quality modeling is useful to simulate and predict the levels, distributions, and risks of chemical pollutants in a given water body. Surface water quality modeling results from surface water quality models under different pollution scenarios, which are very important components of environmental impact assessment and can provide a basis and technique for environmental management agencies to make right decisions.
Modeling can be used to assess and predict future water quality situations resulting from different management strategies. Artificial Neural Networks (ANNs) have seen an explosion of interest over the last two decades and have been
successfully applied in all fields of chemistry and particularly in analytical chemistry. ANNs are capable of gradual learning over time and modeling extremely
complex functions. ANNs are often applied for prediction, clustering, classification, modelling of a property, process control, procedural optimization and/or regression of the obtained data.
Regression analysis is one of the most frequently used tools in market research. In its simplest form, regression analysis allows market researchers to analyze relationships between one independent and one dependent variable..The key benefits of using regression analysis are that it can indicate if independent variables have a significant relationship with a dependent variable, Indicate the relative strength of different independent variables effects on dependent variable, Make predictions.
-
OBJECTIVE
-
To predict the accuracy of water quality models of Vembanad Lake and its primary inflows
-
To develop a relationship between water quality index of Vembanad lake and primary inflows
-
To develop a relationship between water quality parameters and water quality index of Vembanad Lake and its primary inflows.
-
-
METHODOLOGY
3.1. Study Area Identification
Vembanad Lake is the longest lake in India as well as the largest lake in the state of Kerala. The Vembanad Lake spanning between latitudes 9° 28' and 10°10' N and
longitudes 76° 13' and 76° 31' E is the biggest among the 30 backwaters in the State. This has an area of about 22750 km2 spread in to three districts, viz., Ernakulam in the north, Kottayam in the east and Alappuzha (Alleppey) in the south. The western side is bordered by a narrow belt of land separating the lake from the LakshadweepSea. It extends to a length of 96.5 km from Azhikode in the north to Alappuzha town in the south. The lake north of Aroor is situated in the erstwhile Cochin State, and is generally referred to as the Cochin backwaters.. The lake is fed by 10 rivers flowing into it including the six major rivers of central Kerala
namelythe Achenkovil, Manimala, Meenachil.river, Muvatt upuzha river, Pamba and Periyar. Table 1 anf fig 2 shows
the detailed study locations. Vembanad Lake and its incoming rivers is shown on fig 1.
Fig 1 Map of Vembanad Lake indicating incoming rivers Table 1 Sample Locations in detail
Forest and Climate Change, Govt. of India. The data includes temperature, pH, total coliform, fecal coliform, electrical conductivity, nitrate and dissolved oxygen.
Water quality index of Vembanad Lake and its primary inflows are calculated by weighted arithmetic method based on the data collected from CPCB from the year 2007 to 2019. Weighted arithmetic water quality index method classified the water quality according to the degree of purity by using the most commonly measured water quality variables. The method has been widely used by the various scientists and the calculation of WQI was made by using the following equation:[8]
The quality rating scale (Qi) for each parameter is calculated by using this expression:
Where,
Vi is estimated concentration of ith parameter in the analysed water , Vo is the ideal value of this parameter in pure water,
Vo = 0 (except pH =7.0 and DO = 14.6 mg/l),Si is recommended standard value of ith parameter
The unit weight (Wi) for each water quality parameter is calculated by using the following formula:
No |
Location |
District |
Latitude and Longitude |
R1 |
Vembanad Lake |
Kottayam |
9.5968° N, 76.3985° E |
S1 |
Manimala River |
Pathanamthitta |
9.4949° N, 76.7466° E |
S2 |
Pamba River |
Alappuzha |
9.3707° N, 76.4115° E |
S3 |
Achenovil River |
Alappuzha |
9.2814° N, 76.5199° E |
S4 |
Meenachil River |
Kottayam |
9.6821° N, 76.6080° E |
S5 |
Periyar River |
Kottayam |
10.0669° N, 76.2990° E |
S6 |
Muvattupuzha River |
Ernakulam- Kottayam |
9.8038° N, 76.4556° E |
No |
Location |
District |
Latitude and Longitude |
R1 |
Vembanad Lake |
Kottayam |
9.5968° N, 76.3985° E |
S1 |
Manimala River |
Pathanamthitta |
9.4949° N, 76.7466° E |
S2 |
Pamba River |
Alappuzha |
9.3707° N, 76.4115° E |
S3 |
Achenovil River |
Alappuzha |
9.2814° N, 76.5199° E |
S4 |
Meenachil River |
Kottayam |
9.6821° N, 76.6080° E |
S5 |
Periyar River |
Kottayam |
10.0669° N, 76.2990° E |
S6 |
Muvattupuzha River |
Ernakulam- Kottayam |
9.8038° N, 76.4556° E |
Where,
Wi =K /Si
K = proportionality constant and can also be calculated by using the following equation;
Table 2 Water quality rating as per weighted arithmetic method [6]
Where, wi = Average weight of parameter, Wi= Relative weight of parameter
Figure 2 Detail sample locations
-
Water Quality Index Calculation
Water quality data of Vembanad Lake and its primary inflows are collected from the year 2007 to2019 from water quality database, which is hosted by central pollution control board, sponsored by Ministry of Environment,
-
Regression Analysis
The relationship between water quality parameters of the selected areas and also water quality index of each location is found out by using regression analysis. Similarly the relationship between water quality index of Vembanad Lake and water quality parameters of primary inflows are also determined. For finding out the relationship between them, the seven parameters (temperature, pH, fecal
coliform, total coliform, electrical conductivity, dissolved oxygen, nitrate) are given as independent variable and water quality index is given as a dependent variable. In the same way, in case of Vembanad Lake and its primary inflows, water quality of index of Vembanad Lake is given as dependent variable and that of primary inflows is given as independent variable.[9]
Stepwise Multiple Regression
-
Main Regression analysis window
Select the path.Analyze – Regression – Linear. This brings us to the Linear Regression main dialog window. From the variables list panel, we click over esteem to the Dependent panel and negafect, posafect, neoopen, neoextra, neoneuro, and tanx to the Independent(s) panel. The Method drop-down menu contains the set of step methods that IBM SPSS can run. The only one you may not recognize is Remove, which allows a set of variables to be removed from the model together. Choose Stepwise as the Method from the drop-down menu shown in fig 3
Figure 3 Linear Regression toolbox
-
Statistical Window
Selecting the Statistics pushbutton brings us to the Linear Regression: Statistics window. Clicking Continue shown in fig 4 returns us to the main dialog box
Figure 4 Linear Regression Statistics toolbox
-
Options Window
Selecting the Options pushbutton brings us to the Linear Regression: Options dialog window displayed. The top panel is now applicable as we are using the stepwise method. To avoid looping variables continually in and out
of the model, it is appropriate to set different significance levels for entry and exit. Earning entry to the model is set at an alpha level of .05 (e.g., a variable with a probability of .07 will not be entered) and is the more stringent of the two settings. But to be removed, a variable must have an associated probability of greater than 0.10 (e.g., a variable with an associated probability of 0.12 will be removed but one with an associated probability of .07 will remain in the model). In essence, it is more difficult to get in than be removed. This is a good thing and allows the stepwise procedure to function. Click Continue shown in fig 5 to return to the main dialog window, and click OK to perform the analysis.
Figure 5 Linear regression option toolbar
-
Stepwise Multiple Regression Output
The four ANOVAs that are reported correspond to four models, but dont let the terminology confuse us. The stepwise procedure adds only one variable at a time to the model as the model is slowly built. At the third step and beyond, it is also possible to remove a variable from the model (although that did not happen in our example). In the terminology used by IBM SPSS, each step results in a model as shown in fig 6 and 7 and each successive step modifies the older model and replaces it with a newer one. Each model is tested for statistical significance
Figure 6 Model Summary from spss software
Of primary interest are the R Square and Adjusted R Square values, which are 1.000 and 0.999, respectively. We learn from these that the weighted combination of the predictor variables explained approximately 100% of the variance of self-esteem. The loss of so little strength in computing the Adjusted R Square value is primarily due to
our relatively large sample size combined with a relatively small set of predictors.
Figure 7 Regression analysis graph from spss software
The Part column under Correlations lists the semi partial correlations for each predictor once the model is finalized; squaring these values informs us of the percentage of variance each predictor uniquely explains the Y intercept of the raw score model is labeled as the Constant.
-
-
Water Quality modeling using ANN
Methodology is presented in fig 8 indicates the application of ANN to predict the WQI.
Neural Networks Models were developed for the prediction of yearly values of the water quality index of Vembanad Lake and its primary inflows.The yearly data of eight water quality parameters (pH, temperature, electrical conductivity, fecal coliform, total coliform, dissolved oxygen, nitrate), for the time period 2007-2019 were selected for this analysis. For neural network models construction, yearly data is randomly divided into three subsets:[7]
-
Training set (70%)
-
Validation set (15%)
-
Testing sets (15 %)
Figure 8 Methodology to apply ANN for WQI
IV RESULTS AND DISCUSSION
-
Water quality index calculation
Table 3 Water quality index obtined by weighted arithmetic method
Using weighted arithmetic method, water quality index was calculated for vembanad lake and its primary inflows with the data from 2007 to 2019
-
Regression analysis output
Figure 9 Model summary of vembanad lake from spss software
g o
g o
raphs of the primary inflows of Vembanad lake can be btained.
Figure 10 Coefficients obtained from spss software for vembanad lake
Fig 9 and 10 shows the output from spss software for regression analysis. Similarly, the outputs of the primary inflows of Vembanad lake can be obtained.
-
Relationship between Water Quality Index
Y=0.586Y1-0.147Y2-6.283Y3-5.040Y4+2.452Y5-7.235Y6+331.628
Where,
Y-WQI of Vembanad Lake
Y1-WQI of MeenachilY2-WQI of Periyar Y3- WQI of Muvattupuzha
Y4-WQI of Manimala Y5-WQI of Pambha
Y6-WQI of Achankovil
-
Water quality modeling using Artificial Neural Network
Figure 11 Regression graph from matlab
Figure 11 shows the output from matlab for predicting water quality model accuracy. Similarly, the Regression
V CONCLUSION
Regression analysis is a set of statistical processes for estimating the relationships between a dependent variable and one or more independent variables. This project deals with two cases of establishing relationship between water quality parameters of Vembanad Lake and its primary inflows. The project also concluded how the quality of water in the primary inflows of Vembanad Lake affects the quality of the water in the lake.This also established a relationship between water quality index of Vembanad Lake and its primary inflows thereby understanding the contribution of pollution of primary inflows into the Vembanad Lake.
an th
an th
In this project, Neural Networks Models were developed for the prediction of yearly values of the water quality index of Vembanad Lake and its primary inflows. The yearly data of eight water quality parmeters (pH, temperature, electrical conductivity, fecal coliform, total coliform, dissolved oxygen, nitrate), for the time period 2007-2019 were selected for this analysis. For neural network models construction, yearly data is randomly divided into three subsets: training set (70%), validation d testing sets (each is 15 %), were used. The results, for
e training and the test data sets distinctly prove the ability of the Neural Network models to predict yearly values of water quality index, at the monitoring station by using the yearly values of the other existing water quality parameters which are inserted as inputs into the optimized Neural Network models. Since the ability of ANN (Artificial Neural Network) model to predict water quality index depends on regression value,this project conclude that ,the best models to predict water quality index will be of Vemband Lake(R=1), Manimala(R=1), Achenkovil(R=0.92), Meenachil(R=1),and Periyar(R=1) and the the other two i.eMuvattupuzha (R=0.3) and Pambha(R= 0.4) are poor models to predict WQI. Hence, the Neural Network models can be used for the prediction of water quality parameters.
REFERENCES
-
Singkran, N., Yenpiem, A. and Sasitorn, P. (2010).Determining Water Conditions in the North eastern Rivers of Thailand Using Time Series and Water Quality Index Models. Journal of Sustainable Energy & Environment.1, 47- 48.
-
Yan, H., Zou, Z. and wang, H. (2010). Adaptive neuro fuzzy inference system for classification of water quality status.
Journal of Environmental Sciences. 22(12), 1891-1896
-
Khalil, B.M., Awadallah, A.G., Karaman, H. and El-Sayed, A. (2012).Application of Artificial Neural Networks for the Prediction of Water Quality Variables in the Nile Delta. Journal of Water Resource and Protection. 4, 388-394.
-
Areerachakul, S. (2012). Comparison of ANFIS and ANN for Estimation of Biochemical Oxygen Demand Parameter in Surface Water. International Journal of Chemical and Biological Engineering. 6, 286-290
-
Mahapatra, S.S., Sahu, M., Patel, R.K. and Panda, B.N. (2012). Prediction of Water Quality Using Principal Component Analysis. Water Qual Expo Health.4, 93104.
-
Mangukiya, R., Bhattacharya, T. and Chakraborty, S. (2012). Quality Characterization of Groundwater using Water Quality Index in Surat City, Gujarat, India. International Research Journal of Environment Sciences. 1(4), 14-23.
-
Jiang, Y., Nan, Z. and Yang, S. (2013). Risk assessment of water quality using Monte Carlo simulation and artificial neural network method. Journal of Environmental Management. 122, 130-136.
-
D. Satish Chandra. (2017). Estimation Of Water Quality Index By Weighted Arithmetic Water Quality Index Method. International Journal of Civil Engineering and Technology (IJCIET) Volume 8, Issue 4.
-
Sarad Chandra Kafle.(2019). Correlation and Regression Analysis Using SPSS.OCEM Journal of Management,Technology&SocialSciences